Quantum-Based Feature Selection for Multiclassification Problem in Complex Systems with Edge Computing
COMPLEXITY(2020)
摘要
The complex systems with edge computing require a huge amount of multifeature data to extract appropriate insights for their decision making, so it is important to find a feasible feature selection method to improve the computational efficiency and save the resource consumption. In this paper, a quantum-based feature selection algorithm for the multiclassification problem, namely, QReliefF, is proposed, which can effectively reduce the complexity of algorithm and improve its computational efficiency. First, all features of each sample are encoded into a quantum state by performing operations CMP and R-y, and then the amplitude estimation is applied to calculate the similarity between any two quantum states (i.e., two samples). According to the similarities, the Grover-Long method is utilized to find the nearest k neighbor samples, and then the weight vector is updated. After a certain number of iterations through the above process, the desired features can be selected with regards to the final weight vector and the threshold tau. Compared with the classical ReliefF algorithm, our algorithm reduces the complexity of similarity calculation from O(MN) to O(M), the complexity of finding the nearest neighbor from O(M) to OM, and resource consumption from O(MN) to O(MlogN). Meanwhile, compared with the quantum Relief algorithm, our algorithm is superior in finding the nearest neighbor, reducing the complexity from O(M) to OM. Finally, in order to verify the feasibility of our algorithm, a simulation experiment based on Rigetti with a simple example is performed.
更多查看译文
AI 理解论文
溯源树
样例
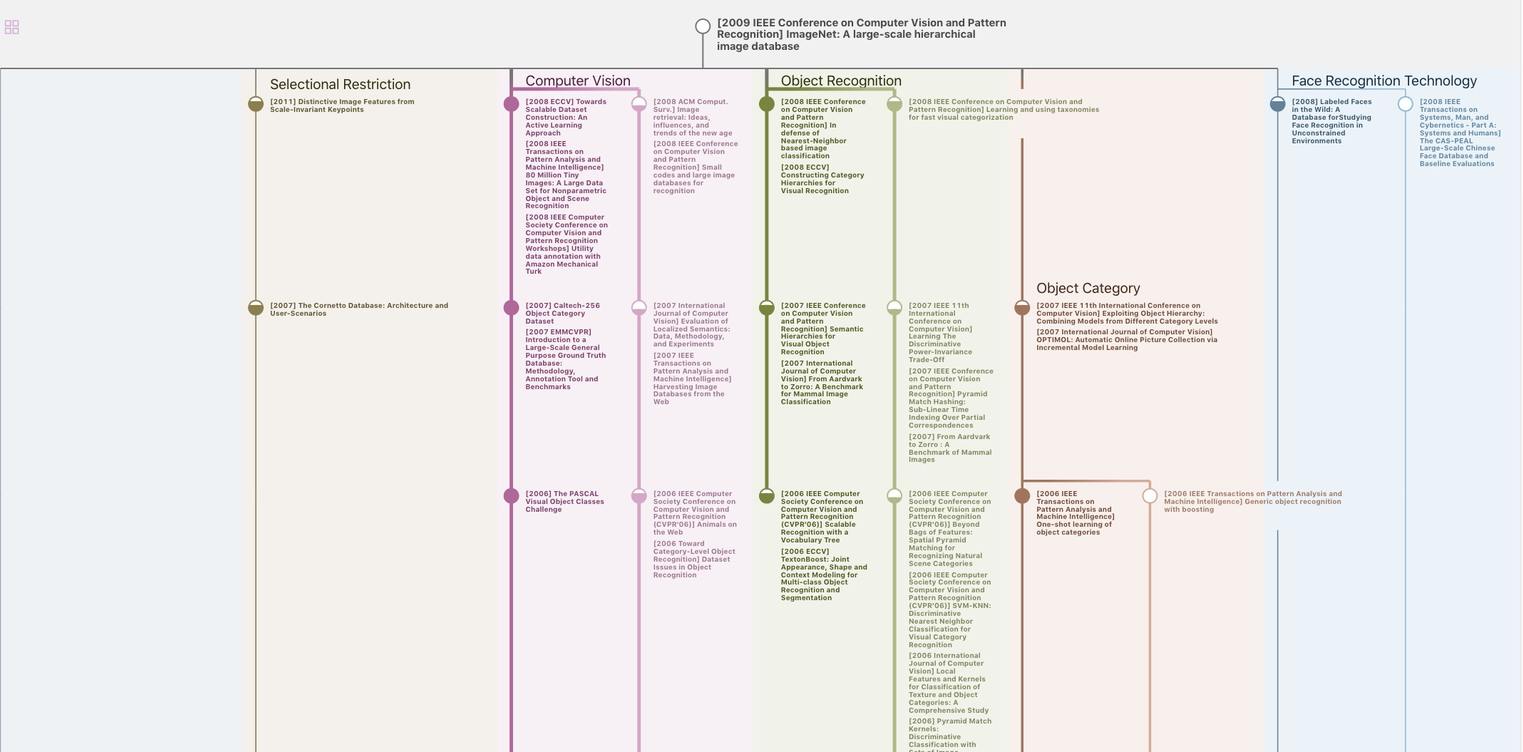
生成溯源树,研究论文发展脉络
Chat Paper
正在生成论文摘要