Persuasion-based recommender system ensambling matrix factorisation and active learning models
Personal and Ubiquitous Computing(2024)
摘要
Recommendation systems are gaining popularity on Internet platforms such as Amazon, Netflix, Spotify or Booking. As more users are joining these online consumer and entertainment sectors, the profile-based data for providing accurate just-in-time recommendations is rising thanks to strategies based on collaborative filtering or content-based metrics. However, these systems merely focus on providing the right item for the users without taking into account what would be the best strategy to suggest the movie, the product or the song (i.e. the strategy to increase the success or impact of the recommendation). Taking this research gap into consideration, this paper proposes a profile-based recommendation system that outputs a set of potential persuasive strategies that can be used with users with similar characteristics. The case study presented provides tailored persuasive strategies to make office-based employees enhance the energy efficiency at work (the dataset used on this research is specific of this sector). Throughout the paper, shreds of evidence are reported assessing the validity of the proposed system. Specifically, two approaches are compared: a profile-based recommendation system (RS) vs. the same RS enriched by adding an ensemble with an active learning model. The results shed light on not only providing effective mechanisms to increase the success of the recommendations but also alleviating the cold start problem when newcomers arrive.
更多查看译文
关键词
Recommender systems,Persuasive strategies,User profile,Workplace,Cold start,Preference recommendations
AI 理解论文
溯源树
样例
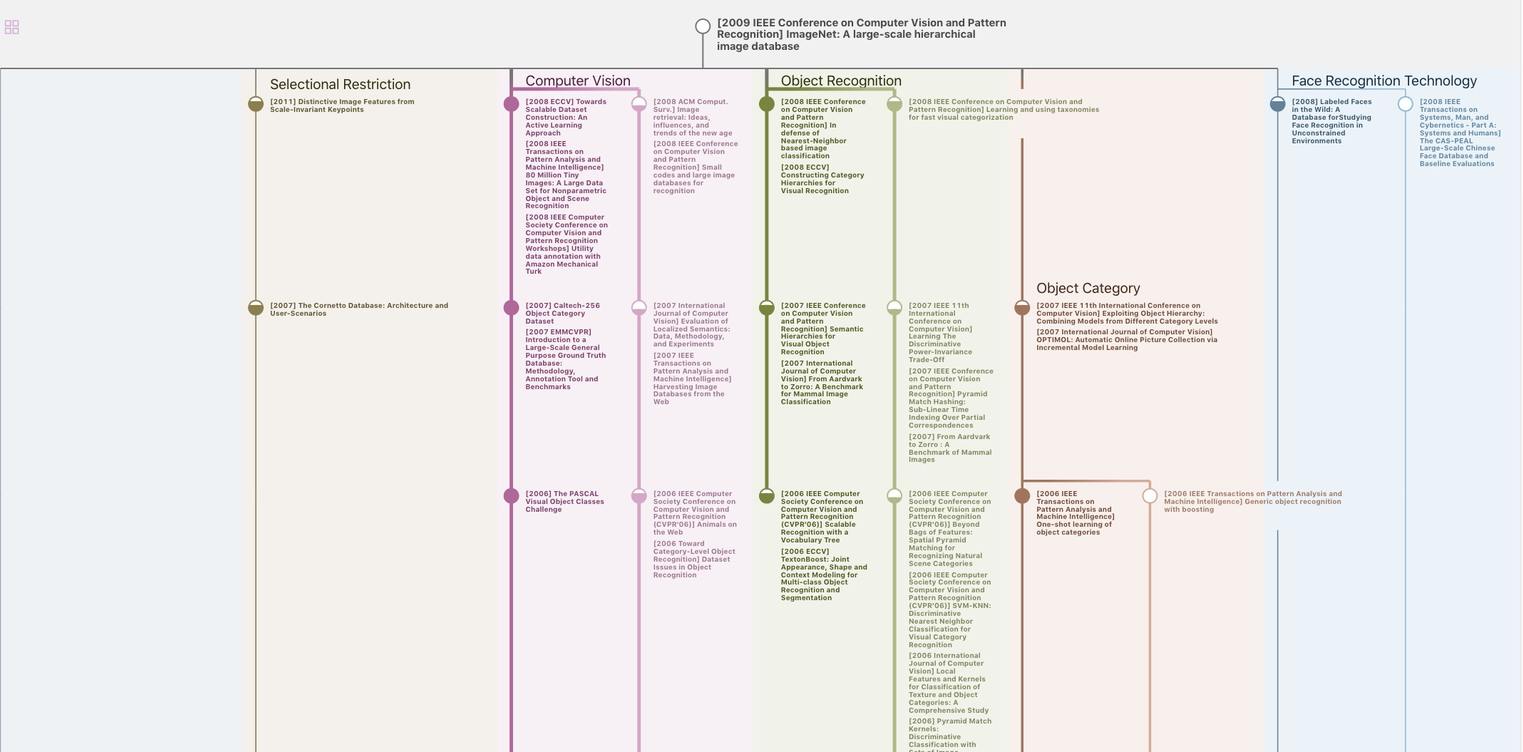
生成溯源树,研究论文发展脉络
Chat Paper
正在生成论文摘要