Analyzing and Detecting Adversarial Spam on a Large-scale Online APP Review System
WWW '20: The Web Conference 2020 Taipei Taiwan April, 2020(2020)
摘要
The online review system provides a platform for consumers to express their opinions and make decisions. However, the spam review has become a widespread problem recently. Existing detection systems are mostly designed for fraudsters who write fake reviews to promote their products or mislead consumers in E-commerce (e.g., Amazon, Yelp, Alibaba). In this paper, we focus on spams in the large-scale online app review platform. Through an in-depth analysis of 5 million reviews from the Tencent App store, we find almost half of the reviews are spams. Even worse, most of the reviews are deliberately designed by fraud reviewers for misleading classifiers. Specially, we conclude three popular patterns of generating adversarial spams by attackers.
Considering the characteristics of spam reviews are adversarial, irregular, and distributed. Human labeling is costly. We build our detection system by utilizing a collective method to spot suspicious users and reviews. First, we trained our two models based on the Behavior-Based Module (BBM) and Content-Based Module (CBM) individually. Then, they are co-trained by getting the feedback from each other. Experiment results show that our method outperforms the state-of-the-art baselines in identifying adversarial examples. Besides, the scalability and effectiveness of our approach have also been demonstrated by deploying it on Tencent Beacon Anti-Fraud System.
更多查看译文
关键词
Adversarial Detection, Online APP Review System
AI 理解论文
溯源树
样例
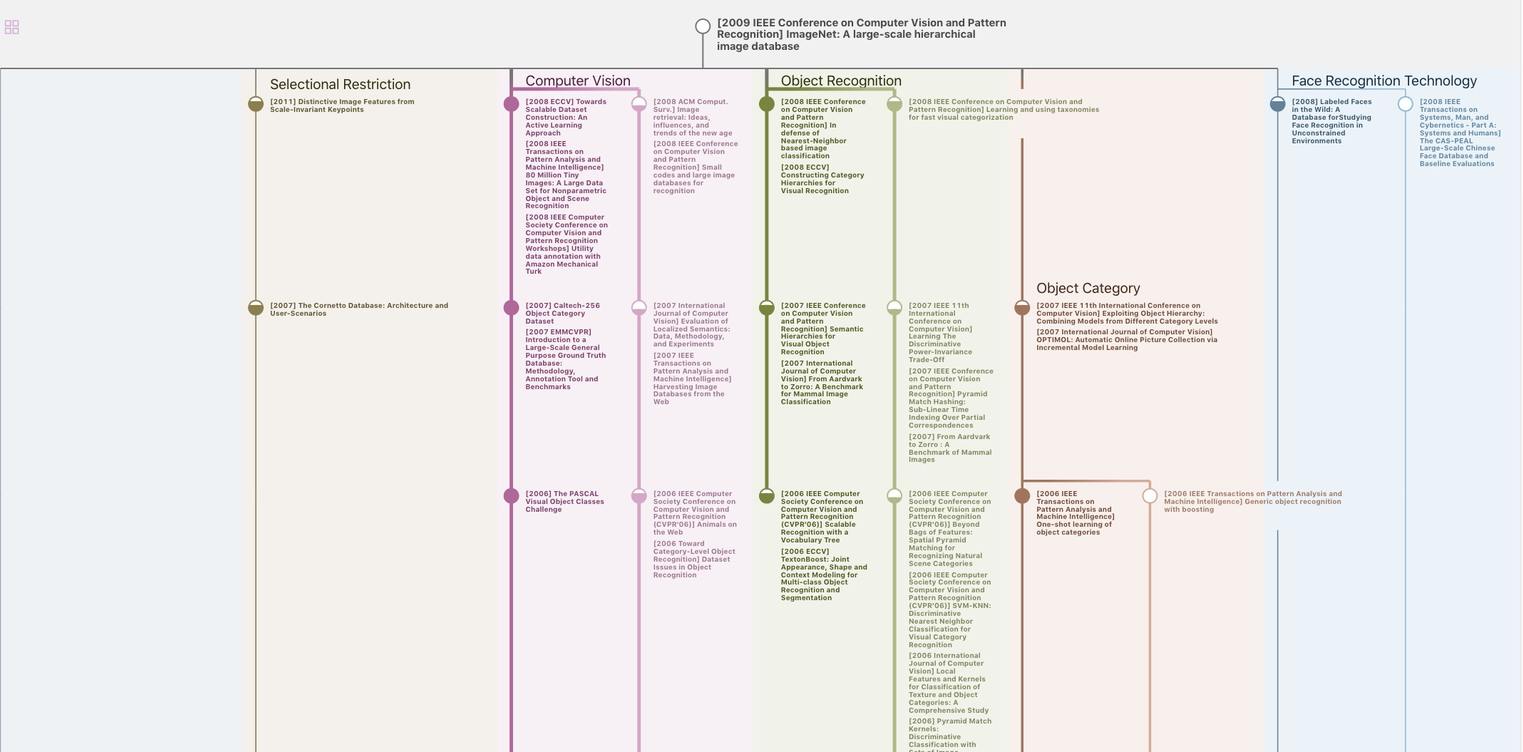
生成溯源树,研究论文发展脉络
Chat Paper
正在生成论文摘要