Learning Model-Agnostic Counterfactual Explanations for Tabular Data
WWW '20: The Web Conference 2020 Taipei Taiwan April, 2020(2020)
摘要
Counterfactual explanations can be obtained by identifying the smallest change made to an input vector to influence a prediction in a positive way from a user’s viewpoint; for example, from ’loan rejected’ to ’awarded’ or from ’high risk of cardiovascular disease’ to ’low risk’. Previous approaches would not ensure that the produced counterfactuals be proximate (i.e., not local outliers) and connected to regions with substantial data density (i.e., close to correctly classified observations), two requirements known as counterfactual faithfulness. Our contribution is twofold. First, drawing ideas from the manifold learning literature, we develop a framework, called C-CHVAE, that generates faithful counterfactuals. Second, we suggest to complement the catalog of counterfactual quality measures using a criterion to quantify the degree of difficulty for a certain counterfactual suggestion. Our real world experiments suggest that faithful counterfactuals come at the cost of higher degrees of difficulty.
更多查看译文
关键词
Transparency, Counterfactual explanations, Interpretability
AI 理解论文
溯源树
样例
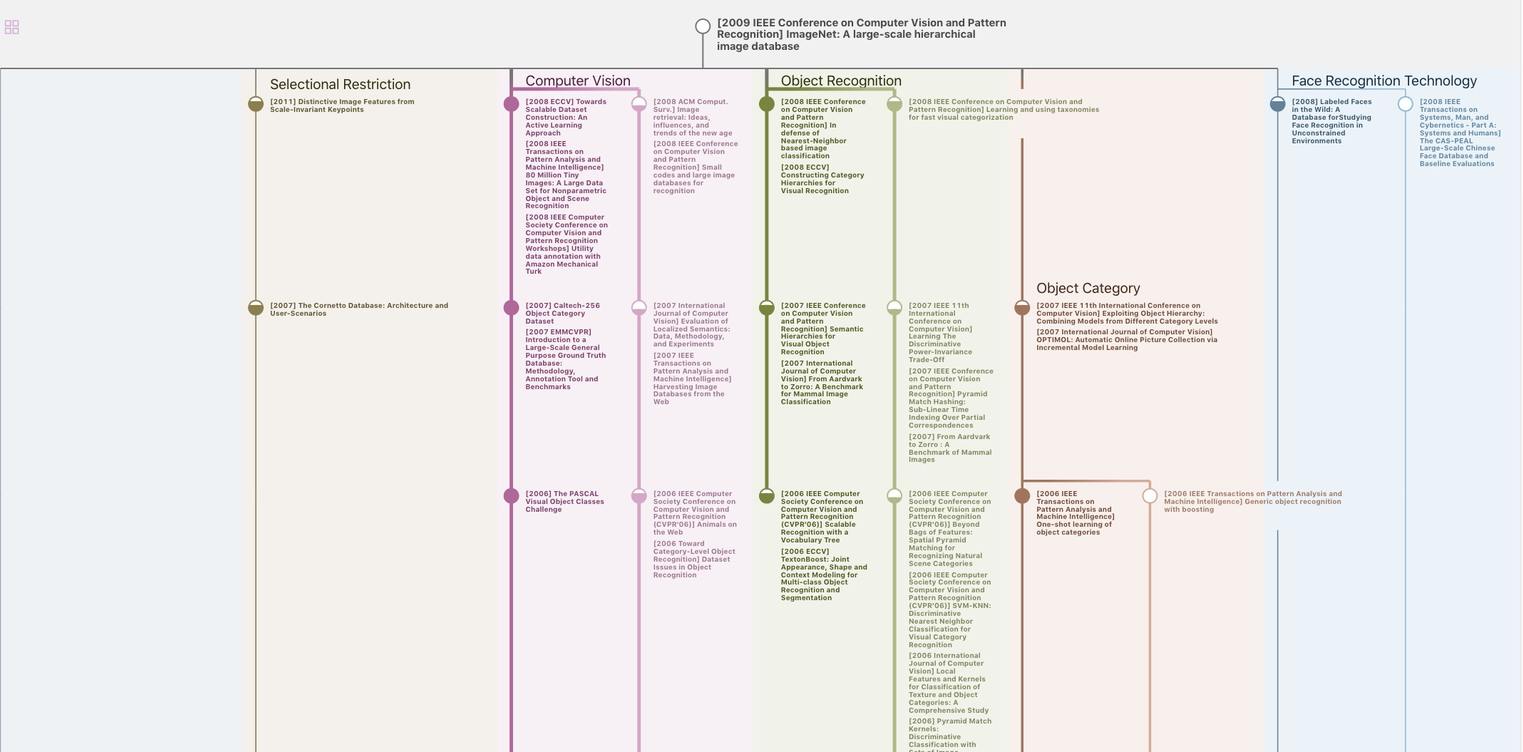
生成溯源树,研究论文发展脉络
Chat Paper
正在生成论文摘要