Graph Enhanced Representation Learning for News Recommendation
WWW '20: The Web Conference 2020 Taipei Taiwan April, 2020(2020)
摘要
With the explosion of online news, personalized news recommendation becomes increasingly important for online news platforms to help their users find interesting information. Existing news recommendation methods achieve personalization by building accurate news representations from news content and user representations from their direct interactions with news (e.g., click), while ignoring the high-order relatedness between users and news. Here we propose a news recommendation method which can enhance the representation learning of users and news by modeling their relatedness in a graph setting. In our method, users and news are both viewed as nodes in a bipartite graph constructed from historical user click behaviors. For news representations, a transformer architecture is first exploited to build news semantic representations. Then we combine it with the information from neighbor news in the graph via a graph attention network. For user representations, we not only represent users from their historically clicked news, but also attentively incorporate the representations of their neighbor users in the graph. Improved performances on a large-scale real-world dataset validate the effectiveness of our proposed method.
更多查看译文
关键词
News Recommendation, Transformer, Graph Attention Network
AI 理解论文
溯源树
样例
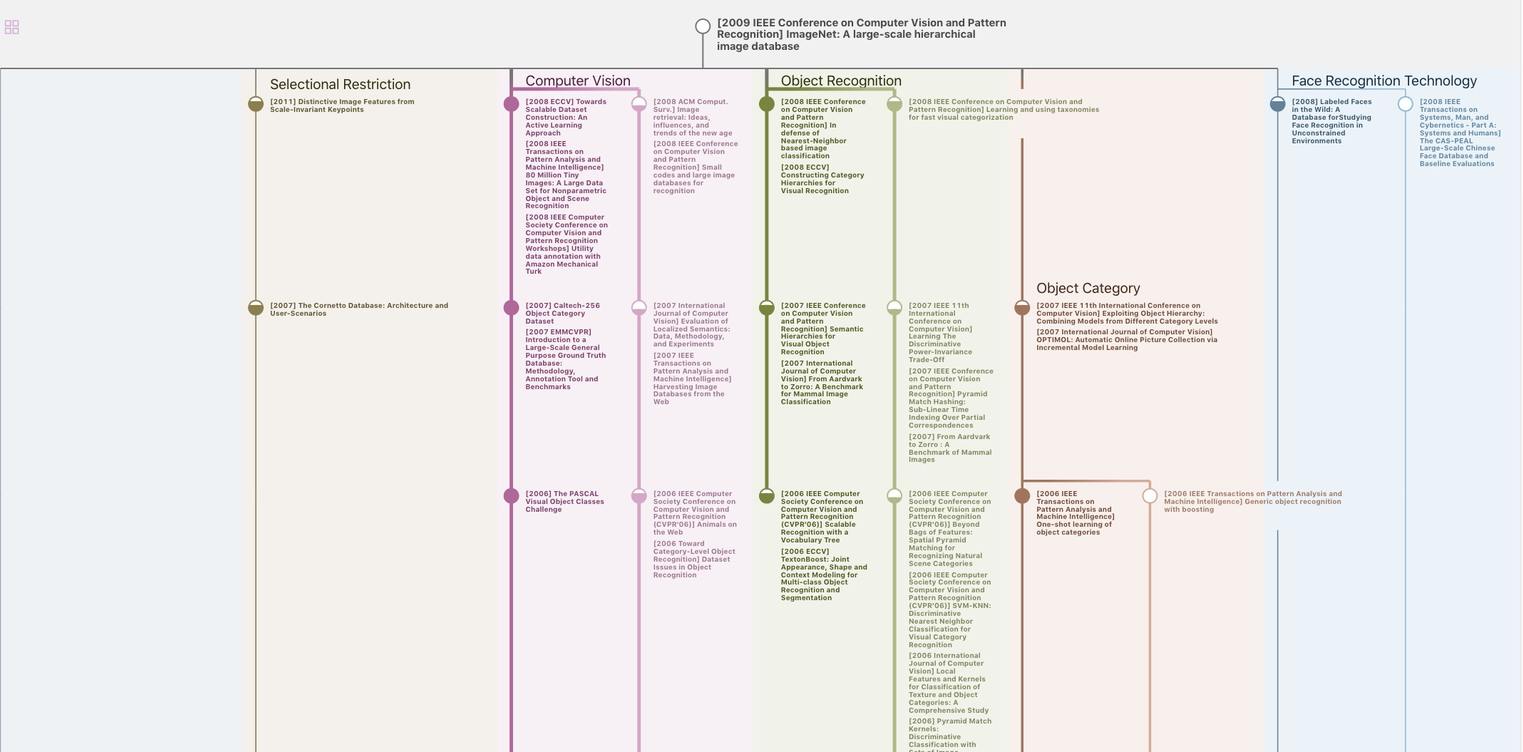
生成溯源树,研究论文发展脉络
Chat Paper
正在生成论文摘要