Recurrent Neural Networks-Based Collision-Free Motion Planning for Dual Manipulators Under Multiple Constraints
IEEE ACCESS(2020)
摘要
Dual robotic manipulators are robotic systems that are developed to imitate human arms, which shows great potential in performing complex tasks. Collision-free motion planning in real time is still a challenging problem for controlling a dual robotic manipulator because of the overlap workspace. In this paper, a novel planning strategy under physical constraints of dual manipulators using dynamic neural networks is proposed, which can satisfy the collision avoidance and trajectory tracking. Particularly, the problem of collision avoidance is first formulated into a set of inequality formulas, whereas the robotic trajectory is then transformed into an equality constraint by introducing negative feedback in outer loop. The planning problem subsequently becomes a Quadratic Programming (QP) problem by considering the redundancy, the boundaries of joint angles and velocities of the system. The QP is solved using a convergent provable recurrent neural network that without calculating the pseudo-inversion of the Jacobian. Consequently, numerical experiments on 8-DoF modular robot and 14-DoF Baxter robot are conducted to show the superiority of the proposed strategy.
更多查看译文
关键词
Motion planning,dual robotic manipulators,dynamic neural networks,zeroing neural networks,redundant resolution
AI 理解论文
溯源树
样例
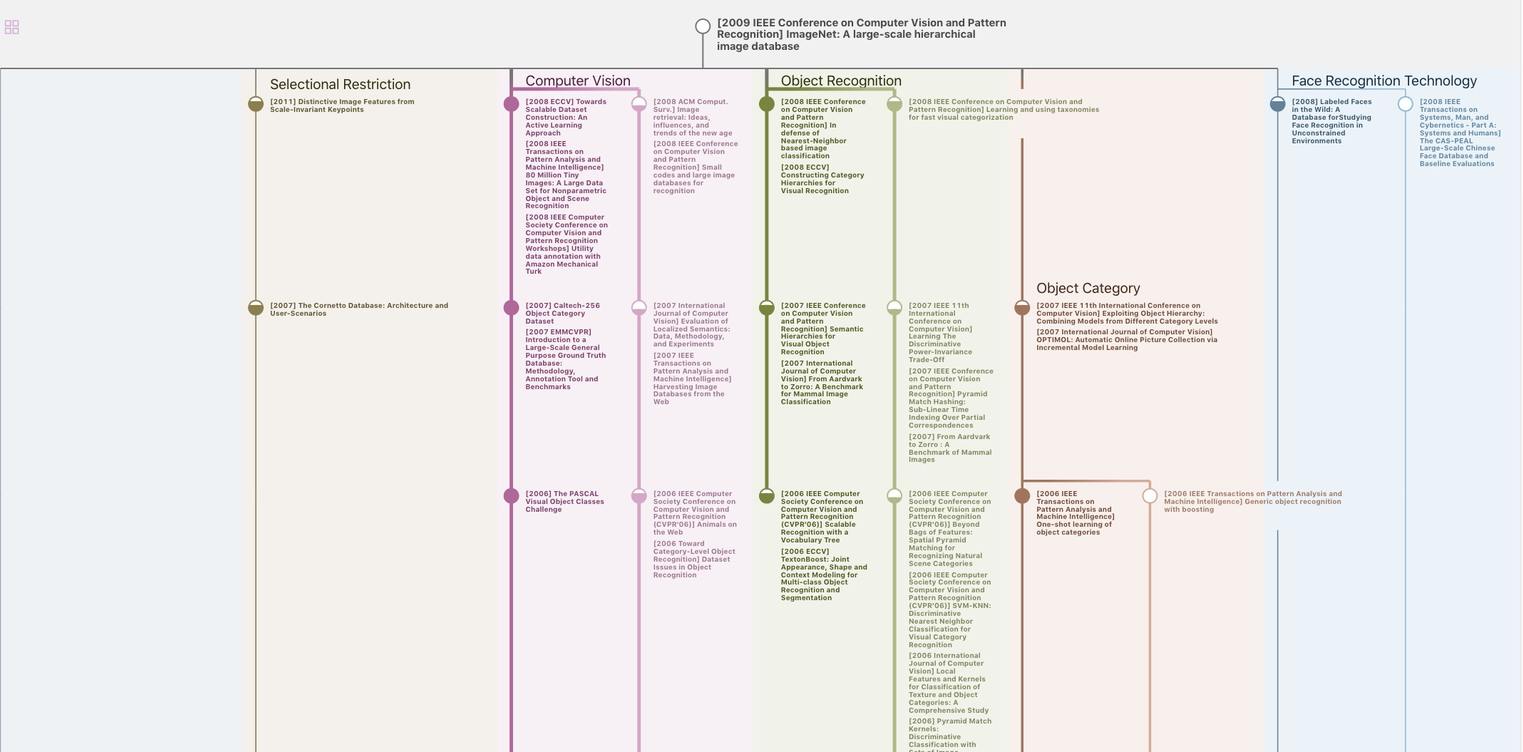
生成溯源树,研究论文发展脉络
Chat Paper
正在生成论文摘要