Multi-step medical image segmentation based on reinforcement learning
J. Ambient Intell. Humaniz. Comput.(2020)
摘要
Image segmentation technology has made a remarkable effect in medical image analysis and processing, which is used to help physicians get a more accurate diagnosis. Manual segmentation of the medical image requires a lot of effort by professionals, which is also a subjective task. Therefore, developing an advanced segmentation method is an essential demand. We propose an end-to-end segmentation method for medical images, which mimics physicians delineating a region of interest (ROI) on the medical image in a multi-step manner. This multi-step operation improves the performance from a coarse result to a fine result progressively. In this paper, the segmentation process is formulated as a Markov decision process and solved by a deep reinforcement learning (DRL) algorithm, which trains an agent for segmenting ROI in images. The agent performs a serial action to delineate the ROI. We define the action as a set of continuous parameters. Then, we adopted a DRL algorithm called deep deterministic policy gradient to learn the segmentation model in continuous action space. The experimental result shows that the proposed method has 7.24% improved to the state-of-the-art method on three prostate MR data sets and has 3.52% improved on one retinal fundus image data set.
更多查看译文
关键词
Reinforcement learning,Deep deterministic policy gradient,Image segmentation,Multi-step manner
AI 理解论文
溯源树
样例
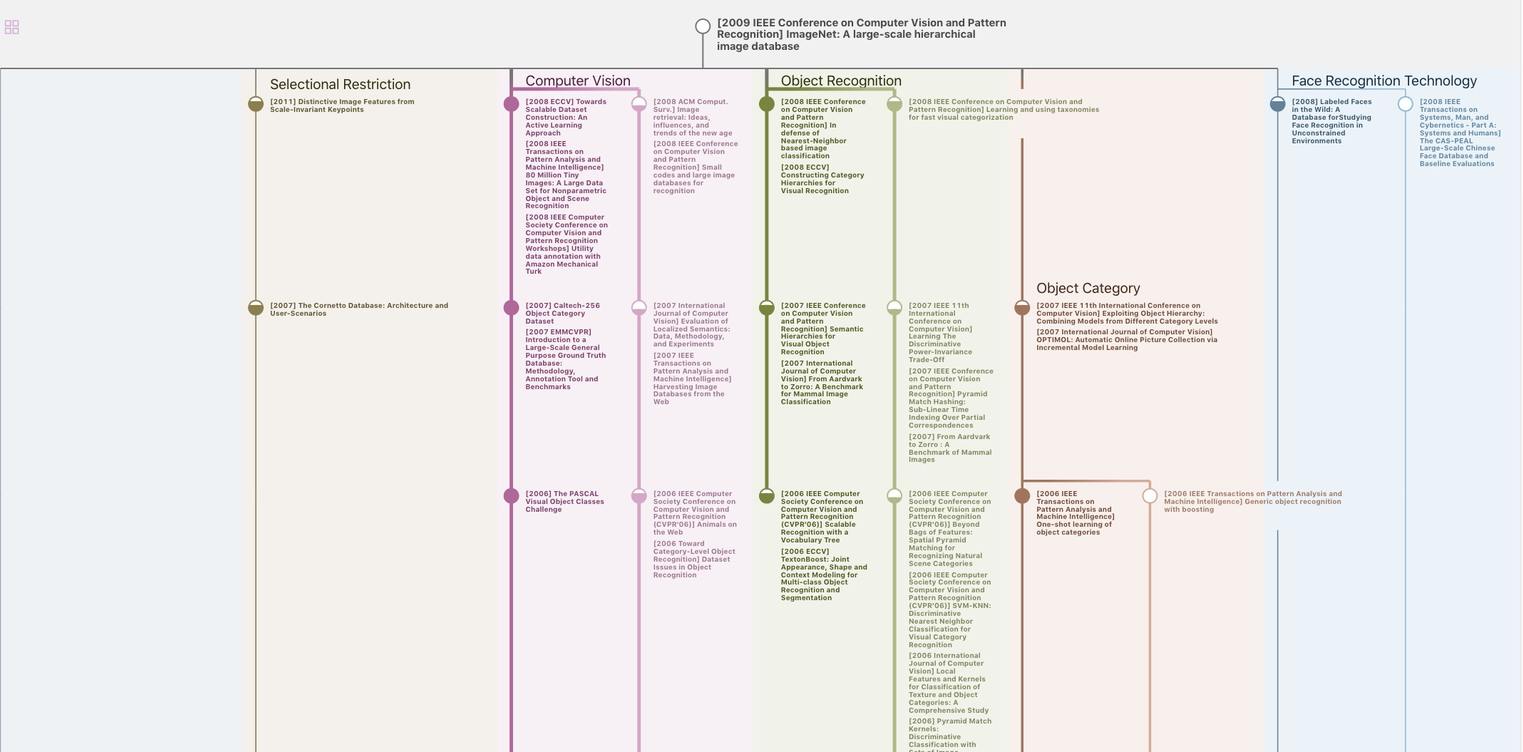
生成溯源树,研究论文发展脉络
Chat Paper
正在生成论文摘要