Inference Of Suspicious Co-Visitation And Co-Rating Behaviors And Abnormality Forensics For Recommender Systems
IEEE TRANSACTIONS ON INFORMATION FORENSICS AND SECURITY(2020)
摘要
The pervasiveness of personalized collaborative recommender systems has shown the powerful capability in a wide range of E-commerce services such as Amazon, TripAdvisor, Yelp, etc. However, fundamental vulnerabilities of collaborative recommender systems leave space for malicious users to affect the recommendation results as the attackers desire. A vast majority of existing detection methods assume certain properties of malicious attacks are given in advance. In reality, improving the detection performance is usually constrained due to the challenging issues: (a) various types of malicious attacks coexist, (b) limited representations of malicious attack behaviors, and (c) practical evidences for exploring and spotting anomalies on real-world data are scarce. In this paper, we investigate a unified detection framework in an eye for an eye manner without being bothered by the details of the attacks. Firstly, co-visitation and co-rating graphs are constructed using association rules. Then, attribute representations of nodes are empirically developed from the perspectives of linkage pattern, structure-based property and inherent association of nodes. Finally, both attribute information and connective coherence of graph are combined in order to infer suspicious nodes. Extensive experiments on both synthetic and real-world data demonstrate the effectiveness of the proposed detection approach compared with competing benchmarks. Additionally, abnormality forensics metrics including distribution of rating intention, time aggregation of suspicious ratings, degree distributions before as well as after removing suspicious nodes and time series analysis of historical ratings, are provided so as to discover interesting findings such as suspicious nodes (items or ratings) on real-world data.
更多查看译文
关键词
Recommender systems, Forensics, Anomaly detection, Time series analysis, Collaboration, Couplings, Measurement, Abnormality forensics, malicious attack, attack detection, recommender system
AI 理解论文
溯源树
样例
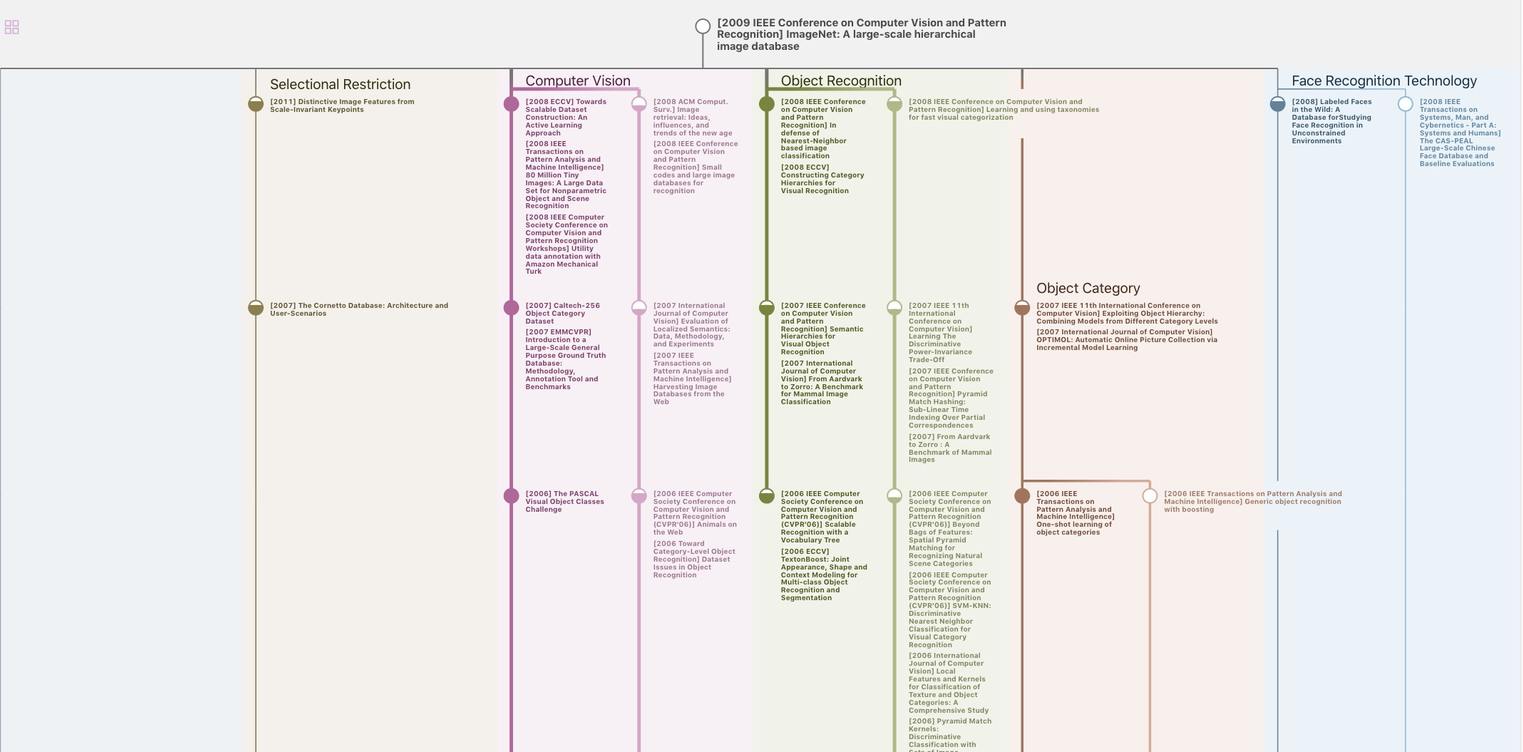
生成溯源树,研究论文发展脉络
Chat Paper
正在生成论文摘要