Domain Adaptation with Category Attention Network for Deep Sentiment Analysis
WWW '20: The Web Conference 2020 Taipei Taiwan April, 2020(2020)
摘要
Domain adaptation tasks such as cross-domain sentiment classification aim to utilize existing labeled data in the source domain and unlabeled or few labeled data in the target domain to improve the performance in the target domain via reducing the shift between the data distributions. Existing cross-domain sentiment classification methods need to distinguish pivots, i.e., the domain-shared sentiment words, and non-pivots, i.e., the domain-specific sentiment words, for excellent adaptation performance. In this paper, we first design a Category Attention Network (CAN), and then propose a model named CAN-CNN to integrate CAN and a Convolutional Neural Network (CNN). On the one hand, the model regards pivots and non-pivots as unified category attribute words and can automatically capture them to improve the domain adaptation performance; on the other hand, the model makes an attempt at interpretability to learn the transferred category attribute words. Specifically, the optimization objective of our model has three different components: 1) the supervised classification loss; 2) the distributions loss of category feature weights; 3) the domain invariance loss. Finally, the proposed model is evaluated on three public sentiment analysis datasets and the results demonstrate that CAN-CNN can outperform other various baseline methods.
更多查看译文
关键词
Domain Adaptation, Category Attention Network, Sentiment Analysis, Interpretability
AI 理解论文
溯源树
样例
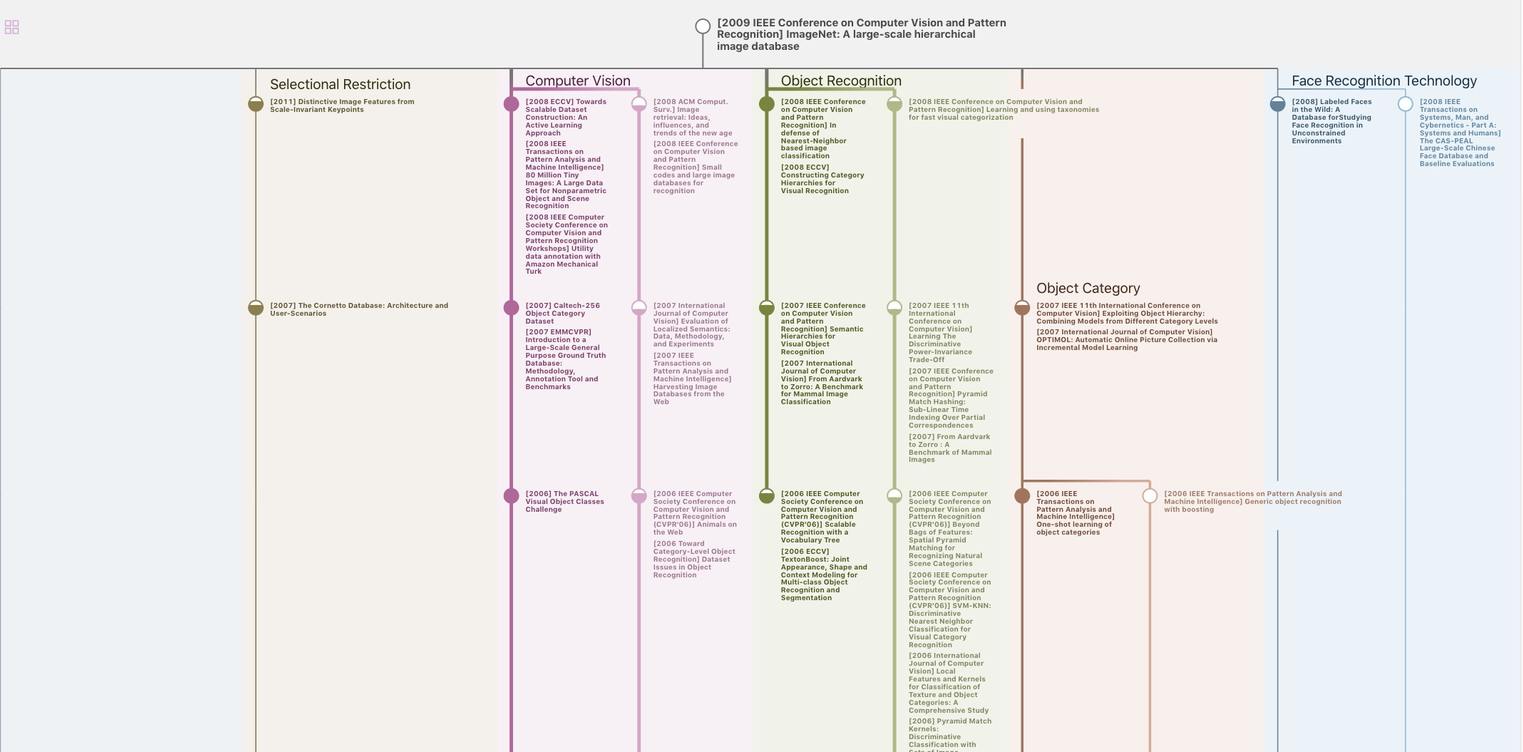
生成溯源树,研究论文发展脉络
Chat Paper
正在生成论文摘要