Combining instance and feature neighbours for extreme multi-label classification
INTERNATIONAL JOURNAL OF DATA SCIENCE AND ANALYTICS(2020)
摘要
Extreme multi-label classification problems occur in different applications such as prediction of tags or advertisements. We propose a new algorithm that predicts labels using a linear ensemble of labels from instance- and feature-based nearest neighbours. In the feature-based nearest neighbours method, we precompute a matrix containing the similarities between each feature and label. For the instance-based nearest neighbourhood, we create an algorithm that uses an inverted index to compute cosine similarity on sparse datasets efficiently. We extend this baseline with a new top- k query algorithm that combines term-at-a-time and document-at-a-time traversal with tighter pruning based on a partition of the dataset. On ten real-world datasets, we find that our method outperforms state-of-the-art methods such as multi-label k -nearest neighbours, instance-based logistic regression, binary relevance with support vector machines and FastXml on different evaluation metrics. We also find that our algorithm is orders of magnitude faster than these baseline algorithms on sparse datasets and requires less than 20 ms per instance to predict labels for extreme datasets without the need for expensive hardware.
更多查看译文
关键词
k -nearest neighbours,Top- k queries
AI 理解论文
溯源树
样例
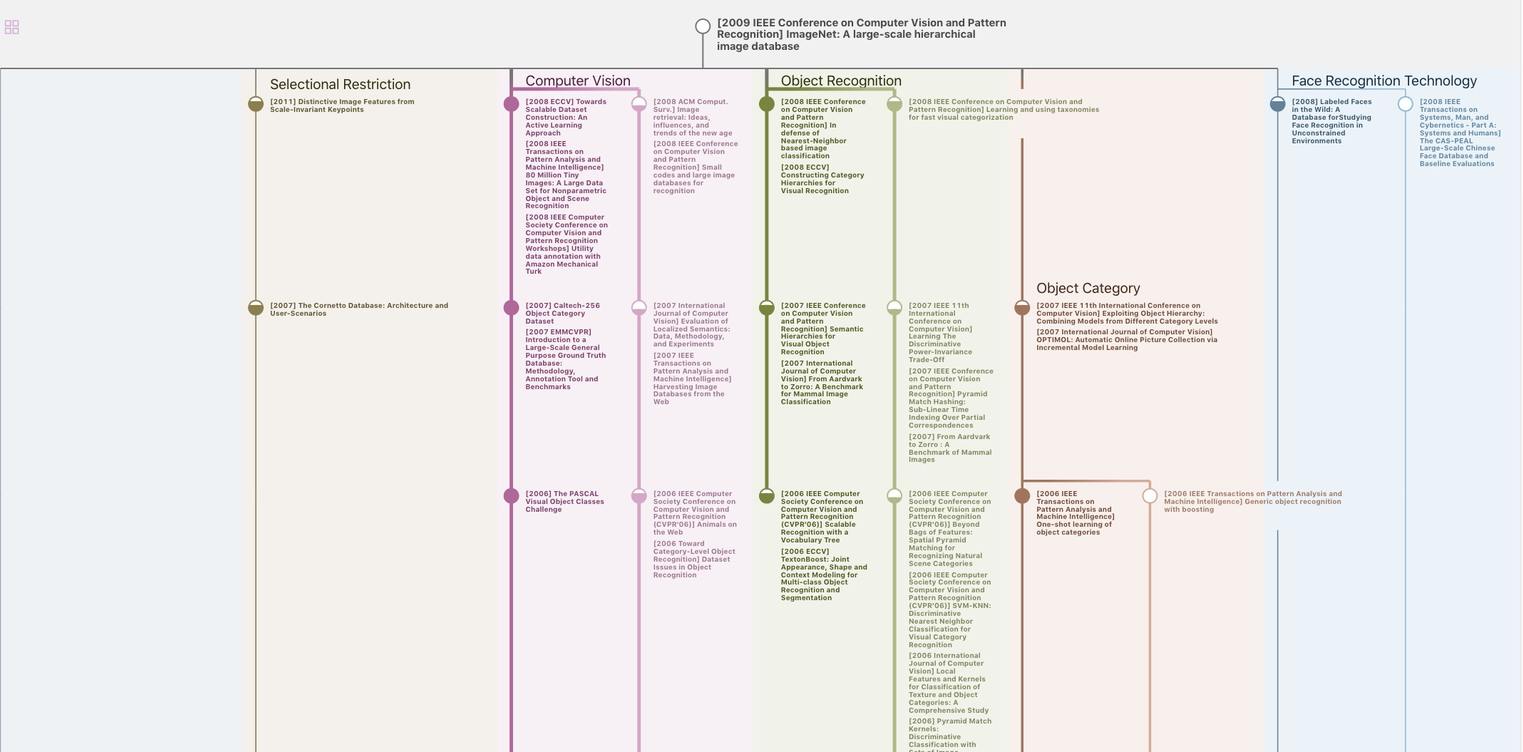
生成溯源树,研究论文发展脉络
Chat Paper
正在生成论文摘要