Algorithmic Effects on the Diversity of Consumption on Spotify
WWW '20: The Web Conference 2020 Taipei Taiwan April, 2020(2020)
摘要
On many online platforms, users can engage with millions of pieces of content, which they discover either organically or through algorithmically-generated recommendations. While the short-term benefits of recommender systems are well-known, their long-term impacts are less well understood. In this work, we study the user experience on Spotify, a popular music streaming service, through the lens of diversity—the coherence of the set of songs a user listens to. We use a high-fidelity embedding of millions of songs based on listening behavior on Spotify to quantify how musically diverse every user is, and find that high consumption diversity is strongly associated with important long-term user metrics, such as conversion and retention. However, we also find that algorithmically-driven listening through recommendations is associated with reduced consumption diversity. Furthermore, we observe that when users become more diverse in their listening over time, they do so by shifting away from algorithmic consumption and increasing their organic consumption. Finally, we deploy a randomized experiment and show that algorithmic recommendations are more effective for users with lower diversity. Our work illuminates a central tension in online platforms: how do we recommend content that users are likely to enjoy in the short term while simultaneously ensuring they can remain diverse in their consumption in the long term?
更多查看译文
AI 理解论文
溯源树
样例
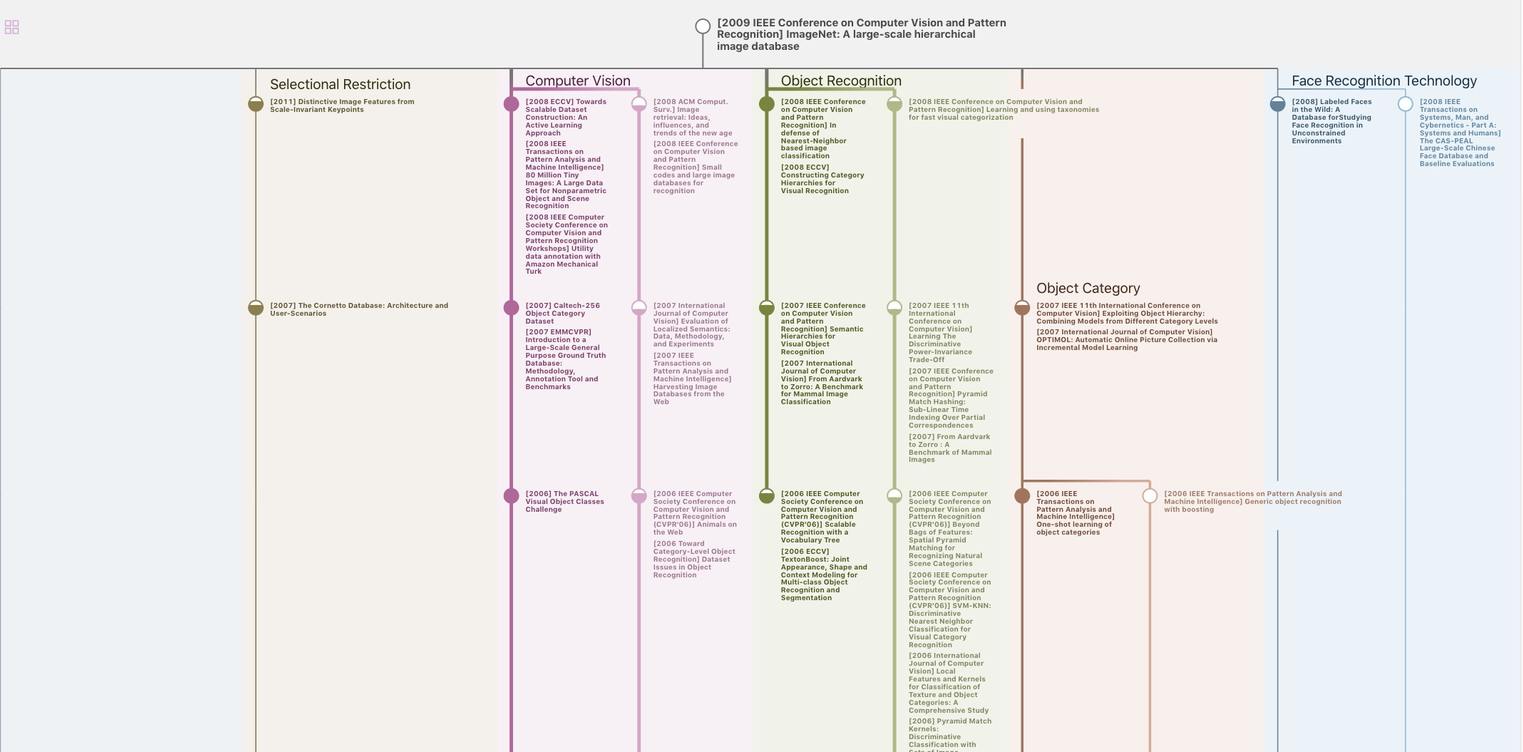
生成溯源树,研究论文发展脉络
Chat Paper
正在生成论文摘要