Construction of Machine-Labeled Data for Improving Named Entity Recognition by Transfer Learning
IEEE ACCESS(2020)
摘要
Deep neural networks (DNNs) require a large amount of manually labeled training data to make significant achievements. However, manual labeling is laborious and costly. In this study, we propose a method for automatically generating training data and effectively using the generated data to reduce the labeling cost. The generated data (called "machine-labeled data") is generated using a bagging-based bootstrapping approach. However, using the machine-labeled data does not guarantee high performance because of errors in the automatic labeling. In order to reduce the impact of mislabeling, we applied a transfer learning approach. The effect of our proposed method was verified with two versions of DNN-based named entity recognition (NER) models: bidirectional LSTM-CRF and vanilla BERT. We conducted NER tasks in two languages (English and Korean). The proposed method results in average F1 scores of 78.87% (3.9% point improvement) with bidirectional LSTM-CRF and 82.08% (1% point improvement) with BERT on three Korean NER datasets. In English, the performance increased by an average of 0.45% points with the two DNN-based models. The proposed NER systems outperform the baseline systems in both languages without the need for additional manual labeling.
更多查看译文
关键词
Named entity recognition,bootstrapping,bagging,transfer learning,deep learning
AI 理解论文
溯源树
样例
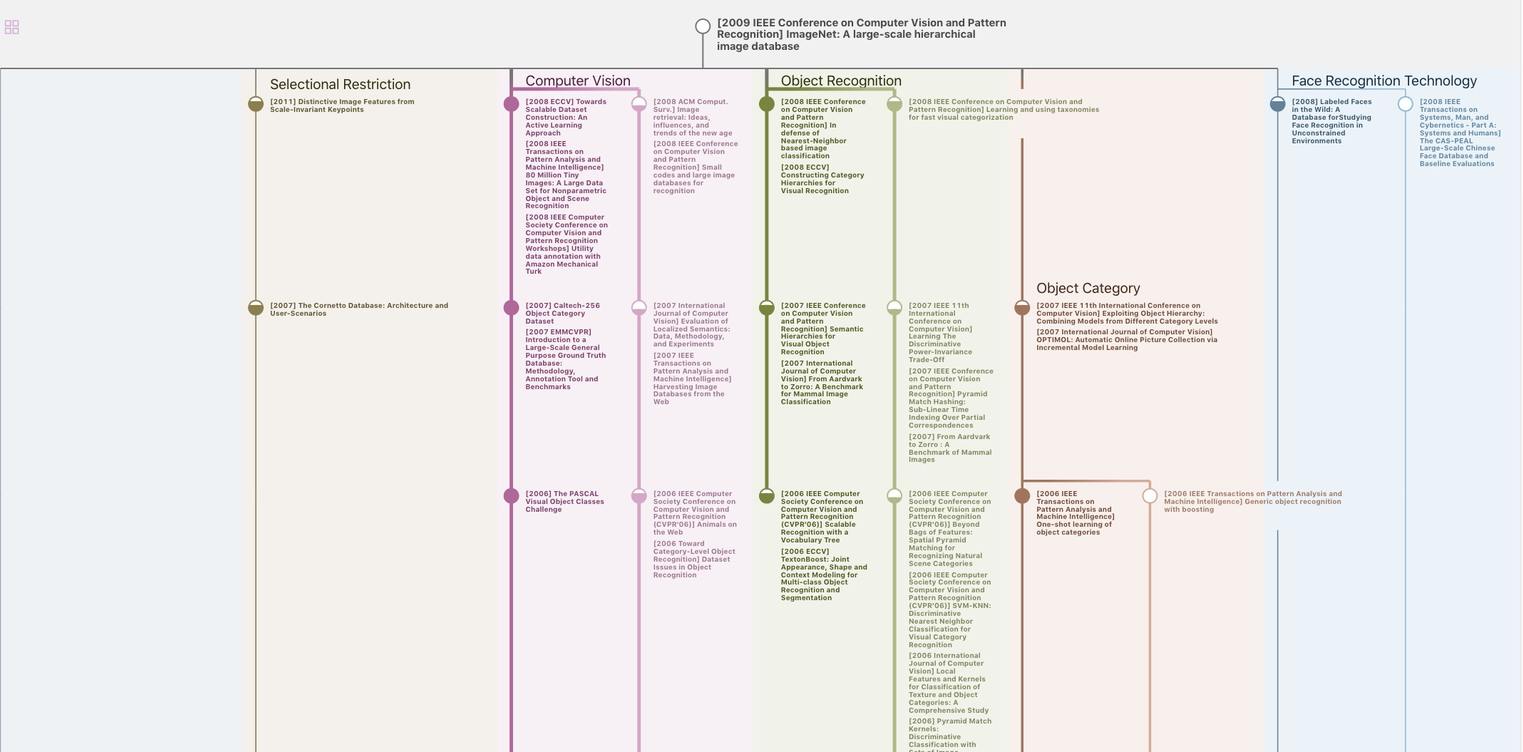
生成溯源树,研究论文发展脉络
Chat Paper
正在生成论文摘要