Deep Graph-Based Multimodal Feature Embedding for Endomicroscopy Image Retrieval.
IEEE Transactions on Neural Networks and Learning Systems(2021)
摘要
Representation learning is a critical task for medical image analysis in computer-aided diagnosis. However, it is challenging to learn discriminative features due to the limited size of the data set and the lack of labels. In this article, we propose a deep graph-based multimodal feature embedding (DGMFE) framework for medical image retrieval with application to breast tissue classification by learning discriminative features of probe-based confocal laser endomicroscopy (pCLE). We first build a multimodality graph model based on the visual similarity between pCLE data and reference histology images. The latent similar pCLE-histology pairs are extracted by walking with the cyclic path on the graph while the dissimilar pairs are extracted based on the geodesic distance. Given the similar and dissimilar pairs, the latent feature space is discovered by reconstructing the similarity between pCLE and histology images via deep Siamese neural networks. The proposed method is evaluated on a clinical database with 700 pCLE mosaics. The accuracy of image retrieval demonstrates that DGMFE can outperform previous works on feature learning. Especially, the top-1 accuracy in an eight-class retrieval task is 0.739, thus demonstrating a 10% improvement compared to the state-of-the-art method.
更多查看译文
关键词
Algorithms,Breast,Breast Neoplasms,Databases, Factual,Diagnosis, Computer-Assisted,Endoscopy,Female,Humans,Image Processing, Computer-Assisted,Machine Learning,Microscopy,Microscopy, Confocal,Neural Networks, Computer
AI 理解论文
溯源树
样例
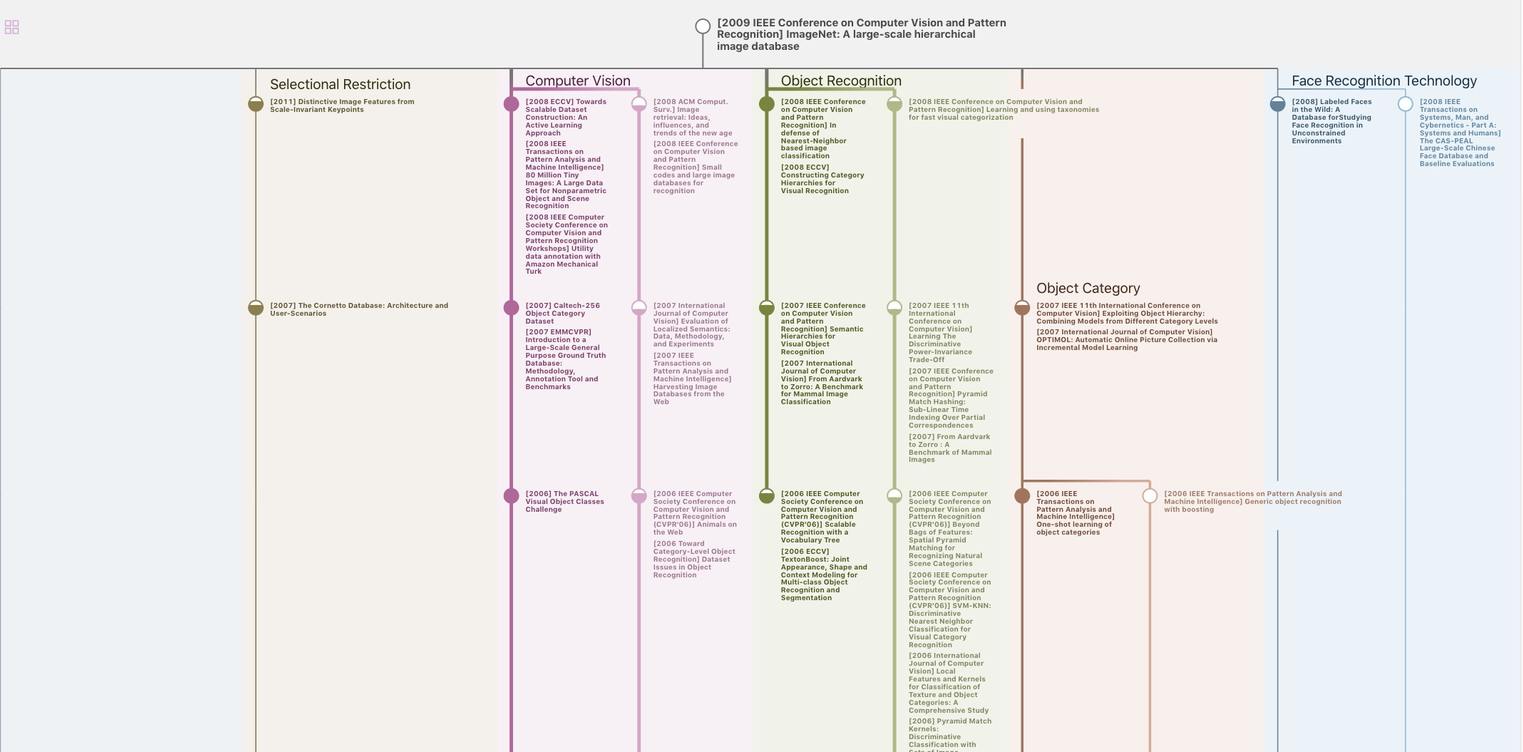
生成溯源树,研究论文发展脉络
Chat Paper
正在生成论文摘要