Recursive Learning-Based Bilinear Subspace Identification for Online Modeling and Predictive Control of a Complicated Industrial Process
IEEE Access(2020)
摘要
In this paper, a recursive learning based bilinear subspace identification (R-B-SI) algorithm is proposed for online modeling and data-driven predictive control of blast furnace (BF) ironmaking process with strong nonlinear time-varying dynamics. Different from the existing linear SI algorithms, the R-B-SI algorithm can make full use of the process data information by adding the Kronecker product term of input data and the Kronecker product term between input and output data into the data block Hankel matrices. Moreover, the parameters of the R-B-SI model can be updated online with the latest data using recursive learning. In order to adjust the sensitivity of the algorithm to the data at different sampling times, the forgetting factor is introduced into the involved recursive learning as well. Therefore, the nonlinear time-varying dynamics of process can be well captured and described by the R-B-SI model. As a result, the data-driven predictive controller designed by the R-B-SI prediction model with parameter adaption is always capable of achieving satisfactory control performances regardless of the variation in various process operating conditions. Data experiments using actual industrial data have verified the practicability and superiority of the proposed methods.
更多查看译文
关键词
Recursive bilinear subspace identification, online modeling, recursive learning, data-driven predictive control, blast furnace ironmaking process, nonlinear time-varying dynamics
AI 理解论文
溯源树
样例
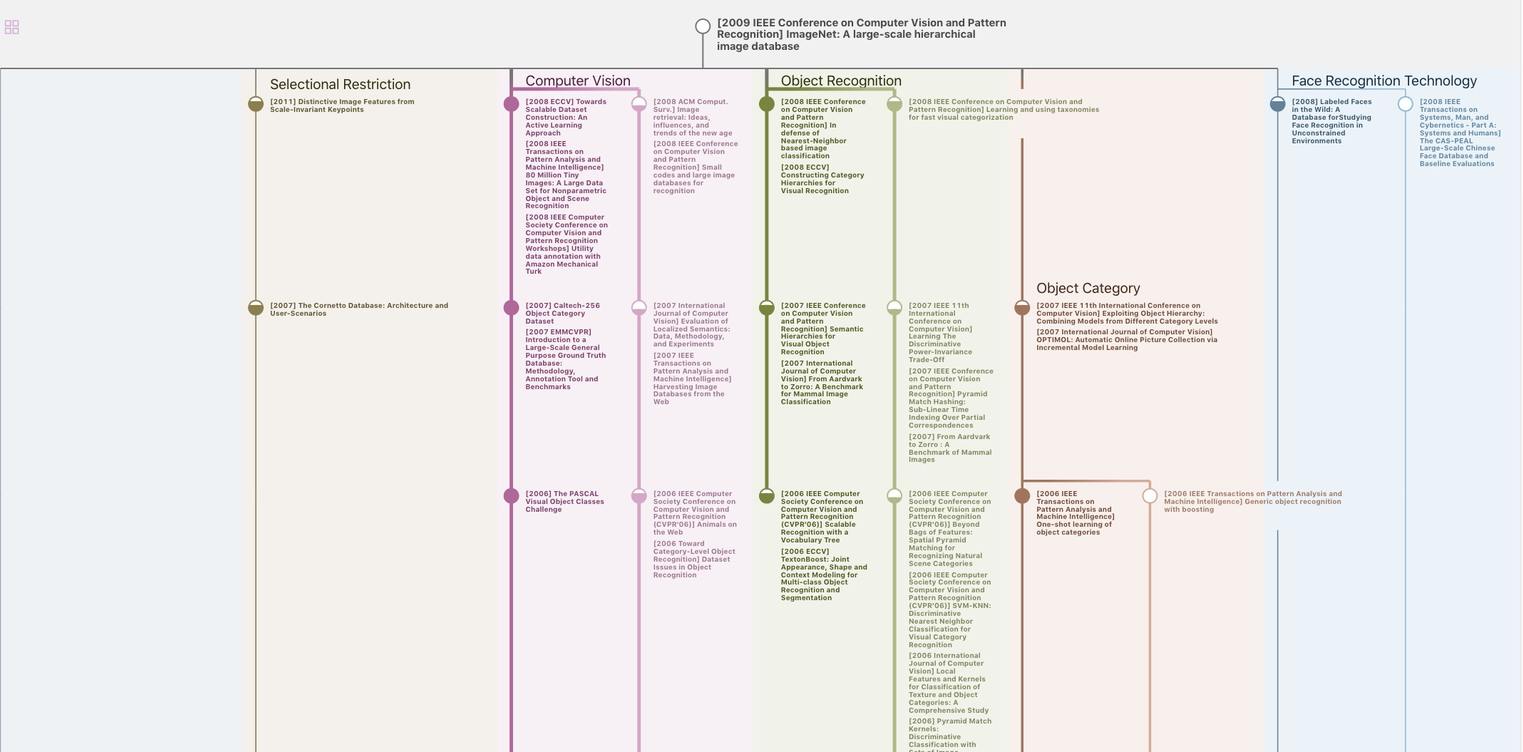
生成溯源树,研究论文发展脉络
Chat Paper
正在生成论文摘要