Complementary Discriminative Correlation Filters Based on Collaborative Representation for Visual Object Tracking
IEEE Transactions on Circuits and Systems for Video Technology(2021)
摘要
In recent years, discriminative correlation filter (DCF) based algorithms have significantly advanced the state of the art in visual object tracking. The key to the success of DCF is an efficient discriminative regression model trained with powerful multi-cue features, including both hand-crafted and deep neural network features. However, the tracking performance is hindered by their inability to respond adequately to abrupt target appearance variations. This issue is posed by the limited representation capability of fixed image features. In this work, we set out to rectify this shortcoming by proposing a complementary representation of a visual content. Specifically, we propose the use of a collaborative representation between successive frames to extract the dynamic appearance information from a target with rapid appearance changes, which results in suppressing the undesirable impact of the background. The resulting collaborative representation coefficients are combined with the original feature maps using a spatially regularised DCF framework for performance boosting. The experimental results on several benchmarking datasets demonstrate the effectiveness and robustness of the proposed method, as compared with a number of state-of-the-art tracking algorithms.
更多查看译文
关键词
Visual object tracking,discriminative correlation filter,feature representation,collaborative representation
AI 理解论文
溯源树
样例
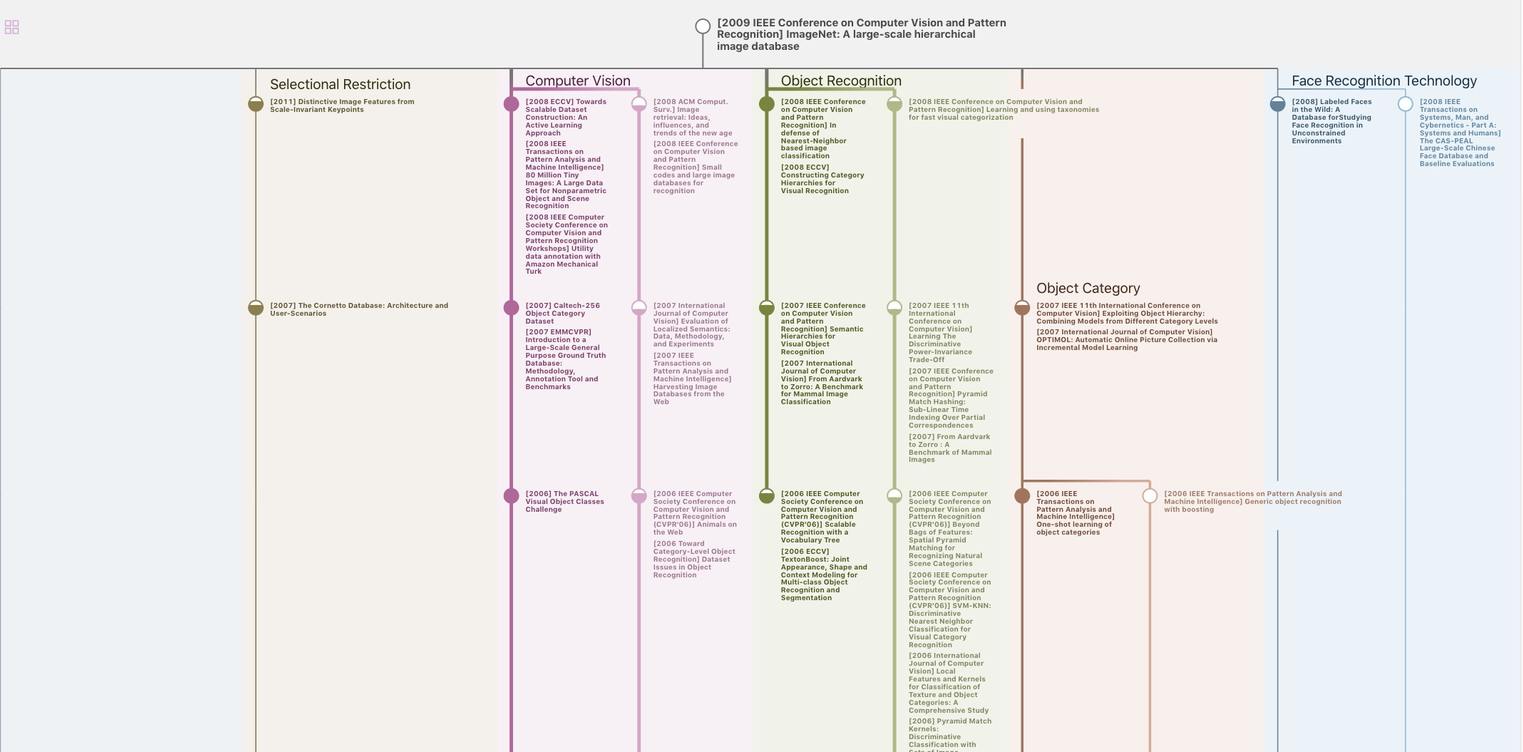
生成溯源树,研究论文发展脉络
Chat Paper
正在生成论文摘要