Automated Redistricting Simulation Using Markov Chain Monte Carlo
JOURNAL OF COMPUTATIONAL AND GRAPHICAL STATISTICS(2020)
摘要
Legislative redistricting is a critical element of representative democracy. A number of political scientists have used simulation methods to sample redistricting plans under various constraints to assess their impact on partisanship and other aspects of representation. However, while many optimization algorithms have been proposed, surprisingly few simulation methods exist in the published scholarship. Furthermore, the standard algorithm has no theoretical justification, scales poorly, and is unable to incorporate fundamental constraints required by redistricting processes in the real world. To fill this gap, we formulate redistricting as a graph-cut problem and for the first time in the literature propose a new automated redistricting simulator based on Markov chain Monte Carlo. The proposed algorithm can incorporate contiguity and equal population constraints at the same time. We apply simulated and parallel tempering to improve the mixing of the resulting Markov chain. Through a small-scale validation study, we show that the proposed algorithm can approximate a target distribution more accurately than the standard algorithm. We also apply the proposed methodology to data from Pennsylvania to demonstrate the applicability of our algorithm to real-world redistricting problems. The open-source software package is available so that researchers and practitioners can implement the proposed methodology. for this article are available online.
更多查看译文
关键词
Gerrymandering, Graph cuts, Metropolis-Hastings algorithm, Parallel tempering, Simulated tempering, Swendsen-Wang algorithm
AI 理解论文
溯源树
样例
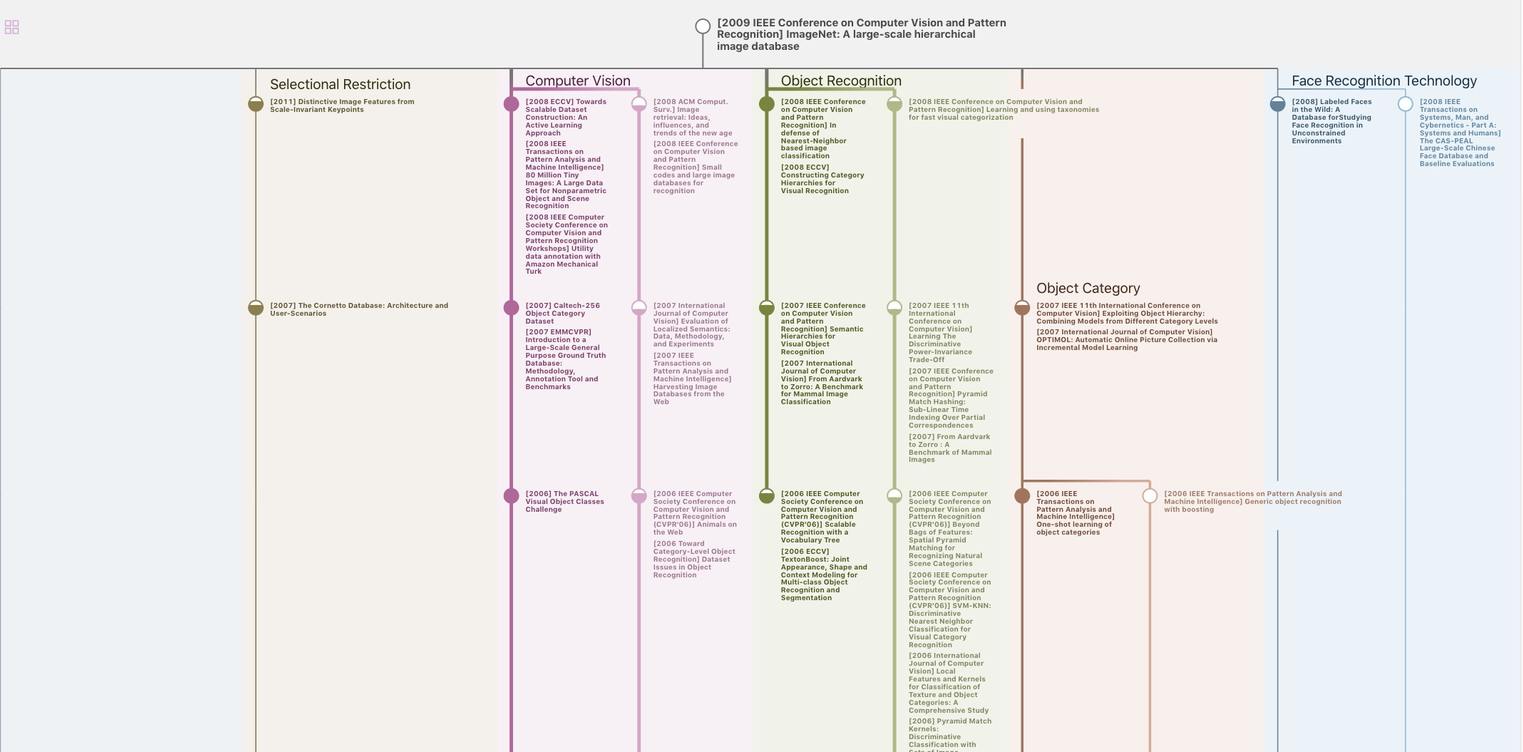
生成溯源树,研究论文发展脉络
Chat Paper
正在生成论文摘要