The curse of dimensionality (COD), misclassified DMUs, and Bayesian DEA
COMMUNICATIONS IN STATISTICS-SIMULATION AND COMPUTATION(2022)
摘要
Data envelopment analysis (DEA) is used to assess the relative efficiency of a set of decision-making units (DMUs). A potential drawback to DEA is that one must include a sufficient number of observations to ensure that all input-output dimensions are adequately characterized. Employing DEA with too few DMUs for a given set of inputs/outputs generates estimates that overstate efficiency. This is known as the "curse of dimensionality (COD)". Because production processes vary widely in technology and complexity, it is difficult to analytically characterize the effects of the COD on DEA-generated efficiency scores. This paper uses Bayesian methods to characterize and adjust for the possibility of misclassified DMUs and COD-related biases in DEA. Based on the nature of the COD bias we propose an appropriate prior distribution for the proportion of misclassified DMUs and use it to derive the concordant posterior distribution. A simulation analysis compares our model to those obtained with an ignorance prior distribution to evaluate the utility of the new model, and it is then applied to data from the Turkish electricity industry. We find that estimates of the probability of misclassification can be improved using our proposed prior distribution, especially in sample sizes of less than 40 observations.
更多查看译文
关键词
Bayesian priors, data envelopment analysis, incidence of inefficiency, simulation
AI 理解论文
溯源树
样例
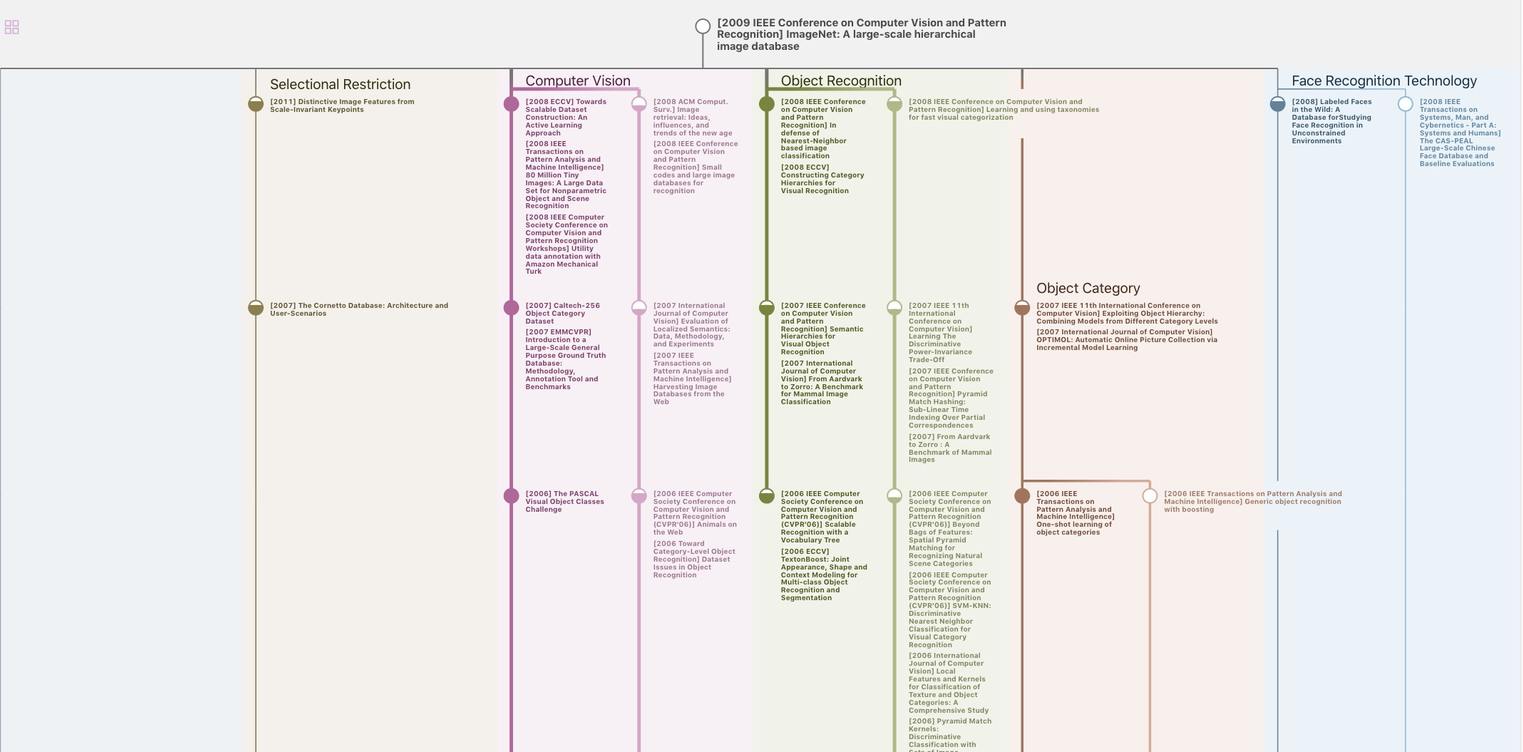
生成溯源树,研究论文发展脉络
Chat Paper
正在生成论文摘要