Online writer identification system using adaptive sparse representation framework
IET Biometrics(2020)
摘要
This study explores an adaptive sparse representation approach for online writer identification. The main focus is on employing prior information that quantifies the degree of importance of a dictionary atom concerning a given writer. This information is proposed by a fusion of two derived components. The first component is a saliency measure obtained from the sum-pooled sparse coefficients corresponding to the sub-strokes of a set of enrolled writers. The second component is a similarity score, computed for each dictionary atom with regards to a given writer, that is related to the reconstruction error of the sub-stroke based feature vectors. The proposed identification is accomplished with an ensemble of support vector machines (SVMs), wherein the input to the SVM trained for a writer is obtained by incorporating the adapted saliency values of that writer on the document descriptor obtained via average pooling of sparse codes. Experiments performed on the IAM and IBM-UB1 online handwriting databases demonstrate the efficacy of the proposed scheme.
更多查看译文
关键词
feature extraction,handwritten character recognition,learning (artificial intelligence),image classification,handwriting recognition,support vector machines,image representation
AI 理解论文
溯源树
样例
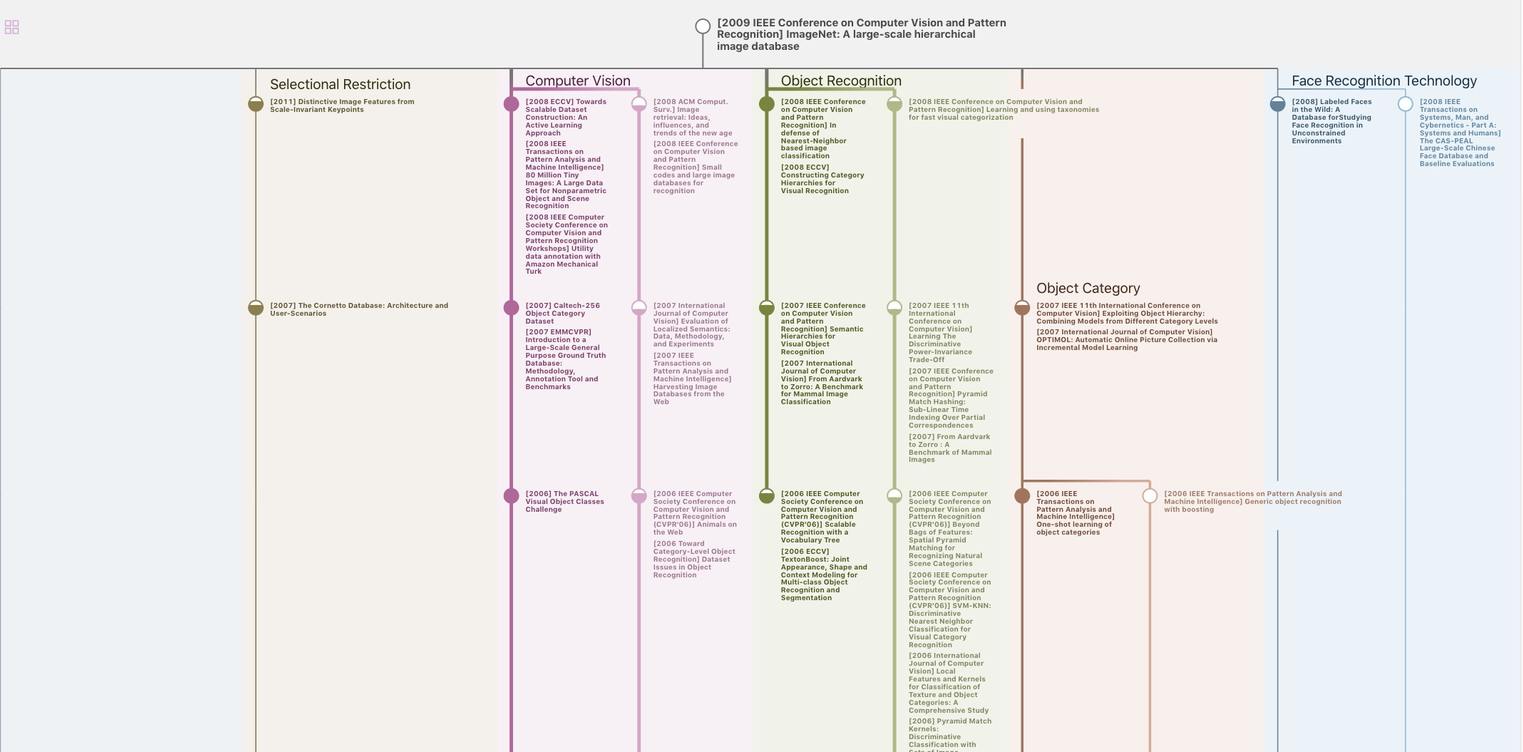
生成溯源树,研究论文发展脉络
Chat Paper
正在生成论文摘要