Self-Paced Clustering Ensemble
IEEE Transactions on Neural Networks and Learning Systems(2021)
摘要
The clustering ensemble has emerged as an important extension of the classical clustering problem. It provides an elegant framework to integrate multiple weak base clusterings to generate a strong consensus result. Most existing clustering ensemble methods usually exploit all data to learn a consensus clustering result, which does not sufficiently consider the adverse effects caused by some difficult instances. To handle this problem, we propose a novel self-paced clustering ensemble (SPCE) method, which gradually involves instances from easy to difficult ones into the ensemble learning. In our method, we integrate the evaluation of the difficulty of instances and ensemble learning into a unified framework, which can automatically estimate the difficulty of instances and ensemble the base clusterings. To optimize the corresponding objective function, we propose a joint learning algorithm to obtain the final consensus clustering result. Experimental results on benchmark data sets demonstrate the effectiveness of our method.
更多查看译文
关键词
Learning systems,Linear programming,Computer science,Task analysis,Clustering methods,Diversity reception,Training data
AI 理解论文
溯源树
样例
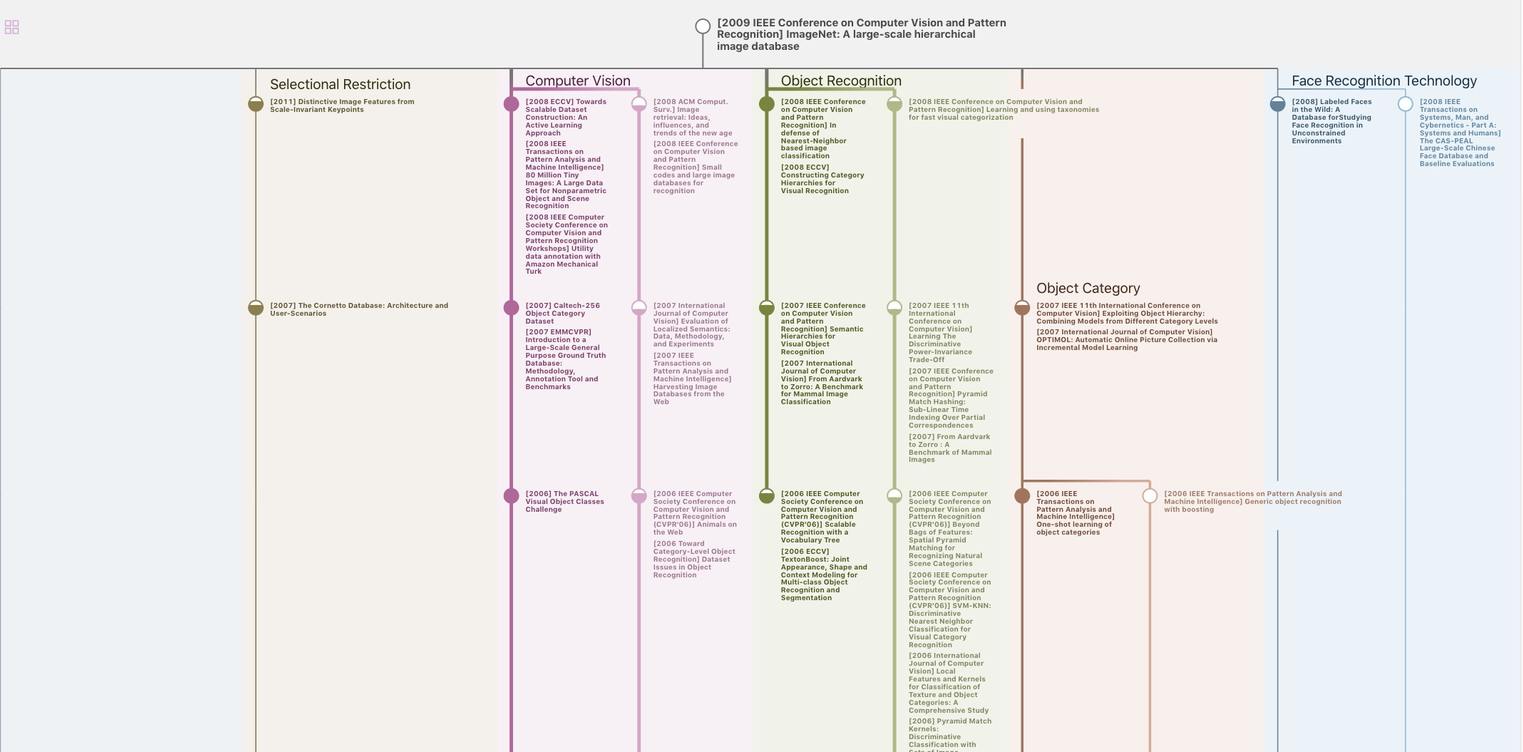
生成溯源树,研究论文发展脉络
Chat Paper
正在生成论文摘要