Improved Spatial Modulation Diversity with High Noise Robust Based on Deep Denoising Convolution Neural Network
Journal of Russian Laser Research(2020)
摘要
Synthetic aperture imaging systems can be applied to equivalently get high-resolution images of traditional monolithic primary mirror systems with less weight and costs. Spatial modulation diversity (SMD), a newly developed post-processing technology applicable for such synthetic aperture systems, is very sensitive to Gaussian noise, which greatly limits its further application. In this paper, we propose an improved SMD strategy by introducing the deep denoising convolutional neural networks (DnCNN) into the image preprocessing to improve the robustness of SMD. Results of the numerical simulations demonstrate that the strategy proposed exhibits superior performance compared to the traditional SMD technique in terms of both the root-mean-square error (RMSE) of phase estimates and the structural similarity (SSIM) of image reconstruction. In view of the superiority and robustness, the method we proposed may have important application prospects in multi-aperture imaging systems.
更多查看译文
关键词
spatial modulation diversity,deep denoising convolutional neural networks,phase estimate,image restoration
AI 理解论文
溯源树
样例
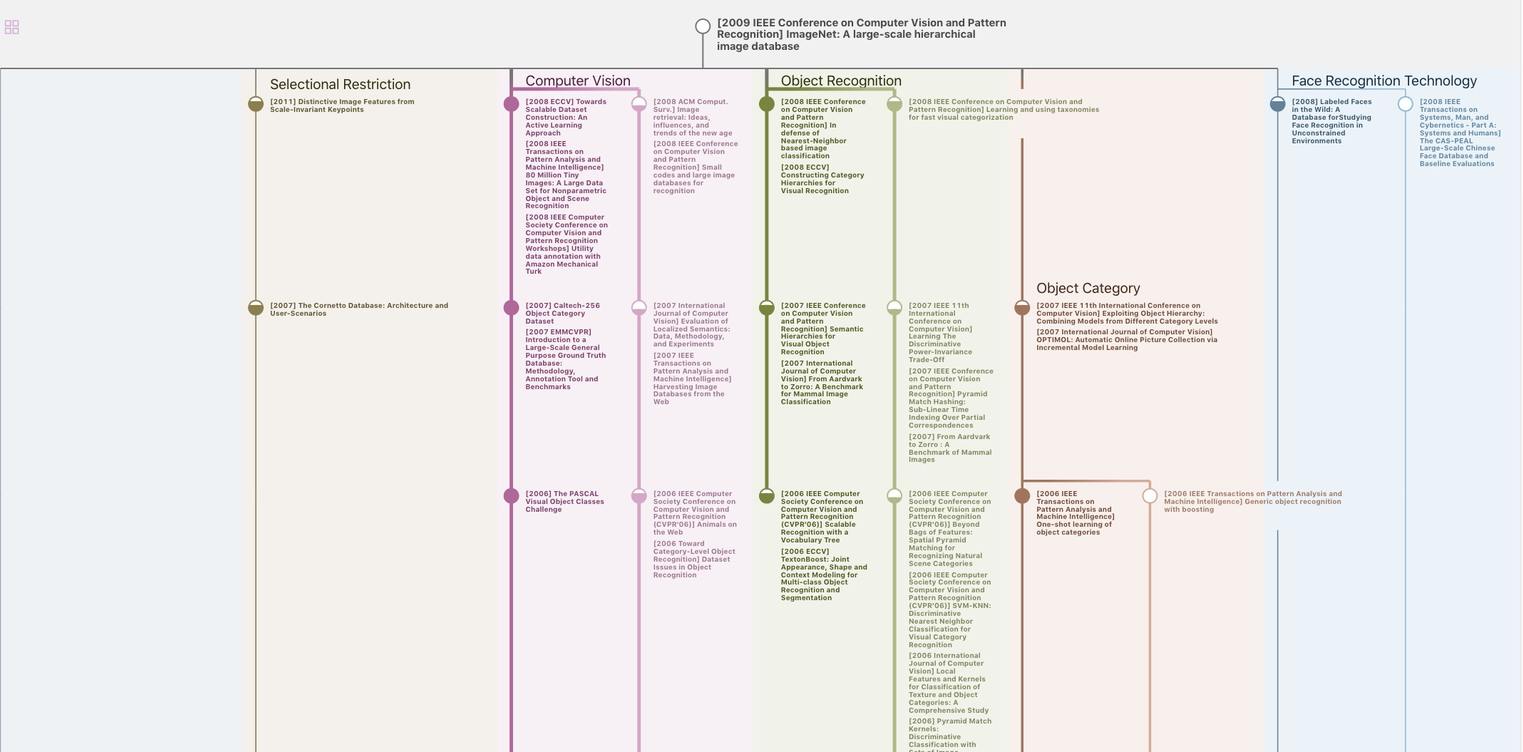
生成溯源树,研究论文发展脉络
Chat Paper
正在生成论文摘要