paper2repo: GitHub Repository Recommendation for Academic Papers
WWW '20: The Web Conference 2020 Taipei Taiwan April, 2020(2020)
摘要
GitHub has become a popular social application platform, where a large number of users post their open source projects. In particular, an increasing number of researchers release repositories of source code related to their research papers in order to attract more people to follow their work. Motivated by this trend, we describe a novel item-item cross-platform recommender system, paper2repo, that recommends relevant repositories on GitHub that match a given paper in an academic search system such as Microsoft Academic. The key challenge is to identify the similarity between an input paper and its related repositories across the two platforms, without the benefit of human labeling. Towards that end, paper2repo integrates text encoding and constrained graph convolutional networks (GCN) to automatically learn and map the embeddings of papers and repositories into the same space, where proximity offers the basis for recommendation. To make our method more practical in real life systems, labels used for model training are computed automatically from features of user actions on GitHub. In machine learning, such automatic labeling is often called distant supervision. To the authors’ knowledge, this is the first distant-supervised cross-platform (paper to repository) matching system. We evaluate the performance of paper2repo on real-world data sets collected from GitHub and Microsoft Academic. Results demonstrate that it outperforms other state of the art recommendation methods.
更多查看译文
关键词
Recommender system, cross-platform recommendation, constrained graph convolutional networks, text encoding
AI 理解论文
溯源树
样例
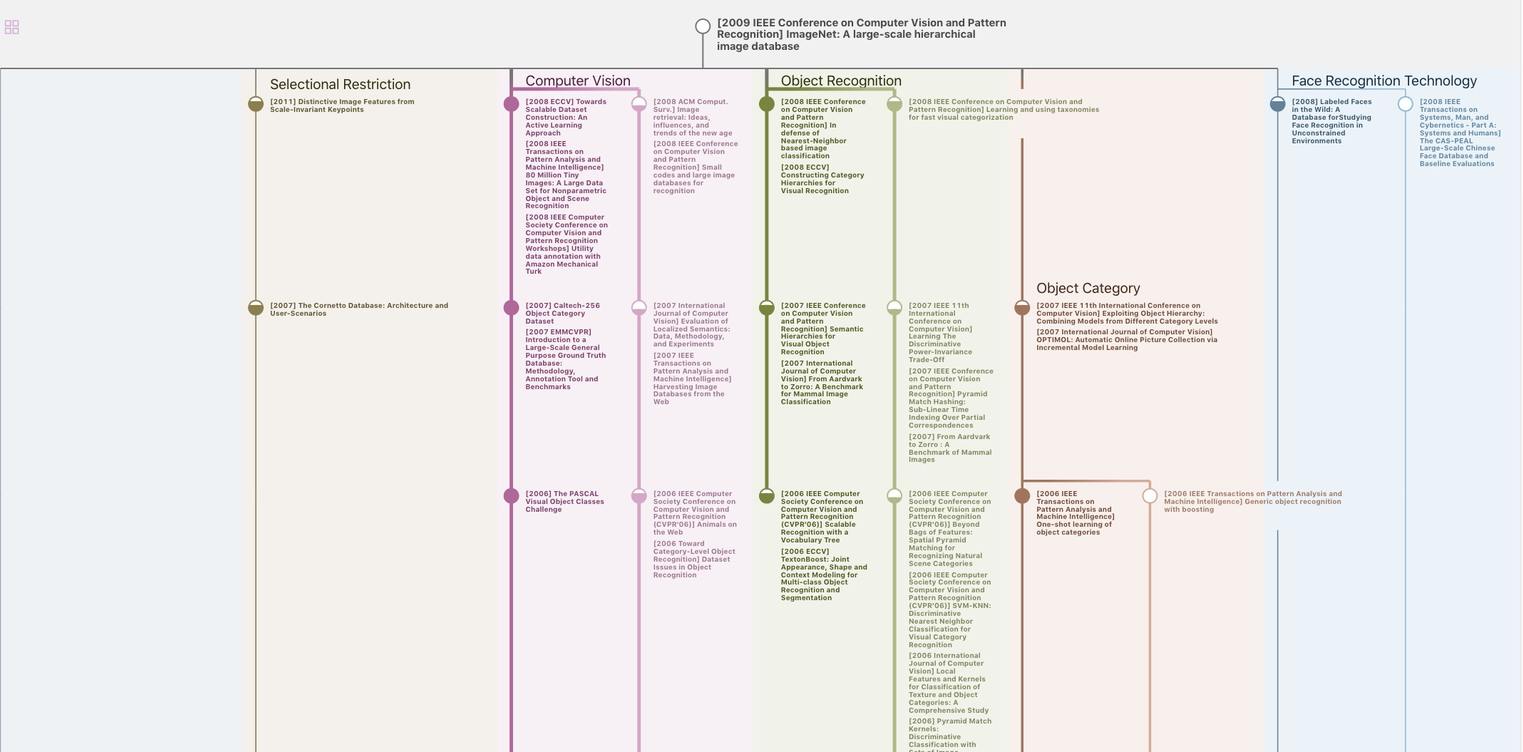
生成溯源树,研究论文发展脉络
Chat Paper
正在生成论文摘要