Session-aware news recommendations using random walks on time-evolving heterogeneous information networks
User Modeling and User-Adapted Interaction(2020)
摘要
Traditional news media Web sites usually provide generic recommendations that are not personalized to the preferences of their users. Typically, news recommendation algorithms mainly rely on the long-term preferences of users and do not adjust their model to the continuous stream of short-lived incoming stories to capture short-term intentions revealed by users’ sessions. In this paper, we therefore study the problem of session-aware recommendations by running random walks on dynamic heterogeneous graphs. Concretely, we construct a heterogeneous information network consisting of users, news articles, news categories, locations and sessions. By using different (1) sliding time window sizes, (2) sub-graphs for model learning, (3) sequential article weighting strategies and (4) more diversified random walks, we perform recommendations in a second step. Our algorithm proposal is evaluated on three real-life data sets, and we demonstrate that our method outperforms state-of-the-art methods by delivering more accurate and diversified recommendations.
更多查看译文
关键词
Link prediction, News recommendation, Heterogeneous information networks
AI 理解论文
溯源树
样例
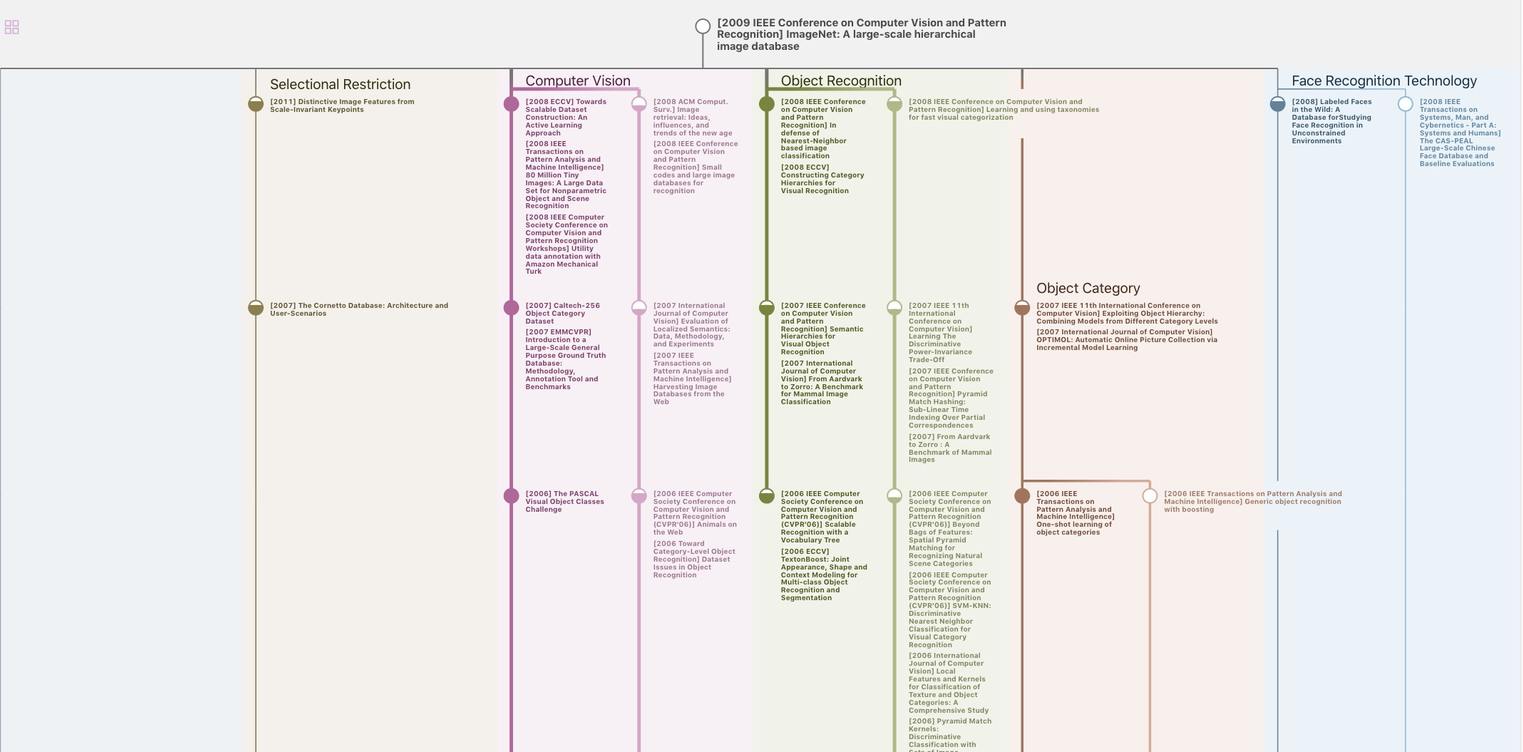
生成溯源树,研究论文发展脉络
Chat Paper
正在生成论文摘要