Using Machine Learning Methods to Support Causal Inference in Econometrics
Studies in computational intelligence(2020)
摘要
We provide an introduction to the use of machine learning methods in econometrics and how these methods can be employed to assist in causal inference. We begin with an extended presentation of the lasso (least absolute shrinkage and selection operator) of Tibshirani [50]. We then discuss the ‘Post-Double-Selection’ (PDS) estimator of Belloni et al. [13, 19] and show how it uses the lasso to address the omitted confounders problem. The PDS methodology is particularly powerful for the case where the researcher has a high-dimensional set of potential control variables, and needs to strike a balance between using enough controls to eliminate the omitted variable bias but not so many as to induce overfitting. The last part of the paper discusses recent developments in the field that go beyond the PDS approach.
更多查看译文
关键词
causal inference,econometrics,machine learning methods,machine learning
AI 理解论文
溯源树
样例
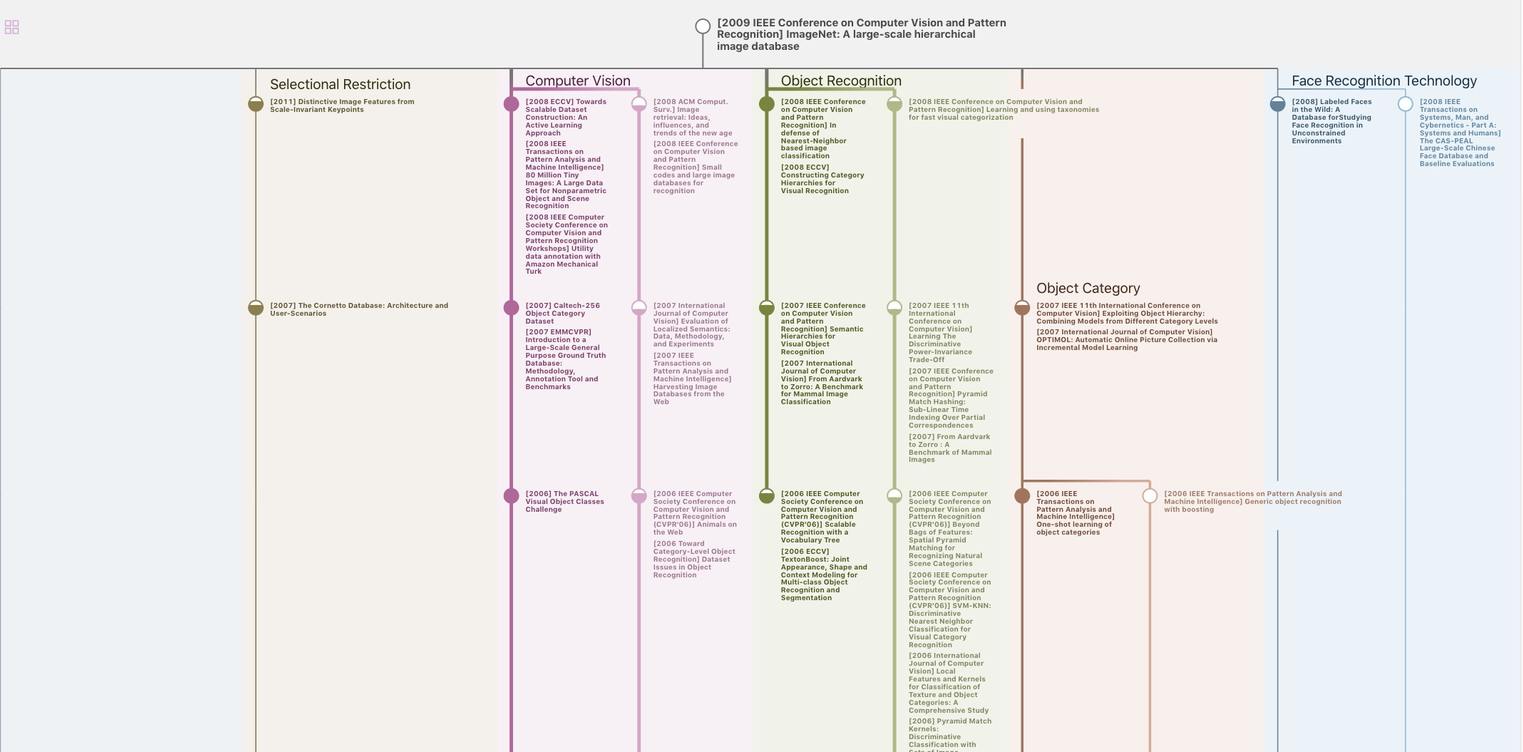
生成溯源树,研究论文发展脉络
Chat Paper
正在生成论文摘要