DFIOT: Data Fusion for Internet of Things
JOURNAL OF NETWORK AND SYSTEMS MANAGEMENT(2020)
摘要
In Internet of Things (IoT) ubiquitous environments, a high volume of heterogeneous data is produced from different devices in a quick span of time. In all IoT applications, the quality of information plays an important role in decision making. Data fusion is one of the current research trends in this arena that is considered in this paper. We particularly consider typical IoT scenarios where the sources measurements highly conflict, which makes intuitive fusions prone to wrong and misleading results. This paper proposes a taxonomy of decision fusion methods that rely on the theory of belief. It proposes a data fusion method for the Internet of Things (DFIOT) based on Dempster–Shafer (D–S) theory and an adaptive weighted fusion algorithm. It considers the reliability of each device in the network and the conflicts between devices when fusing data. This is while considering the information lifetime, the distance separating sensors and entities, and reducing computation. The proposed method uses a combination of rules based on the Basic Probability Assignment (BPA) to represent uncertain information or to quantify the similarity between two bodies of evidence. To investigate the effectiveness of the proposed method in comparison with D–S, Murphy, Deng and Yuan, a comprehensive analysis is provided using both benchmark data simulation and real dataset from a smart building testbed. Results show that DFIOT outperforms all the above mentioned methods in terms of reliability, accuracy and conflict management. The accuracy of the system reached up to 99.18% on benchmark artificial datasets and 98.87% on real datasets with a conflict of 0.58 % . We also examine the impact of this improvement from the application perspective (energy saving), and the results show a gain of up to 90% when using DFIOT.
更多查看译文
关键词
Wireless sensor networks, Dempster-Shafer theory, Conflict management, Energy consumption, Basic Probability Assignment, Similarity distance, Weighted evidences, Communication networks for data
AI 理解论文
溯源树
样例
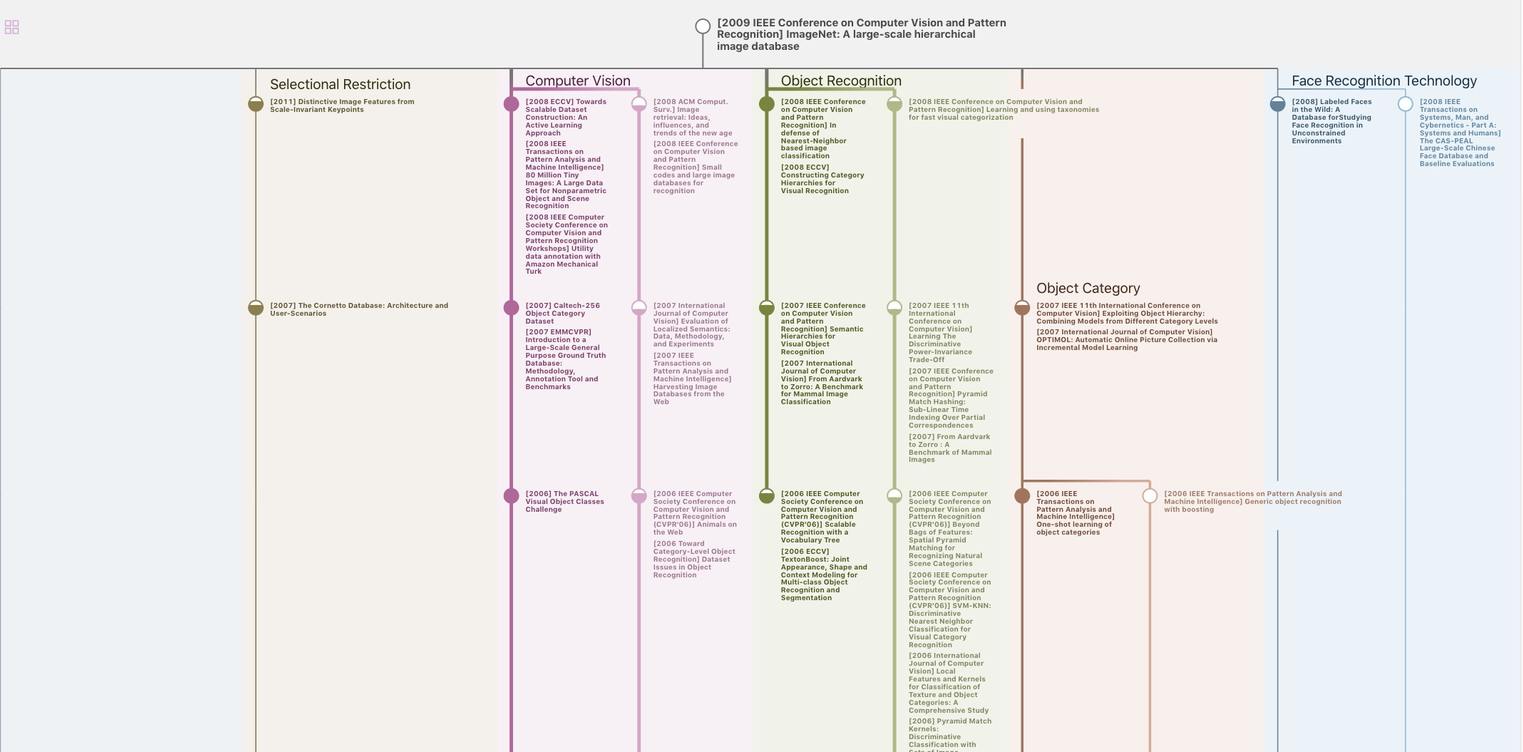
生成溯源树,研究论文发展脉络
Chat Paper
正在生成论文摘要