Re-examination on Rule Based Method in De-identification of Electronic Health Records (Preprint)
JMIR MEDICAL INFORMATICS(2020)
摘要
Background: Deidentification of clinical records is a critical step before their publication. This is usually treated as a type of sequence labeling task, and ensemble learning is one of the best performing solutions. Under the framework of multi-learner ensemble, the significance of a candidate rule-based learner remains an open issue. Objective: The aim of this study is to investigate whether a rule based learner is useful in a hybrid deidentification system and offer suggestions on how to build and integrate a rule-based learner. Methods: We chose a data-driven rule-learner named transformation-based error-driven learning (TBED) and integrated it into the best performing hybrid system in this task. Results: On the popular Informatics for Integrating Biology and the Bedside (i2b2) deidentification data set, experiments showed that TBED can offer high performance with its generated rules, and integrating the rule-based model into an ensemble framework, which reached an F1 score of 96.76%, achieved the best performance reported in the community. Conclusions: We proved the rule-based method offers an effective contribution to the current ensemble learning approach for the deidentification of clinical records. Such a rule system could be automatically learned by TBED, avoiding the high cost and low reliability of manual rule composition. In particular, we boosted the ensemble model with rules to create the best performance of the deidentification of clinical records.
更多查看译文
关键词
ensemble learning,deidentification,transformation-based error-driven rule learner
AI 理解论文
溯源树
样例
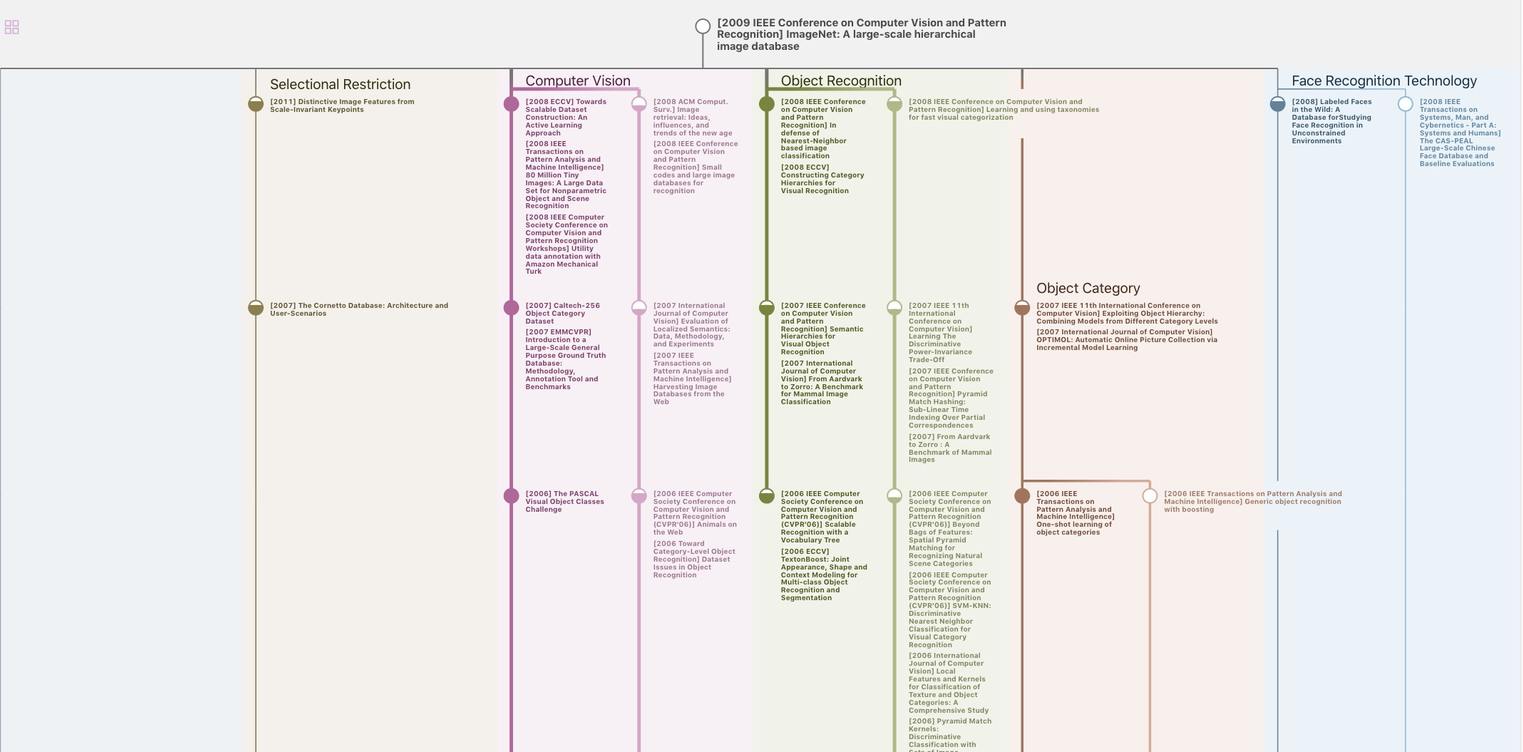
生成溯源树,研究论文发展脉络
Chat Paper
正在生成论文摘要