Riemannian Gradient-Based Online Identification Method For Linear Systems With Symmetric Positive-Definite Matrix
2019 IEEE 58TH CONFERENCE ON DECISION AND CONTROL (CDC)(2019)
摘要
This paper introduces a stochastic optimization-based approach to the online optimal identification of symmetric linear continuous-time systems. The identification problem is formulated as an optimization problem on a Riemannian manifold, which is a product manifold consisting of three manifolds, i.e., the manifold of symmetric positive definite matrices and two matrix spaces. We specifically address the case where the system matrices to be identified vary over time. We develop a novel algorithm for online identification, called the Riemannian online gradient descent method, in a manner similar to the Riemannian stochastic gradient descent method. Numerical experiments show that the proposed online algorithm considerably decreases the value of the objective function in a practical situation, where time intervals in which the system characteristic does not change significantly are assumed to be small.
更多查看译文
关键词
Riemannian gradient-based online identification method,linear systems,symmetric positive-definite matrix,stochastic optimization-based approach,online optimal identification,symmetric linear continuous-time systems,identification problem,optimization problem,Riemannian manifold,product manifold,symmetric positive definite matrices,matrix spaces,system matrices,Riemannian online gradient descent method,Riemannian stochastic gradient descent method,online algorithm,time intervals,system characteristic
AI 理解论文
溯源树
样例
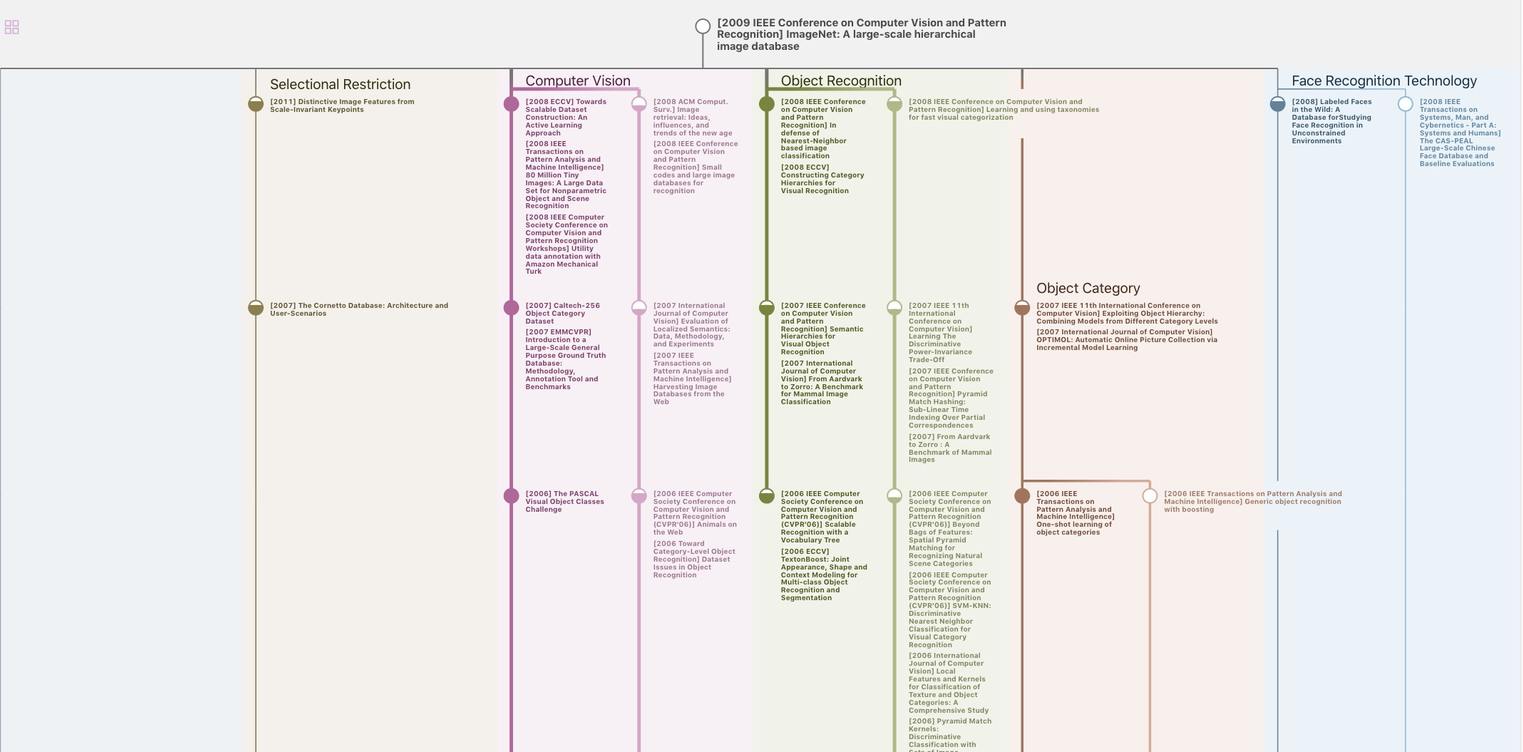
生成溯源树,研究论文发展脉络
Chat Paper
正在生成论文摘要