Variability Evaluation Of Cnns Using Cross-Validation On Viruses Images
VISAPP: PROCEEDINGS OF THE 15TH INTERNATIONAL JOINT CONFERENCE ON COMPUTER VISION, IMAGING AND COMPUTER GRAPHICS THEORY AND APPLICATIONS, VOL 4: VISAPP(2020)
摘要
Virus description and recognition is an essential issue in medicine. It helps researchers to study virus attributes such as its morphology, chemical compositions, and modes of replication. Although it can be performed through visual inspection, it is a task highly dependent on a qualified expert. Therefore, the automation of this task has received great attention over the past few years. In this study, we applied transfer learning from pre-trained deep neural networks for virus species classification. Given that many image datasets do not specify a fixed training and test sets, and to avoid any bias, we evaluated the impact of a cross-validation scheme on the classification accuracy. The experimental results achieved up to 89% of classification accuracy, outperforming previous studies by 2.8% of accuracy.
更多查看译文
关键词
Convolutional Neural Network, Cross-validation, Virus Classification
AI 理解论文
溯源树
样例
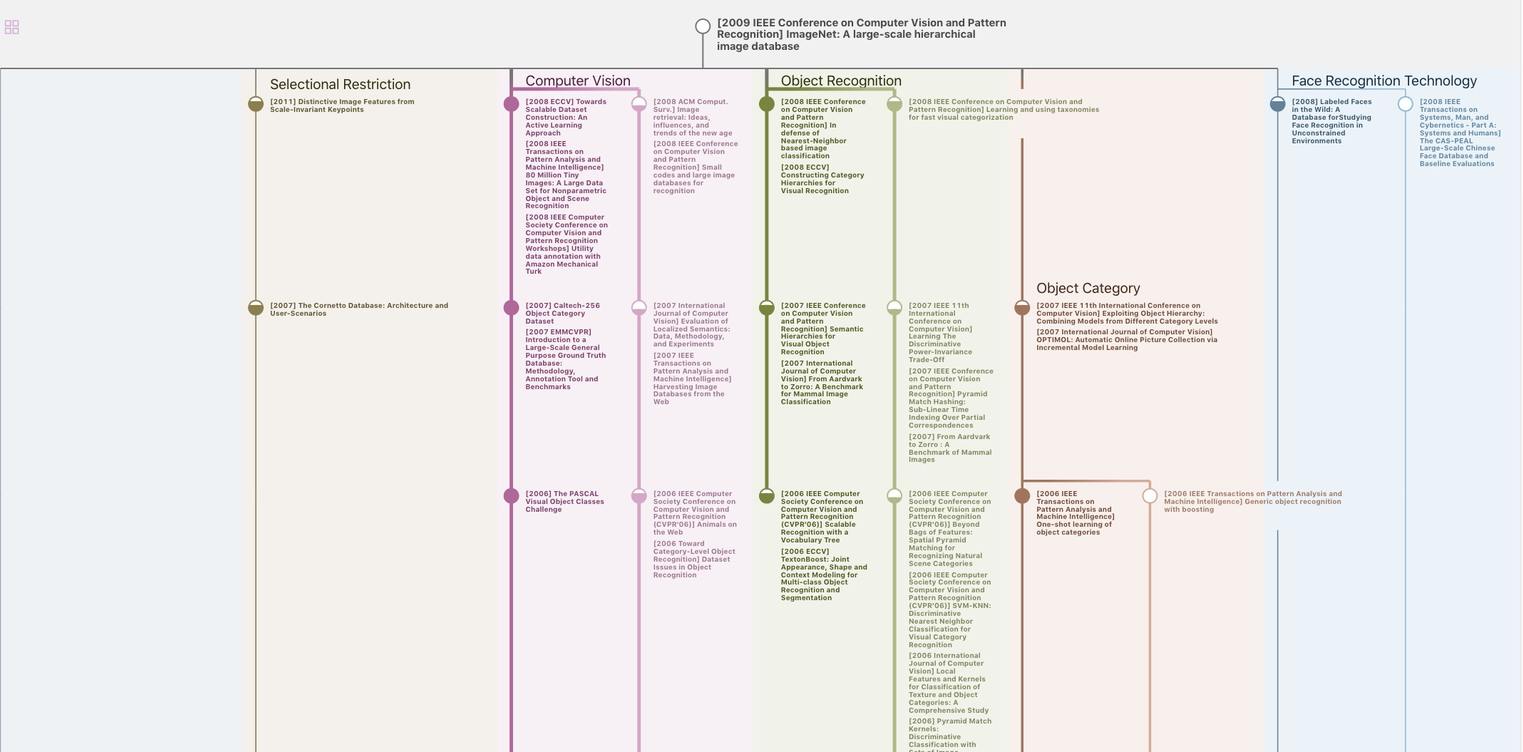
生成溯源树,研究论文发展脉络
Chat Paper
正在生成论文摘要