HTML: Hierarchical Transformer-based Multi-task Learning for Volatility Prediction
WWW '20: The Web Conference 2020 Taipei Taiwan April, 2020(2020)
摘要
The volatility forecasting task refers to predicting the amount of variability in the price of a financial asset over a certain period. It is an important mechanism for evaluating the risk associated with an asset and, as such, is of significant theoretical and practical importance in financial analysis. While classical approaches have framed this task as a time-series prediction one – using historical pricing as a guide to future risk forecasting – recent advances in natural language processing have seen researchers turn to complementary sources of data, such as analyst reports, social media, and even the audio data from earnings calls. This paper proposes a novel hierarchical, transformer, multi-task architecture designed to harness the text and audio data from quarterly earnings conference calls to predict future price volatility in the short and long term. This includes a comprehensive comparison to a variety of baselines, which demonstrates very significant improvements in prediction accuracy, in the range 17% - 49% compared to the current state-of-the-art. In addition, we describe the results of an ablation study to evaluate the relative contributions of each component of our approach and the relative contributions of text and audio data with respect to prediction accuracy.
更多查看译文
关键词
Volatility forecasting, Hierarchical transformer, Multi-task learning
AI 理解论文
溯源树
样例
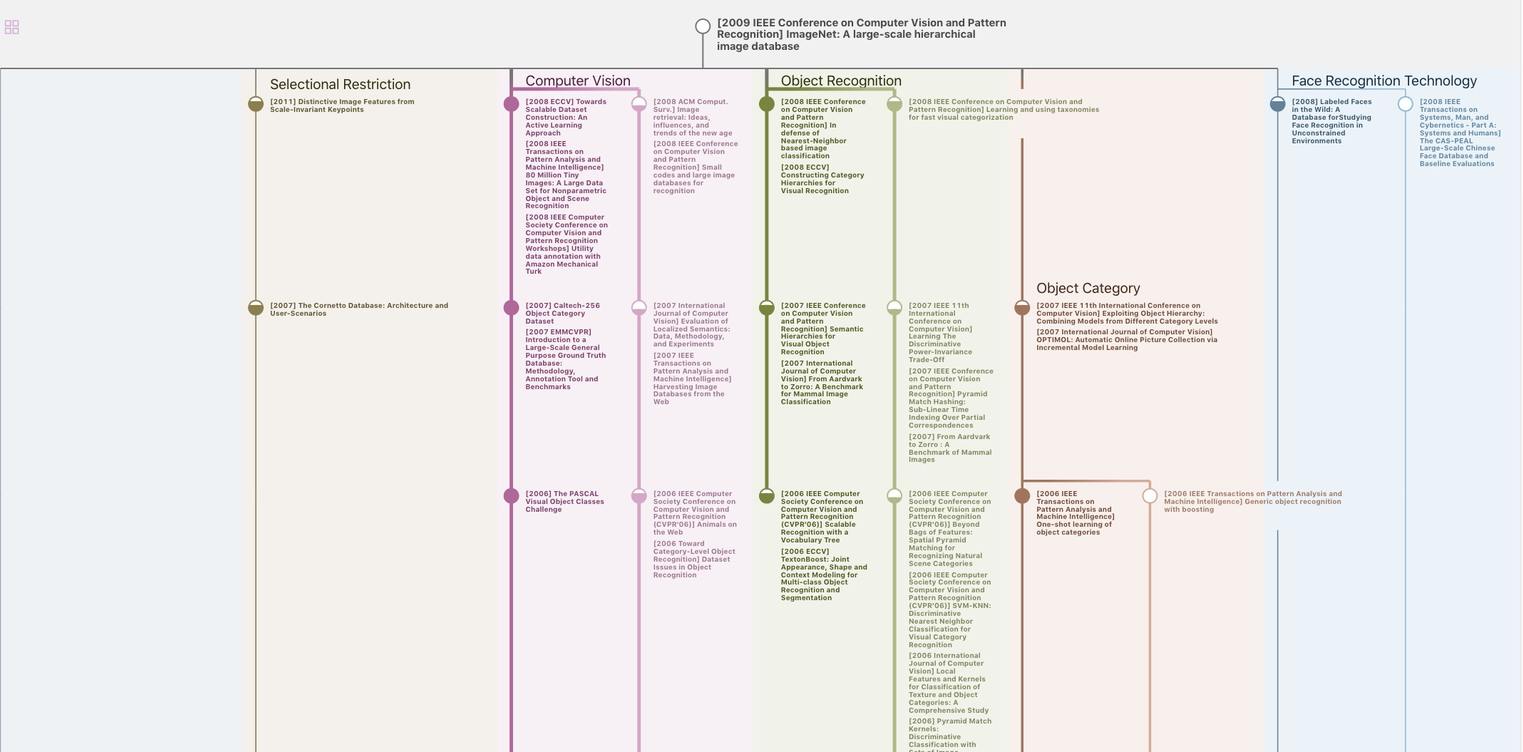
生成溯源树,研究论文发展脉络
Chat Paper
正在生成论文摘要