Adaptive Classifiers: Applied to Radio Waveforms
ICAART: PROCEEDINGS OF THE 12TH INTERNATIONAL CONFERENCE ON AGENTS AND ARTIFICIAL INTELLIGENCE, VOL 2(2020)
摘要
Adaptive classifiers detect previously unknown classes of data, cluster them and adapt itself to classify the newly detected classes without degrading classification performance on known classes. This study explores applying transfer learning from pre-trained CNNs for feature extraction, and adaptive classifier algorithms for predicting radio waveform modulation classes. It is surmised that adaptive classifiers are essential components for cognitive radio and radar systems. Three approaches that use anomaly detection and clustering techniques are implemented for online adaptive RF waveform classification. The use of CNNs is explored because they have been demonstrated previously as highly accurate classifiers on two-dimensional constellation images of RF signals, and because CNNs lend themselves well to transfer learning applications where limited data is available. This study explores replacing the last softmax layer of CNNs with adaptive classifiers to determine if the resulting classifiers can maintain or improve the original accuracy of the CNNs, as well as provide for on-the-fly anomaly detection and clustering in nonstationary RF environments.
更多查看译文
关键词
CNN,Convolutional Neural Networks,Classification,Adaptive,Anomaly,Detection,Radio Waveforms,Modulation,Transfer Learning
AI 理解论文
溯源树
样例
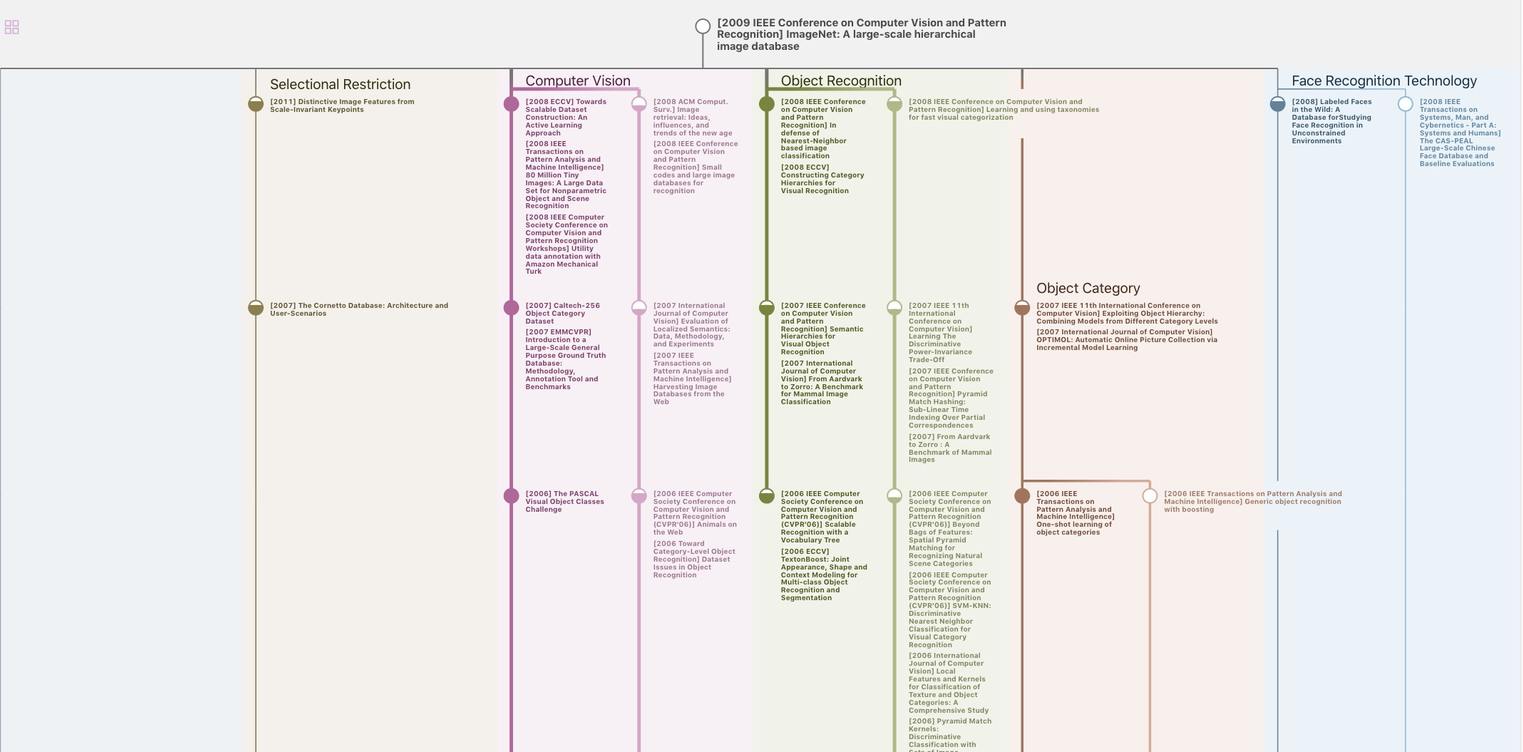
生成溯源树,研究论文发展脉络
Chat Paper
正在生成论文摘要