Application of Convolutional and Recurrent Neural Networks for Buried Threat Detection Using Ground Penetrating Radar Data
IEEE Transactions on Geoscience and Remote Sensing(2020)
摘要
We propose discrimination algorithms for buried threat detection (BTD) that exploit deep convolutional neural networks (CNNs) and recurrent neural networks (RNN) to analyze 2-D GPR B-scans in the down-track (DT) and cross-track (CT) directions as well as 3-D GPR volumes. Instead of imposing a specific model or handcrafted features, as in most existing detectors, we use large real GPR data collections and data-driven approaches that learn: 1) features characterizing buried explosive objects (BEOs) in 2-D B-scans, both in the DT and CT directions; 2) the variation of the CNN features learned in a fixed 2-D view across the third dimension; and 3) features characterizing BEOs in the original 3-D space. The proposed algorithms were trained and evaluated using large experimental GPR data covering a surface area of 120 000 m
2
from 13 different lanes across two U.S. test sites. These data include a diverse set of BEOs consisting of varying shapes, metal content, and underground burial depths. We provide some qualitative analysis of the proposed algorithms by visually comparing their performance and consistency along different dimensions and visualizing typical features learned by some nodes of the network. We also provide quantitative analysis that compares the receiver operating characteristics (ROCs) obtained using the proposed algorithms with those obtained using existing approaches based on CNN as well as traditional learning.
更多查看译文
关键词
Ground penetrating radar,Feature extraction,Computed tomography,Antenna arrays,Recurrent neural networks,Deep learning,Explosives
AI 理解论文
溯源树
样例
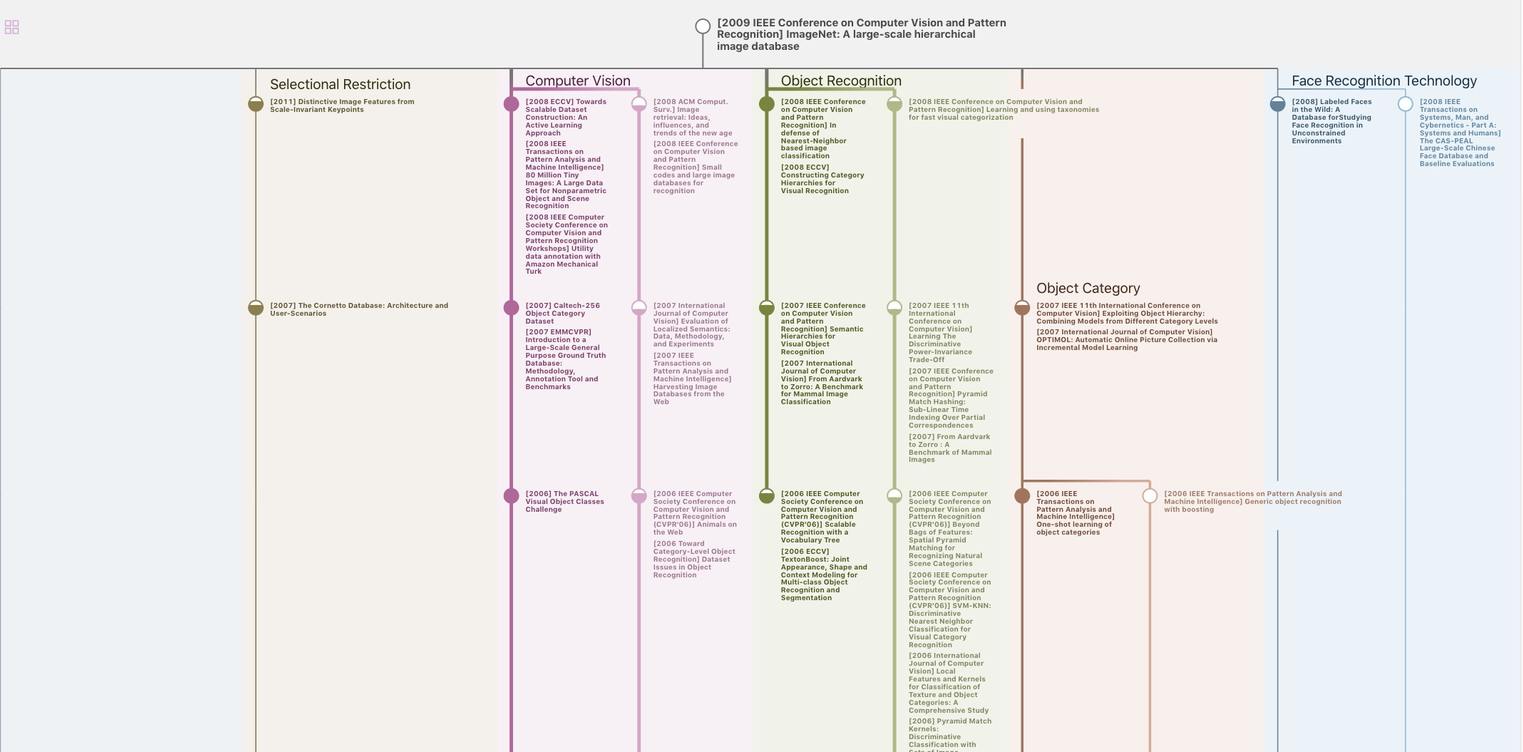
生成溯源树,研究论文发展脉络
Chat Paper
正在生成论文摘要