A Discriminative Distillation Network for Cross-Source Remote Sensing Image Retrieval
IEEE JOURNAL OF SELECTED TOPICS IN APPLIED EARTH OBSERVATIONS AND REMOTE SENSING(2020)
摘要
Nowadays, several remote sensing image capturing technologies are used ranging from unmanned aerial vehicles to satellites. Powerful learning-based discriminative features play an essential role in content-based remote sensing image retrieval (CBRSIR). Cross-source CBRSIR (CS-CBRSIR) is used to find relevant remote sensing images across different remote sensing sources (i.e., multispectral images and panchromatic images). But it is limited by large cross-source and intrasource variations caused by different semantic objects, spatial resolution, and spectral resolution. The main limitation of CS-CBRSIR is that it cannot address the inconsistency between different sources and exploit the intrinsic relation between them. This study proposes a discriminative distillation network for CS-CBRSIR to address this limitation. To enlarge the interclass variations and reduce the intraclass differences, the discriminative features from the first source are first extracted with a well-designed joint optimization configuration (JOC) on the basis of deep neural networks. Thereafter, the features extracted from the first source are used as a supervision signal for the second source; feature distribution in common feature space between the first and second sources are made significantly similar. The method proposed in this study simultaneously handles the cross-source and intersource variations, unlike the existing methods. Extensive experiments on the DSRSID dataset with Euclidean distance verify the effectiveness of our proposed method.
更多查看译文
关键词
Cross-source content-based remote sensing image retrieval (CS-CBRSIR),discriminative features,distillation network,joint optimization configuration (JOC)
AI 理解论文
溯源树
样例
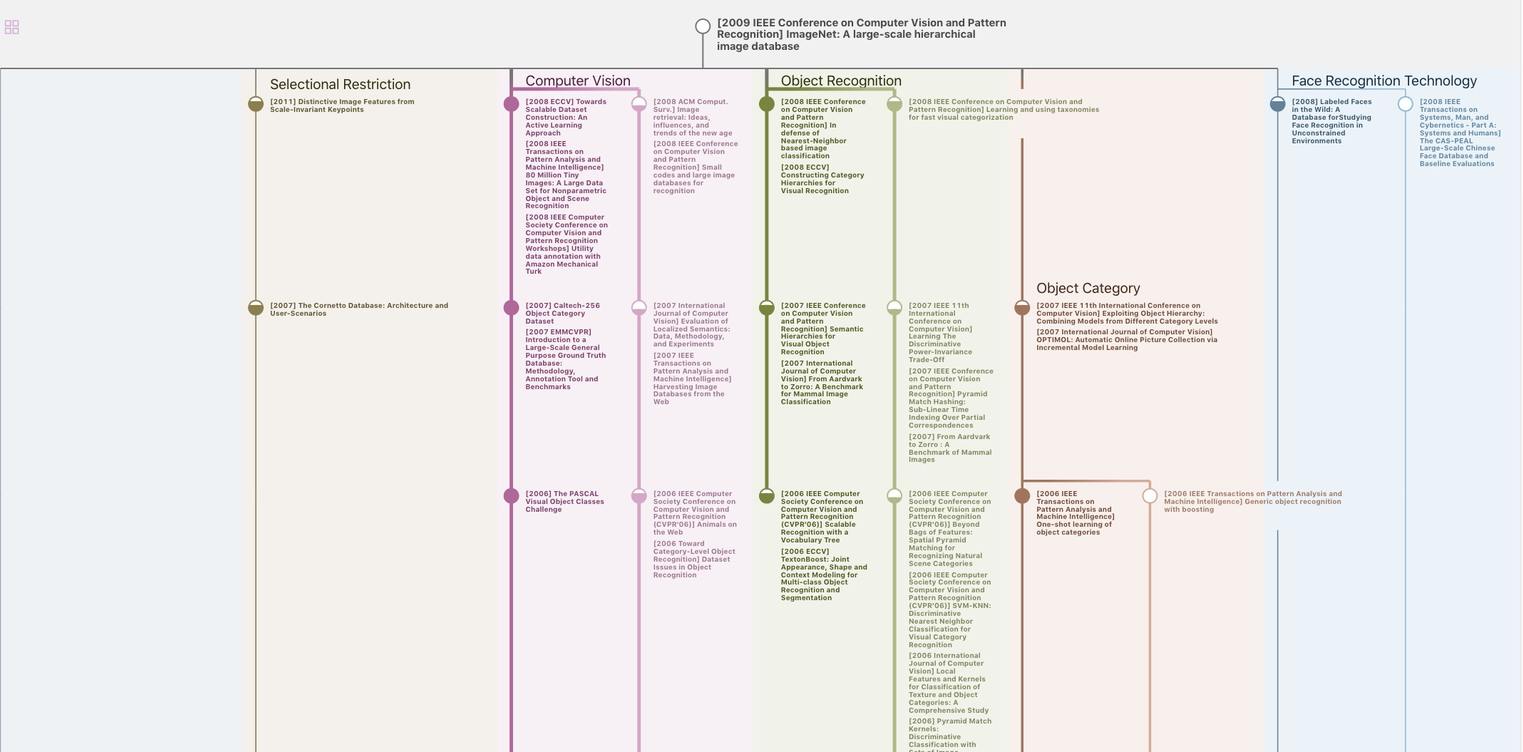
生成溯源树,研究论文发展脉络
Chat Paper
正在生成论文摘要