Computational Serendipitous Recommender System Frameworks: A Literature Survey
2019 IEEE/ACS 16TH INTERNATIONAL CONFERENCE ON COMPUTER SYSTEMS AND APPLICATIONS (AICCSA 2019)(2019)
摘要
Recommender systems help users find items that are relevant or interesting. These recommendations are based on the users profile, past behavior, and items similarity. Various recommender system algorithms presented in literature have achieved high accuracy in predicting relevant recommendations similar to the users profile. Even though with high accuracy, these results tend to be over-specified with obvious recommendations that are too similar to what the user already knows, failing to arouse users long-term interest. This survey paper presents a literature review on serendipity as one of the beyond accuracy measures to overcome the over-specialization problem. The survey starts with an introduction of the concept of serendipity, followed by several definitions of serendipity found in the literature. Then the main components of serendipity are presented novelty, surprise, and value. After that, the survey discusses several well-known serendipity recommender systems. While most surveys in serendipity categorize frameworks based on types of recommender systems, this survey categorizes serendipitous frameworks based on the conceptual view of serendipity. Finally, a discussion with various evaluation strategies on serendipity will be presented.
更多查看译文
关键词
Serendipity, Recommender System, Survey
AI 理解论文
溯源树
样例
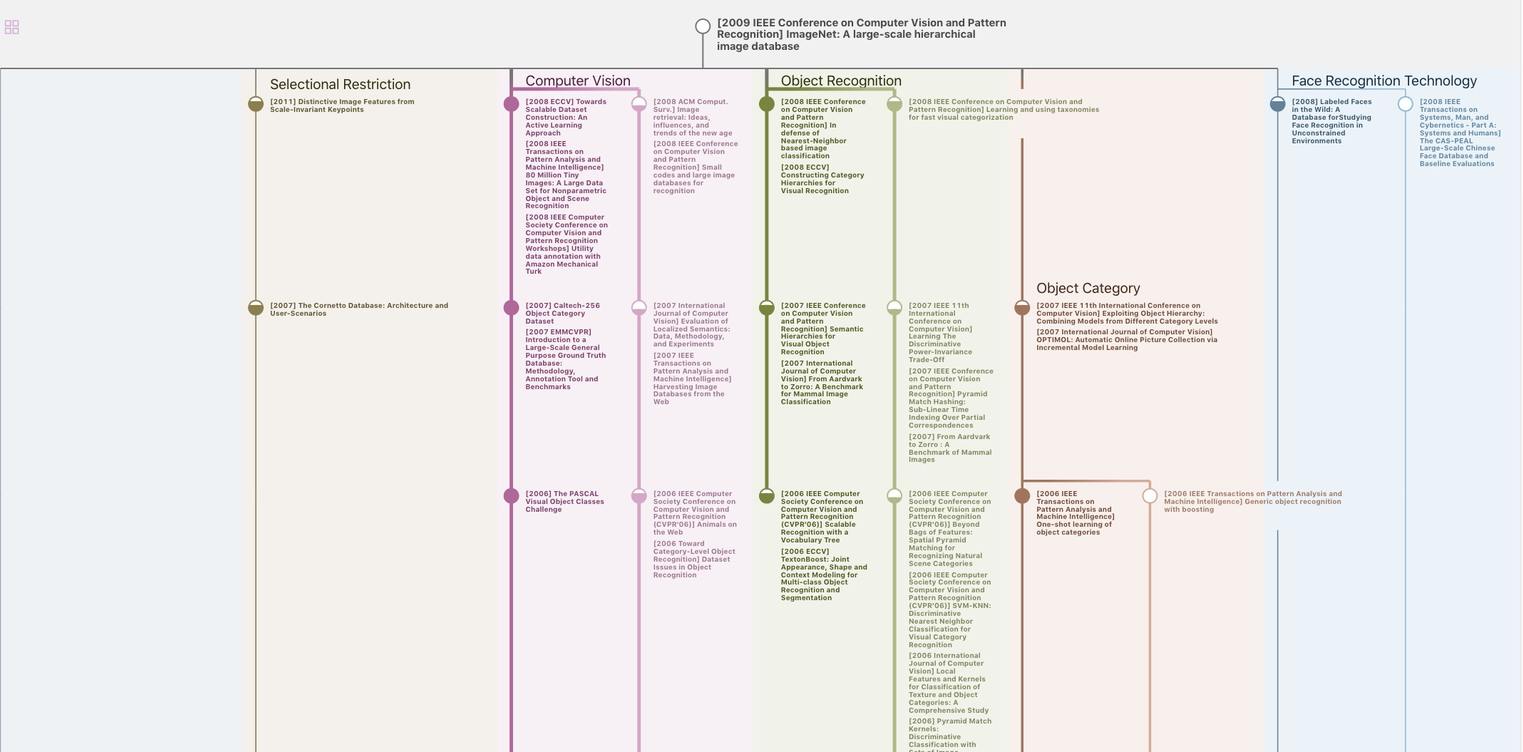
生成溯源树,研究论文发展脉络
Chat Paper
正在生成论文摘要