Low-Rank Regularized Deep Collaborative Matrix Factorization For Micro-Video Multi-Label Classification
IEEE SIGNAL PROCESSING LETTERS(2020)
摘要
Deep matrix factorization can be regarded as an extension of traditional matrix factorization to help improve applications like social image tag refinement, image retrieval, and face clustering. Toward this tendency, in this letter, we proposed a low-rank regularized deep collaborative matrix factorization (LRDCMF) method to better tackle micro-video multi-label classification tasks. The proposed method aims to collaboratively learn two sets of factor matrices for characterization of latent attributes and two deep representations for instances and labels, respectively. During factorization process, the inverse covariance constraints are exploited to capture the latent correlation structures among latent attributes and labels and the low-dimensional intrinsic deep representations are ensured by further considering low-rank constraints. Moreover, a triplet term that connects instances representations, label representations, and labels is constructed to increase discrimination power of our method. Experimental results conducted on a large-scale micro-video dataset illustrate our model achieves superior performance in comparison with state-of-the-art methods.
更多查看译文
关键词
Matrix decomposition, Feature extraction, Collaboration, Covariance matrices, Task analysis, Correlation, Semantics, Micro-video, multi-label classification, deep matrix factorization, low-rank
AI 理解论文
溯源树
样例
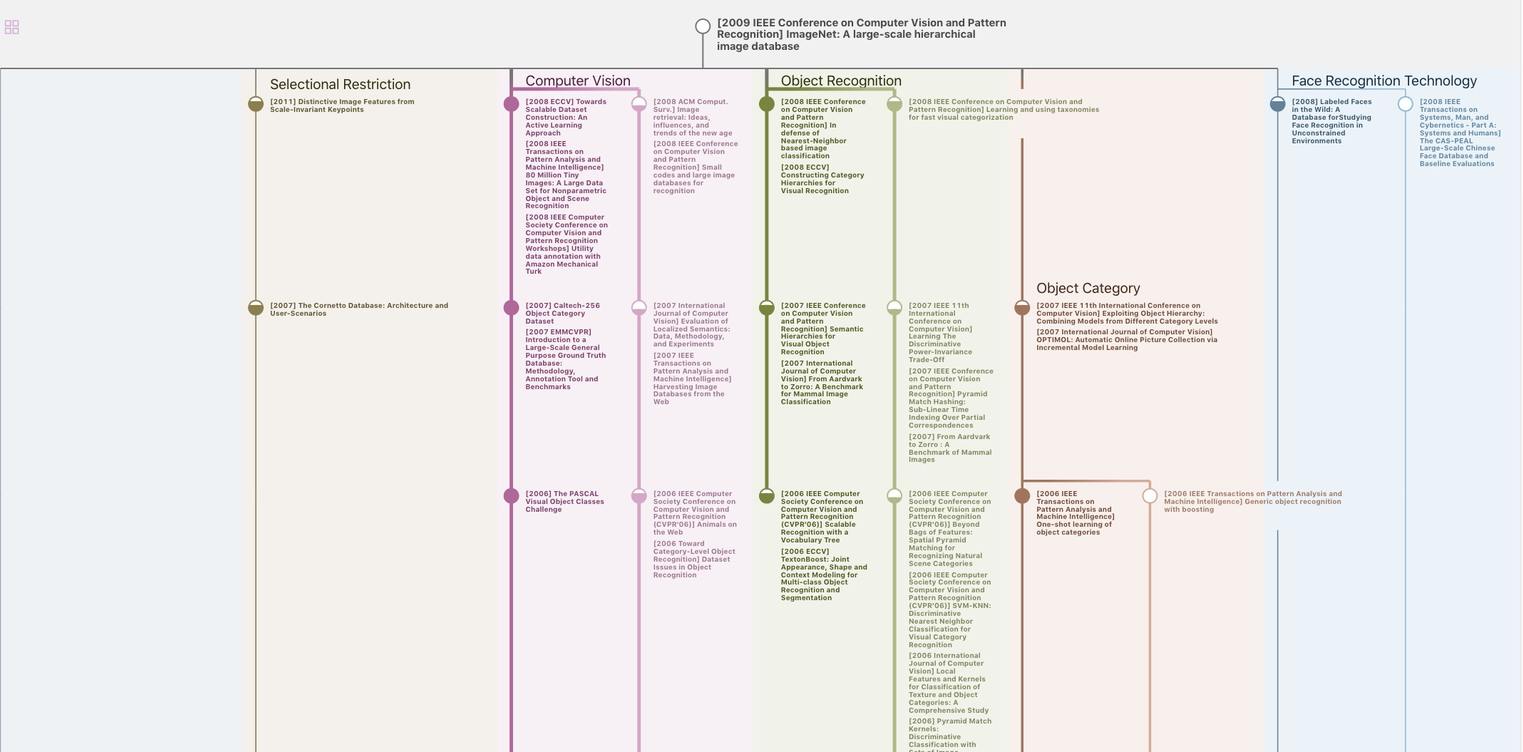
生成溯源树,研究论文发展脉络
Chat Paper
正在生成论文摘要