Multiscale Supervised Kernel Dictionary Learning for SAR Target Recognition
IEEE Transactions on Geoscience and Remote Sensing(2020)
摘要
In this article, a supervised nonlinear dictionary learning (DL) method, called multiscale supervised kernel DL (MSK-DL), is proposed for target recognition in synthetic aperture radar (SAR) images. We use Frost filters with different parameters to extract an SAR image's multiscale features for data augmentation and noise suppression. In order to reduce the computation cost, the dimension of each scale feature is reduced by principal component analysis (PCA). Instead of the widely used linear DL, we learn multiple nonlinear dictionaries to capture the nonlinear structure of data by introducing the dimension-reduced features into the nonlinear reconstruction error terms. A classification model, which is defined as a discriminative classification error term, is learned simultaneously. Hence, the objective function contains the nonlinear reconstruction error terms and a classification error term. Two optimization algorithms, called multiscale supervised kernel K-singular value decomposition (MSK-KSVD) and multiscale supervised incremental kernel DL (MSIK-DL), are proposed to compute the multidictionary and the classifier. Experiments on the moving and stationary target automatic recognition (MSTAR) data set are performed to evaluate the effectiveness of the two proposed algorithms. And the experimental results demonstrate that the proposed scheme outperforms some representative common machine learning strategies, state-of-the-art convolutional neural network (CNN) models and some representative DL methods, especially in terms of its robustness against training set size and noise.
更多查看译文
关键词
Kernel dictionary learning (DL),multiscale supervised incremental kernel DL (MSIK-DL),multiscale supervised kernel K-singular value decomposition (MSK-KSVD),multiscale features,synthetic aperture radar (SAR) target recognition
AI 理解论文
溯源树
样例
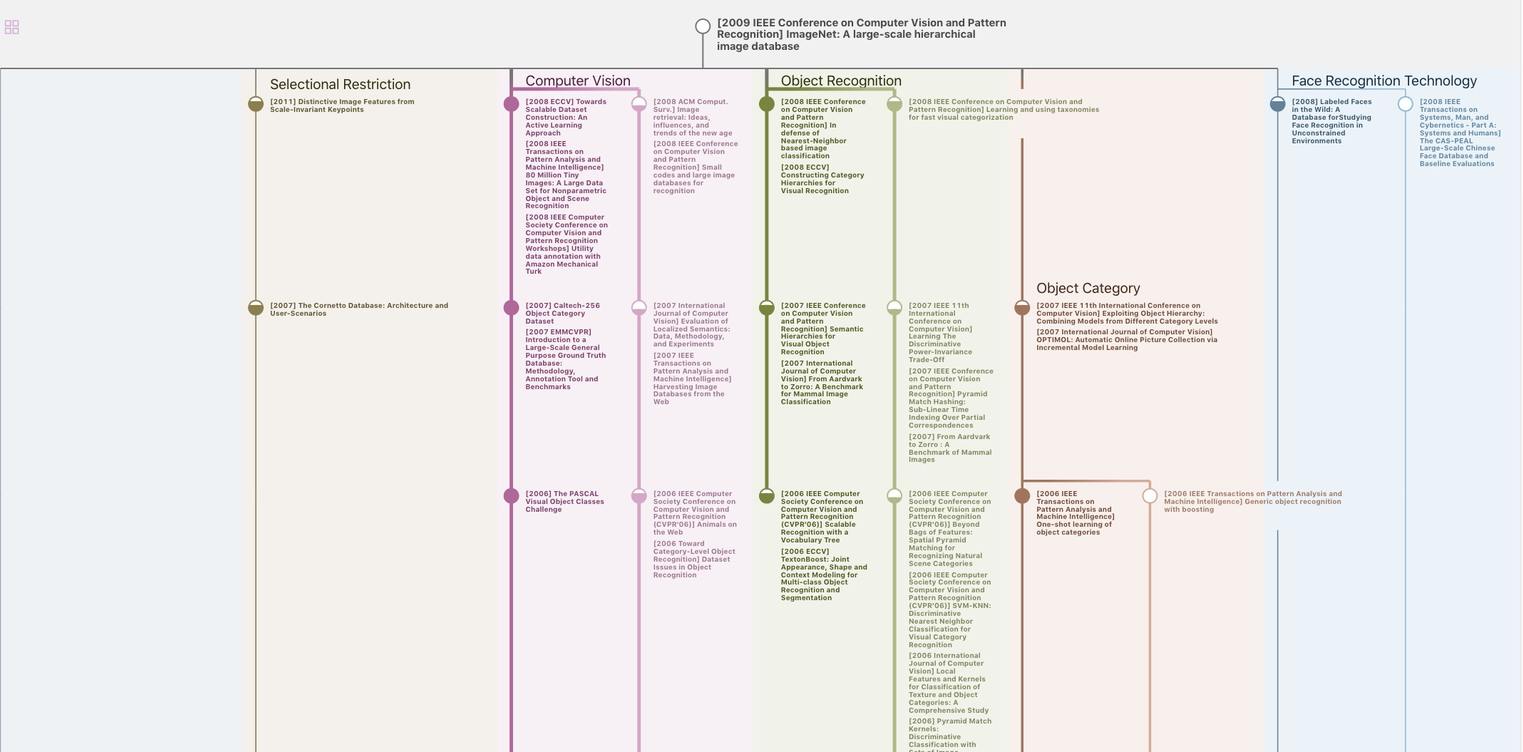
生成溯源树,研究论文发展脉络
Chat Paper
正在生成论文摘要