Improving Recommendation Diversity by Highlighting the ExTrA Fabricated Experts
IEEE ACCESS(2020)
摘要
Nowadays, recommender systems (RSes) are becoming increasingly important to individual users and business marketing, especially in the online e-commerce scenarios. However, while the majority of recommendation algorithms proposed in the literature have focused their efforts on improving prediction accuracy, other important aspects of recommendation quality, such as diversity of recommendations, have been more or less overlooked. In the latest decade, recommendation diversity has drawn more research attention, especially in the models based on user-item bipartite networks. In this paper, we introduce a family of approaches to extract fabricated experts from users in RSes, named as the Expert Tracking Approaches (ExTrA for short), and explore the capability of these fabricated experts in improving the recommendation diversity, by highlighting them in a well-known bipartite network-based method, called the Mass Diffusion (MD for short) model. These ExTrA-based models are compared with two state-of-the-art MD-improved models HHP and BHC, with respect to recommendation accuracy and diversity. Comprehensive empirical results on three real-world datasets MovieLens, Netflix and RYM show that, our proposed ExTrA-based models can achieve significant diversity gain while maintain comparable level of recommendation accuracy.
更多查看译文
关键词
Bipartite networks,diversity,fabricated experts,recommender systems
AI 理解论文
溯源树
样例
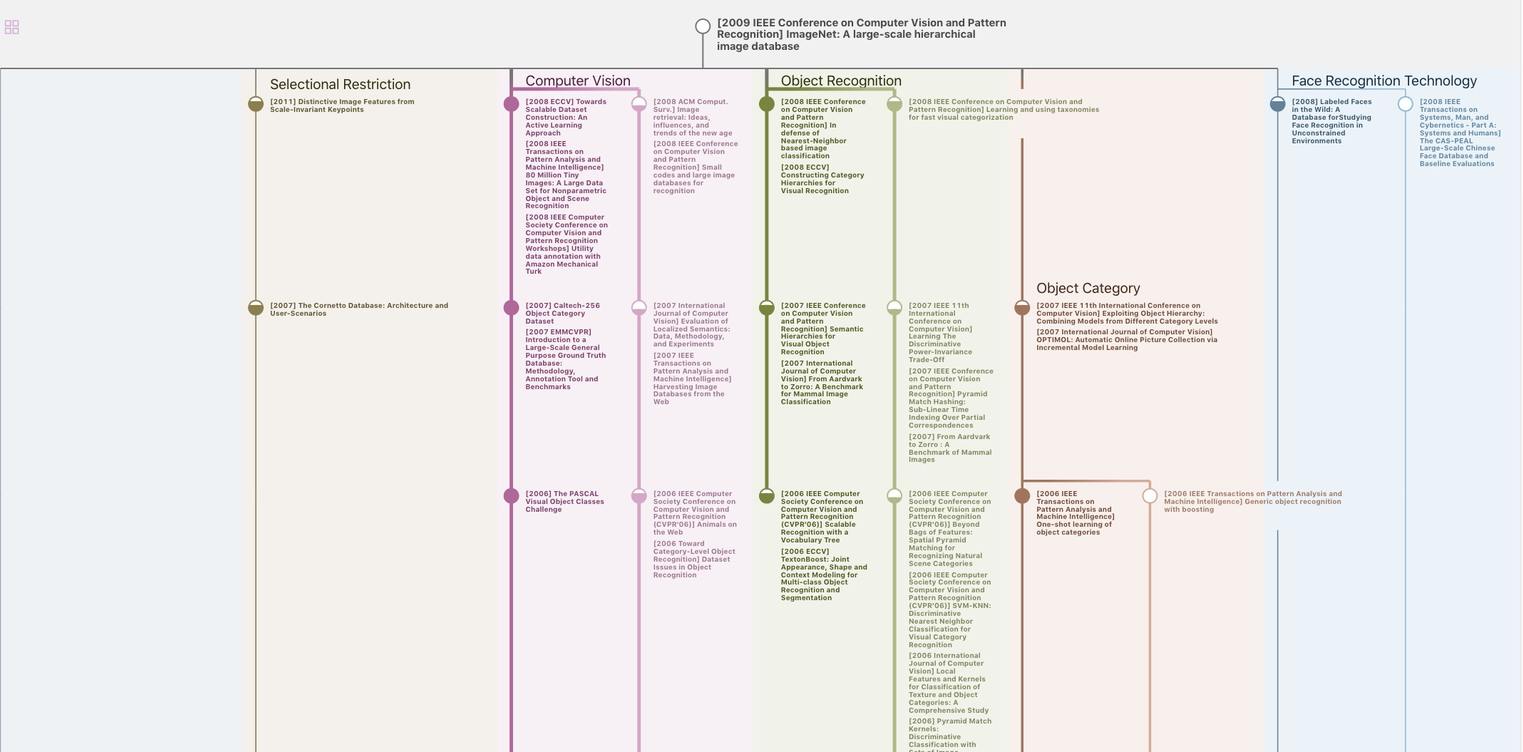
生成溯源树,研究论文发展脉络
Chat Paper
正在生成论文摘要