Privacy-preserving AI Services Through Data Decentralization
WWW '20: The Web Conference 2020 Taipei Taiwan April, 2020(2020)
摘要
User services increasingly base their actions on AI models, e.g., to offer personalized and proactive support. However, the underlying AI algorithms require a continuous stream of personal data—leading to privacy issues, as users typically have to share this data out of their territory. Current privacy-preserving concepts are either not applicable to such AI-based services or to the disadvantage of any party. This paper presents PrivAI, a new decentralized and privacy-by-design platform for overcoming the need for sharing user data to benefit from personalized AI services. In short, PrivAI complements existing approaches to personal data stores, but strictly enforces the confinement of raw user data. PrivAI further addresses the resulting challenges by (1) dividing AI algorithms into cloud-based general model training, subsequent local personalization, and community-based sharing of model updates for new users; by (2) loading confidential AI models into a trusted execution environment, and thus, protecting provider’s intellectual property (IP). Our experiments show the feasibility and effectiveness of PrivAI with comparable performance as currently-practiced approaches.
更多查看译文
关键词
AI services, ad-hoc personalization, PDS, IP/data protection
AI 理解论文
溯源树
样例
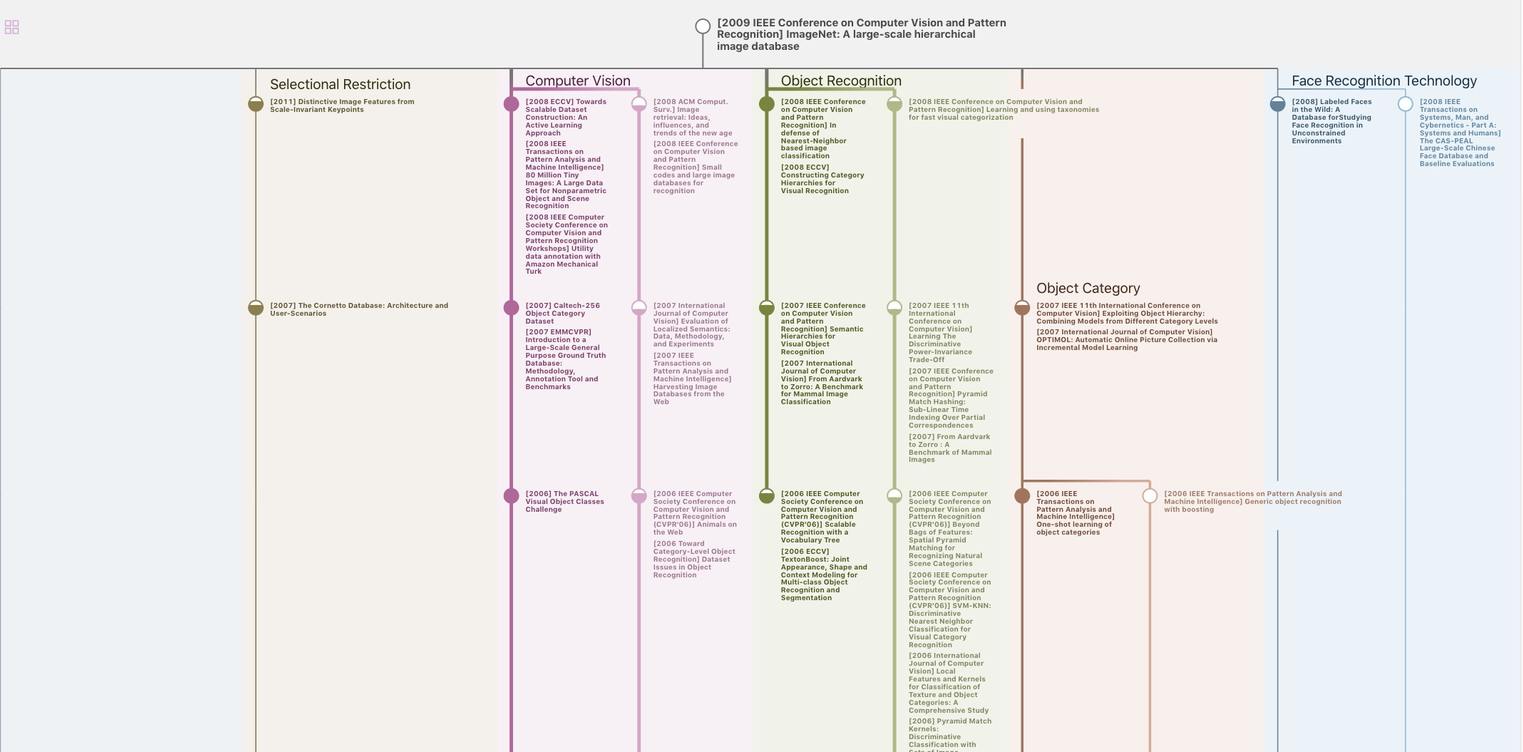
生成溯源树,研究论文发展脉络
Chat Paper
正在生成论文摘要