Quantization-based hashing with optimal bits for efficient recommendation
MULTIMEDIA TOOLS AND APPLICATIONS(2020)
摘要
Recommendation technique has been widely applied in e-commerce systems, but the efficiency becomes challenging due to the growing scale of users and items. In recent years, several hashing-based recommendation frameworks were proposed to solve the efficiency issue successfully by representing users and items with binary codes. These hashing methods consist of two types: two-stage hashing and learning-based hashing. In this paper, we focus on putting forward to a two-stage hashing called quantization-based hashing (QBH) to alleviate the efficiency bottleneck and improve the recommendation accuracy as well. To be specific, we propose the QBH that consists of similarity quantization and norm quantization. To improve the accuracy performance, we search the optimal bits of quantization by minimizing a quantization loss function. We finally evaluate the proposed method on three public datasets to show its superiority on recommendation accuracy over other two-stage hashing methods and advantage on recommendation efficiency over the state-of-the-art recommender systems.
更多查看译文
关键词
Recommender system,Quantization-based hashing,Recommendation efficiency,Matrix factorization
AI 理解论文
溯源树
样例
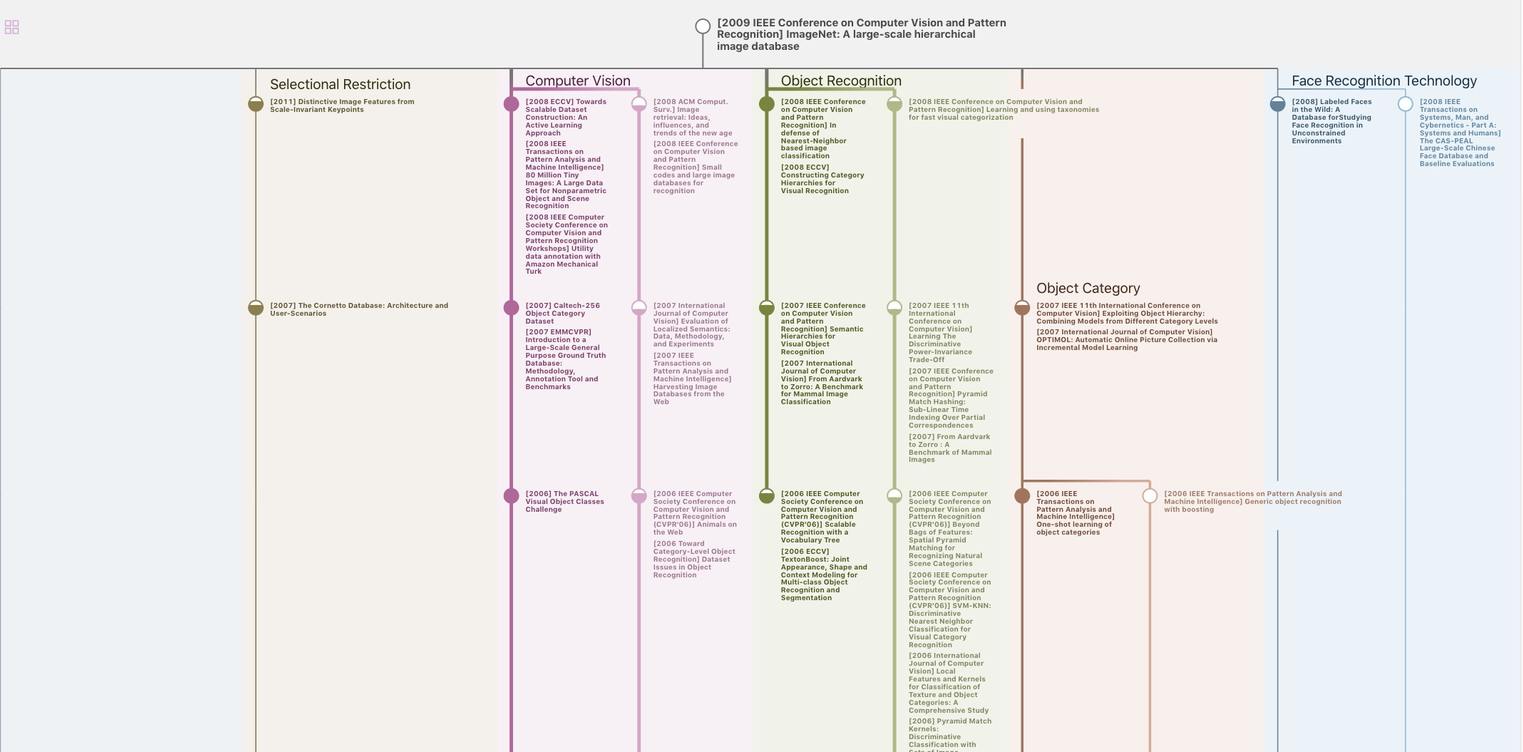
生成溯源树,研究论文发展脉络
Chat Paper
正在生成论文摘要