Empirical Algorithms For General Stochastic Systems With Continuous States And Actions
2019 IEEE 58TH CONFERENCE ON DECISION AND CONTROL (CDC)(2019)
摘要
In this paper, we present Randomized Empirical Value Learning (RAEVL) algorithm for MDPs with continuous state and action spaces. This algorithm combines the ideas of random search over action space with randomized function approximation method to generalize the value functions over state space. Our theoretical analysis is done under a random operator framework combined with stochastic dominance argument. This provides finite-time analysis of the proposed algorithm as well as give the sample complexity.
更多查看译文
关键词
action space,randomized function approximation method,value function generalization,state space,theoretical analysis,random operator framework,stochastic dominance argument,finite-time analysis,empirical algorithms,general stochastic systems,empirical value learning algorithm,RAEVL,continuous state,action spaces,random search,controlled Markov process,complexity
AI 理解论文
溯源树
样例
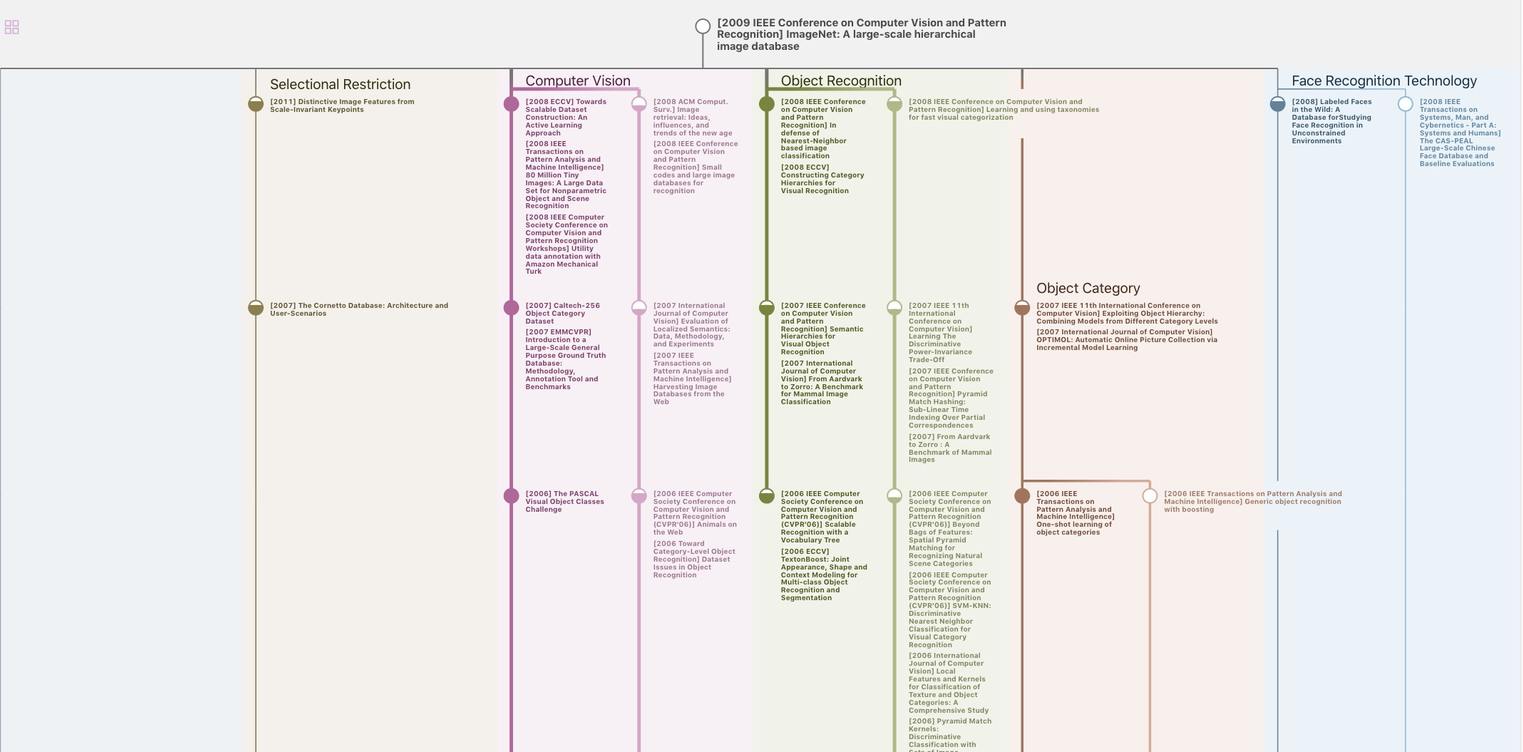
生成溯源树,研究论文发展脉络
Chat Paper
正在生成论文摘要