Deep Reinforcement Learning for Pedestrian Collision Avoidance and Human-Machine Cooperative Driving
Information Sciences(2020)
摘要
With the development of intelligent driving technology, human-machine cooperative driving is significant to improve driving safety in abnormal situations, such as distraction or incorrect operations of drivers. For human-machine cooperative driving, the capacity of pedestrian collision avoidance is fundamental and important. This paper proposes a novel learning-based human-machine cooperative driving scheme (L-HMC) with active collision avoidance capacity using deep reinforcement learning. Firstly, an improved deep Q-network (DQN) method is designed to learn the optimal driving policy for pedestrian collision avoidance. In the improved DQN method, two replay buffers with nonuniform samples are designed to shorten the learning process of the optimal driving policy. Then, a human-machine cooperative driving scheme is proposed to assist human drivers with the learned driving policy for pedestrian collision avoidance when the driving behavior of human drivers is dangerous to the pedestrian. The effectiveness of the human-machine cooperative driving scheme is verified on the simulation platform PreScan using a real vehicle dynamic model. The results demonstrate that the deep reinforcement learning-based method can learn an effective driving policy for pedestrian collision avoidance with a fast convergence rate. Meanwhile, the proposed human-machine cooperative driving scheme L-HMC can avoid potential pedestrian collisions through flexible policies in typical scenarios, therefore improving driving safety.
更多查看译文
关键词
Human-machine cooperative driving,Pedestrian collision avoidance,Deep reinforcement learning,Deep Q-network (DQN)
AI 理解论文
溯源树
样例
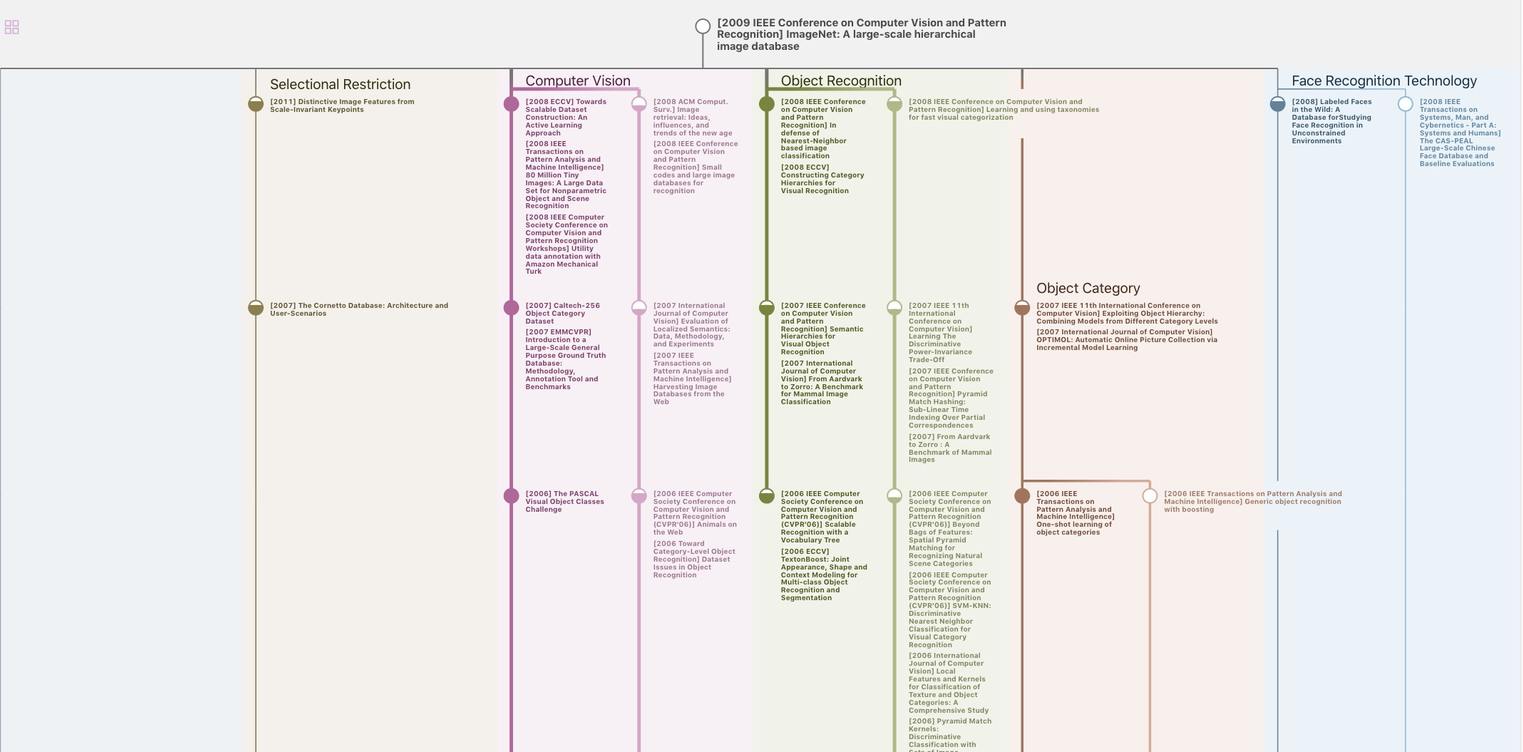
生成溯源树,研究论文发展脉络
Chat Paper
正在生成论文摘要