Quantum Boltzmann Machine Algorithm With Dimension-Expanded Equivalent Hamiltonian
PHYSICAL REVIEW A(2020)
摘要
Quantum machine learning explores the devising and implementing of quantum software to enable the speedup for a classical machine learning counterpart, which has attracted extensive attention. The quantum Boltzmann machine is a promising quantum machine learning model and can be executed on near-term quantum devices to demonstrate quantum supremacy. We investigate an efficient algorithm for training a quantum Boltzmann machine based on a continuous-time quantum cluster Monte Carlo method realized with a dimension-expanded equivalent Hamiltonian. The proposed algorithm is capable of efficiently training various types of quantum Boltzmann machines, including a two-dimensional random transverse Ising Hamiltonian and other stochastic kappa -local (kappa >= 2) Hamiltonians. We further apply a quantum mean-field method to speed up the training process through discarding the quantum fluctuations of visible-hidden interactions. The experiment results show that our algorithm-trained quantum Boltzmann machine is more efficient on a small and large data set in terms of network convergence and time complexity.
更多查看译文
AI 理解论文
溯源树
样例
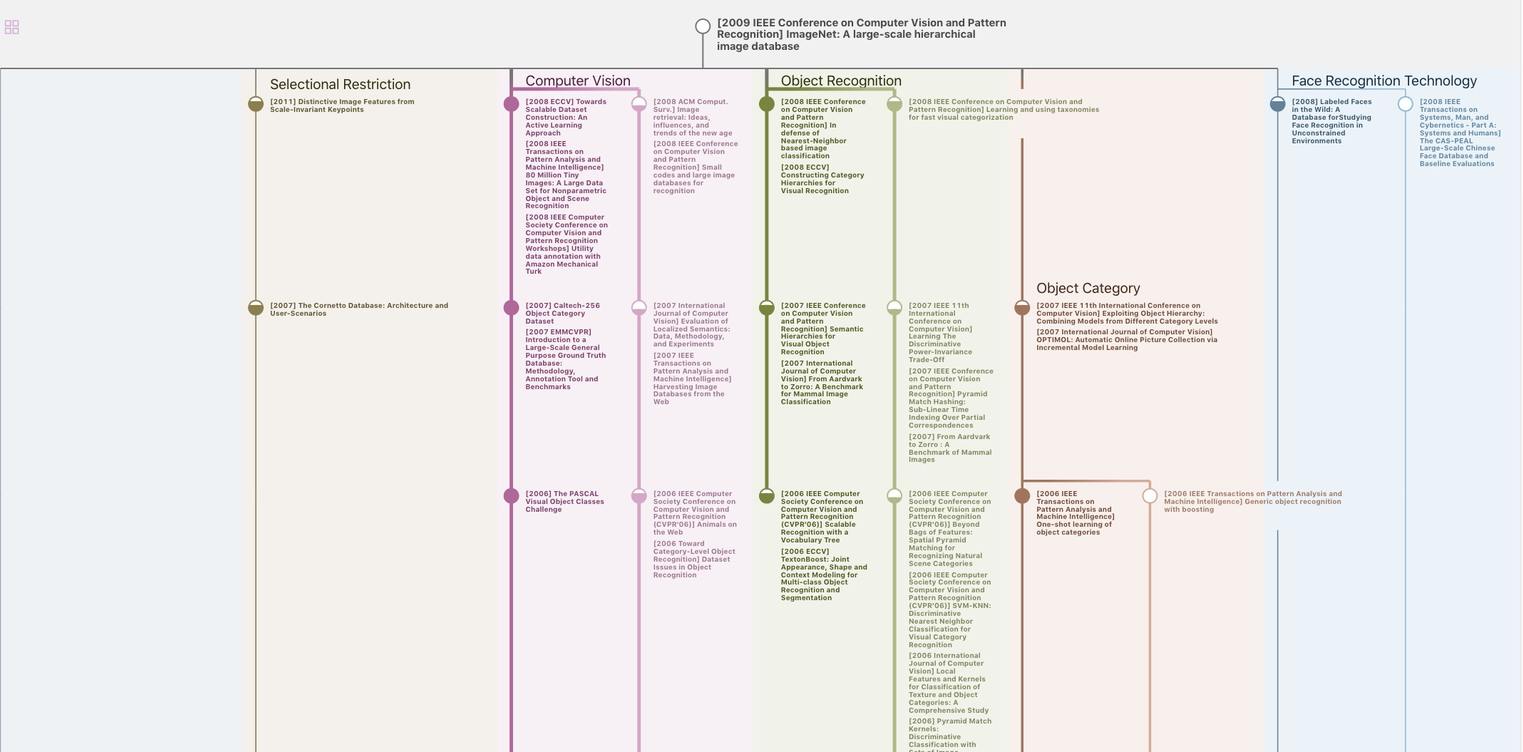
生成溯源树,研究论文发展脉络
Chat Paper
正在生成论文摘要