LTR-expand: query expansion model based on learning to rank association rules
Journal of Intelligent Information Systems(2020)
摘要
Query Expansion (QE) is widely applied to improve the retrieval performance of ad-hoc search, using different techniques and several data sources to find expansion terms. In Information Retrieval literature, selecting expansion terms remains a challenging task that relies on the extraction of term relationships. In this paper, we propose a new learning to rank-based query expansion model. The main idea behind is that, given a query and the set of its related ARs, our model ranks these ARs according to their relevance score regarding to this query and then selects the most suitable ones to be used in the QE process. Experiments are conducted on three test collections, namely: CLEF2003, TREC-Robust and TREC-Microblog, including long, hard and short queries. Results showed that the retrieval performance can be significantly improved when the ARs ranking method is used compared to other state of the art expansion models, especially for hard and long queries.
更多查看译文
关键词
Formal Concept Analysis (FCA),Association Rule (AR),Generic basis,Learning to rank,SVM rank,Query Expansion (QE)
AI 理解论文
溯源树
样例
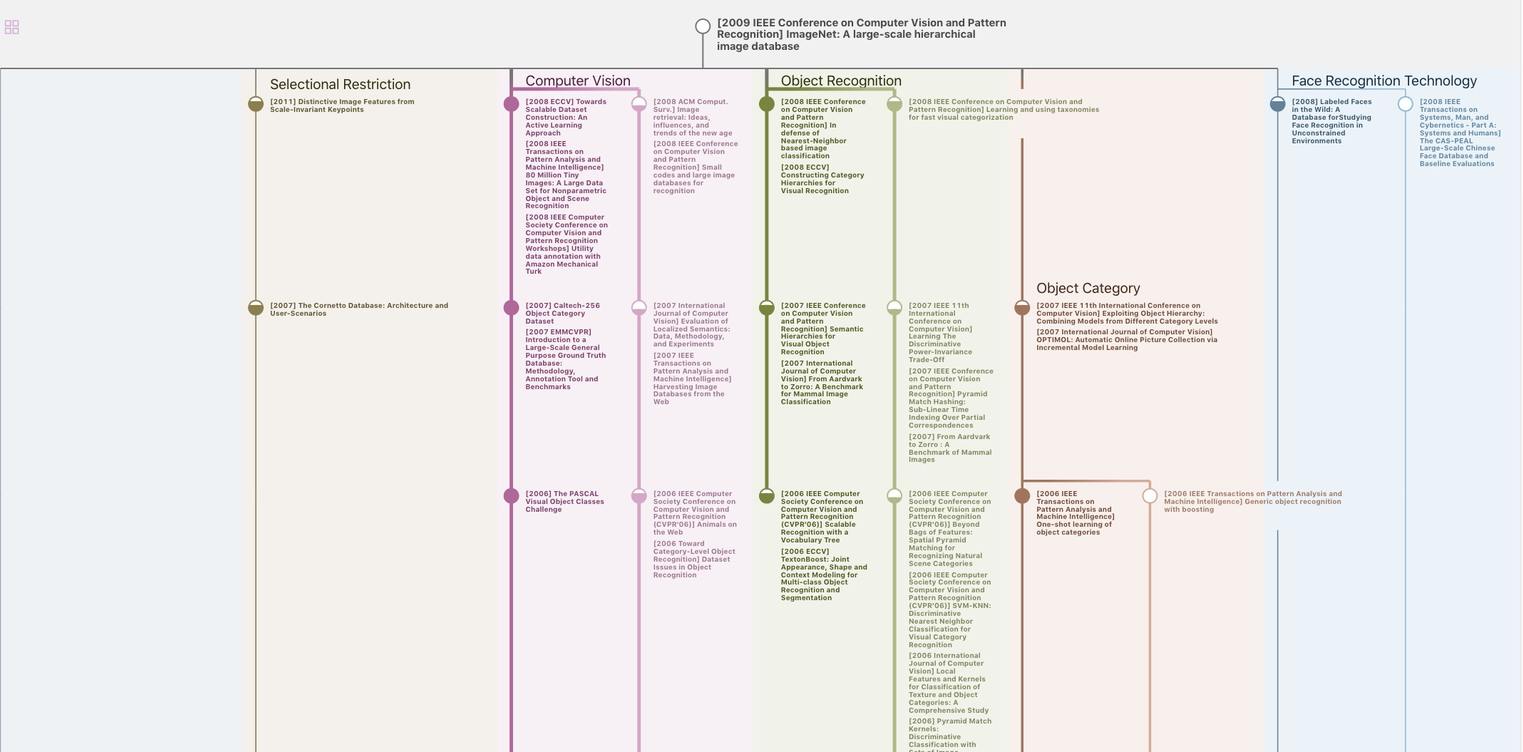
生成溯源树,研究论文发展脉络
Chat Paper
正在生成论文摘要