Persistent Excitation Condition For Mimo Volterra System Identification With Gaussian Distributed Input Signals
2019 IEEE 58TH CONFERENCE ON DECISION AND CONTROL (CDC)(2019)
摘要
A viable approach to the estimation and characterization of non-linear system dynamics on the basis of input/ouput observations of a non-linear system is a parametrization based on a Volterra model. The kernel representation of a Volterra model can approximate a large class of non-linear systems and has the advantage of being linear in the kernel parameters to be estimated. Although the number of kernel parameters typically increases exponentially, the demand for storage requirements during kernel parameter estimation can be relieved via a tensor network technique. This approach allows estimation of high degree and even multi-input multi output (MIMO) Volterra models, which have the potential to capture more complicated non-linear dynamics. This paper gives a persistent excitation condition for the parameter estimation in MIMO Volterra system identification in the case of a zero mean, Gaussian distributed (not necessarily white) input signal. The persistent excitation condition shows that under those input conditions a MIMO Volterra system can be identified consistently via an appropriately sized input signal.
更多查看译文
关键词
persistent excitation condition,MIMO Volterra system identification,Gaussian distributed input signals,nonlinear system dynamics,Volterra model,kernel representation,kernel parameter estimation,multiinput multioutput Volterra models,nonlinear dynamics,tensor network technique
AI 理解论文
溯源树
样例
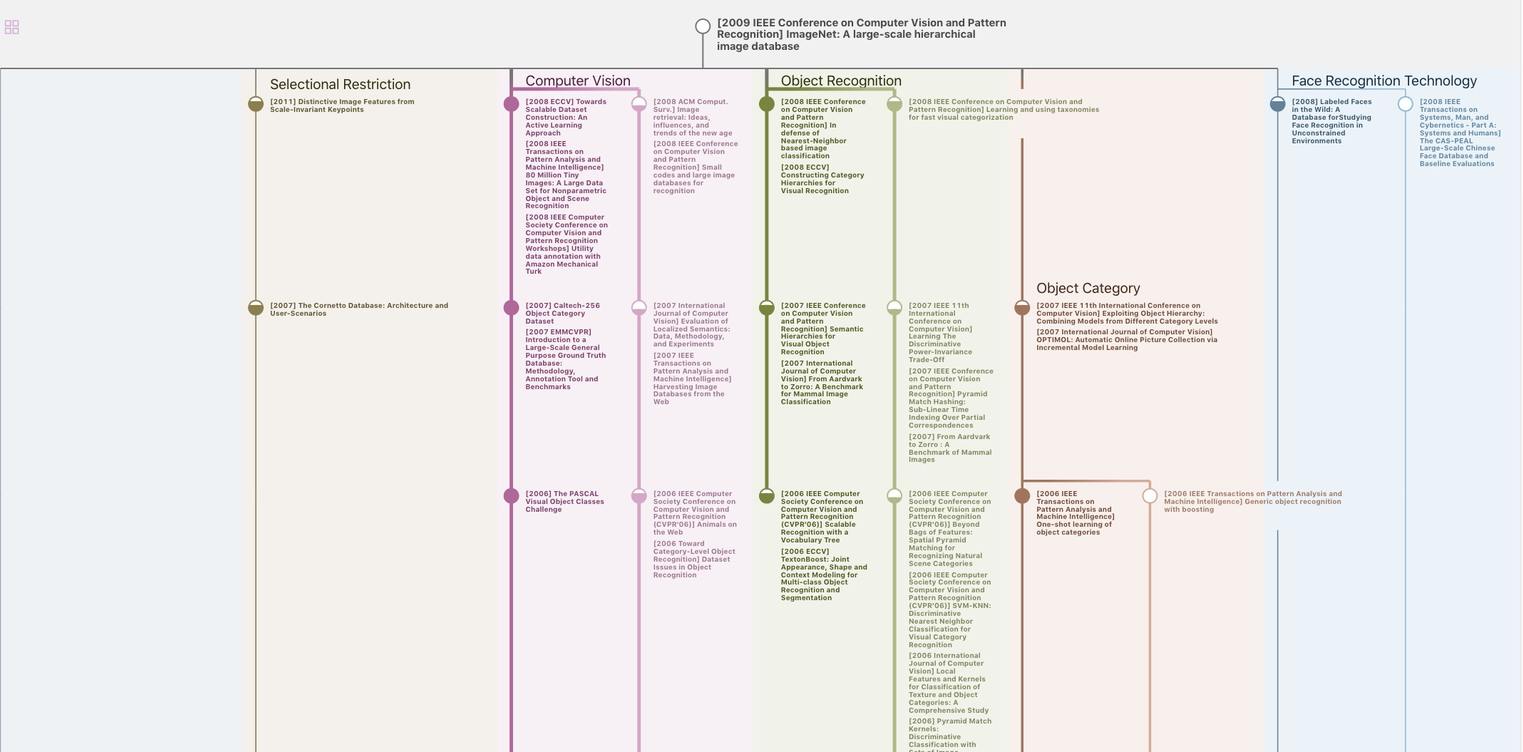
生成溯源树,研究论文发展脉络
Chat Paper
正在生成论文摘要