Airspace Capacity Overload Identification Using Collision Risk Patterns
2020 International Conference on Artificial Intelligence and Data Analytics for Air Transportation (AIDA-AT)(2020)
摘要
The ever increasing demand for air travel may induce en-route airspace capacity overload which endangers flight safety and elicit air traffic congestion. Knowledge of airspace capacity overload is important for air traffic flow management and flight planning to mitigate air traffic congestion without compromising airspace safety level. Since the primary task of air traffic controllers is to manage traffic flow within the constraints imposed by safety requirements, i.e., to warrant the collision risk at a low level, in this paper, we use the aircraft mid-air collision risk for a given airspace as the indicator of airspace capacity overload. With given air traffic data and airspace configurations, the collision risk distributions inside an airspace is determined through collision risk modelling. Based on the density and intensity of collision risk, the collision risk distributions are converted into heatmaps and collision risk patterns are further recognized from the heatmaps using image processing technique. Three major states of airspace workload can be identified from theses patterns: normal state, transition state and overload state. For new traffic data during a given time period, by matching its collision risk distribution to the closest collision risk pattern, we are able to identify whether the airspace is overloaded or not. The experimental study in an en-route sector of the Singapore airspace has manifested the ability of the proposed method in collision risk pattern recognition and capacity overload identification.
更多查看译文
关键词
airspace capacity,collision risk,pattern recognition,clustering
AI 理解论文
溯源树
样例
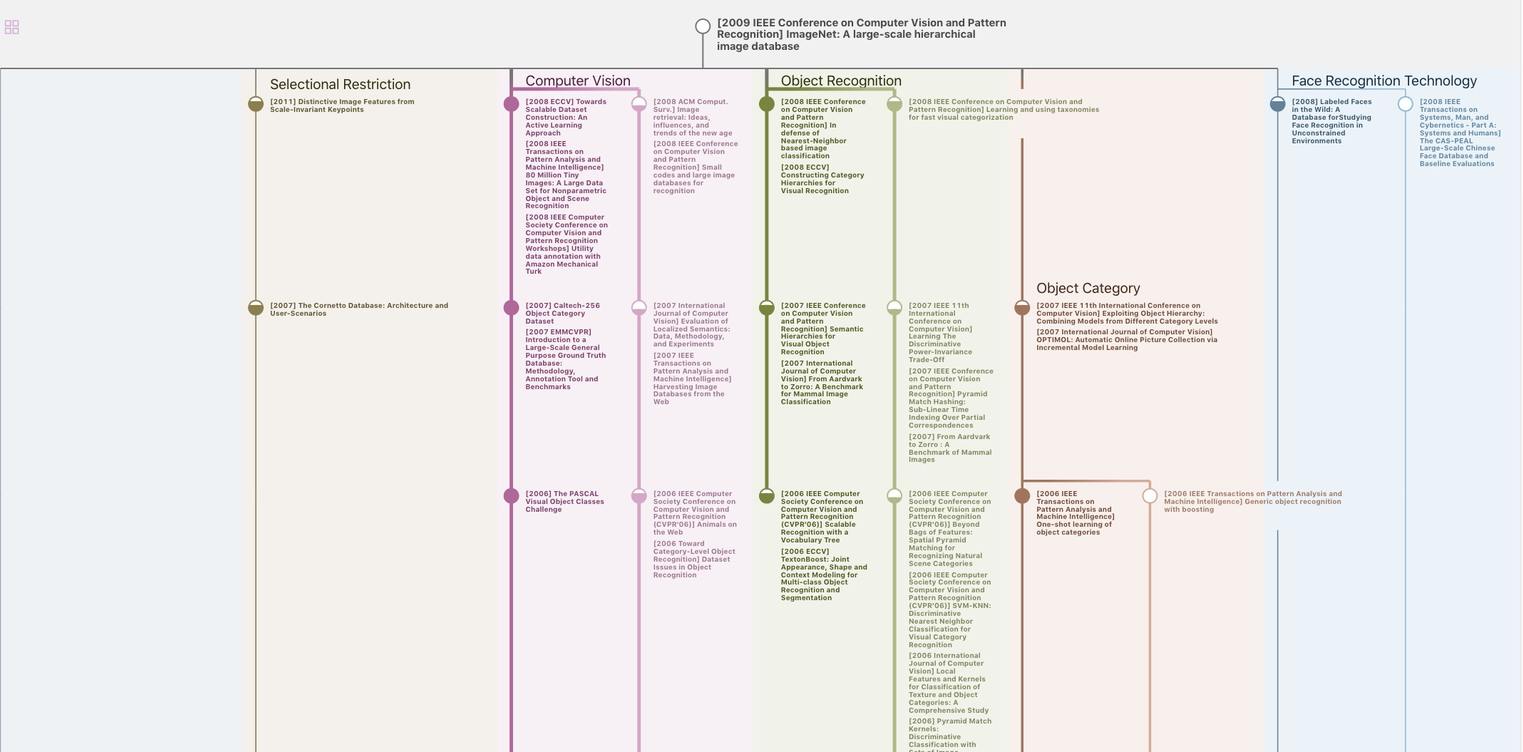
生成溯源树,研究论文发展脉络
Chat Paper
正在生成论文摘要