Near-Perfect Recovery in the One-Dimensional Latent Space Model
WWW '20: The Web Conference 2020 Taipei Taiwan April, 2020(2020)
摘要
Suppose a graph G is stochastically created by uniformly sampling vertices along a line segment and connecting each pair of vertices with a probability that is a known decreasing function of their distance. We ask if it is possible to reconstruct the actual positions of the vertices in G by only observing the generated unlabeled graph. We study this question for two natural edge probability functions — one where the probability of an edge decays exponentially with the distance and another where this probability decays only linearly. We initiate our study with the weaker goal of recovering only the order in which vertices appear on the line segment. For a segment of length n and a precision parameter δ, we show that for both exponential and linear decay edge probability functions, there is an efficient algorithm that correctly recovers (up to reflection symmetry) the order of all vertices that are at least δ apart, using only samples (vertices). Building on this result, we then show that vertices (samples) are sufficient to additionally recover the location of each vertex on the line to within a precision of δ. We complement this result with an lower bound on samples needed for reconstructing positions (even by a computationally unbounded algorithm), showing that the task of recovering positions is information-theoretically harder than recovering the order. We give experimental results showing that our algorithm recovers the positions of almost all points with high accuracy.
更多查看译文
关键词
latent space model, reconstruction, sample complexity
AI 理解论文
溯源树
样例
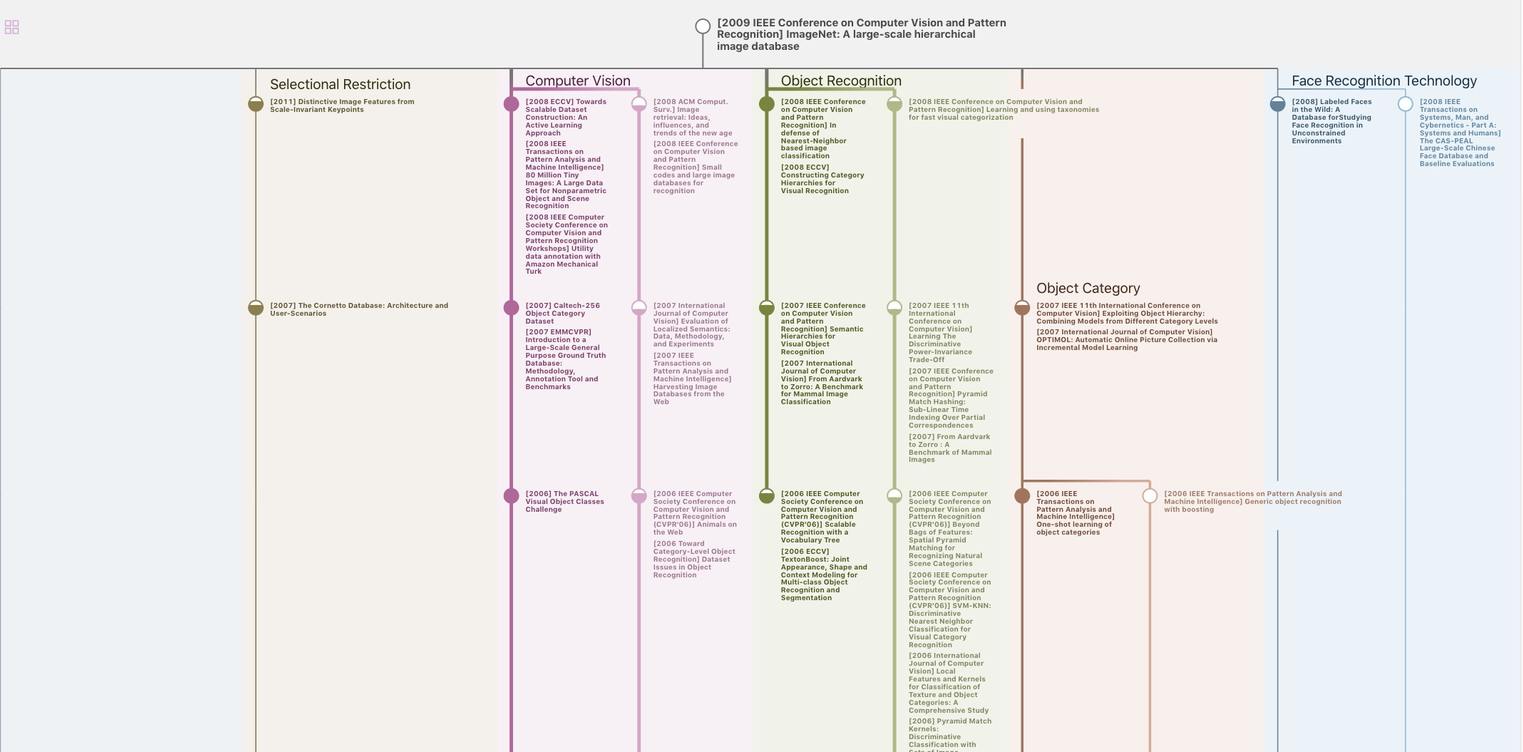
生成溯源树,研究论文发展脉络
Chat Paper
正在生成论文摘要