Attentive Sequential Models of Latent Intent for Next Item Recommendation
WWW '20: The Web Conference 2020 Taipei Taiwan April, 2020(2020)
摘要
Users exhibit different intents across e-commerce services (e.g. discovering items, purchasing gifts, etc.) which drives them to interact with a wide variety of items in multiple ways (e.g. click, add-to-cart, add-to-favorites, purchase). To give better recommendations, it is important to capture user intent, in addition to considering their historic interactions. However these intents are by definition latent, as we observe only a user’s interactions, and not their underlying intent. To discover such latent intents, and use them effectively for recommendation, in this paper we propose an Attentive Sequential model of Latent Intent (ASLI in short). Our model first learns item similarities from users’ interaction histories via a self-attention layer, then uses a Temporal Convolutional Network layer to obtain a latent representation of the user’s intent from her actions on a particular category. We use this representation to guide an attentive model to predict the next item. Results from our experiments show that our model can capture the dynamics of user behavior and preferences, leading to state-of-the-art performance across datasets from two major e-commerce platforms, namely Etsy and Alibaba.
更多查看译文
关键词
Next Item Recommendation, User Modeling, Latent Intent
AI 理解论文
溯源树
样例
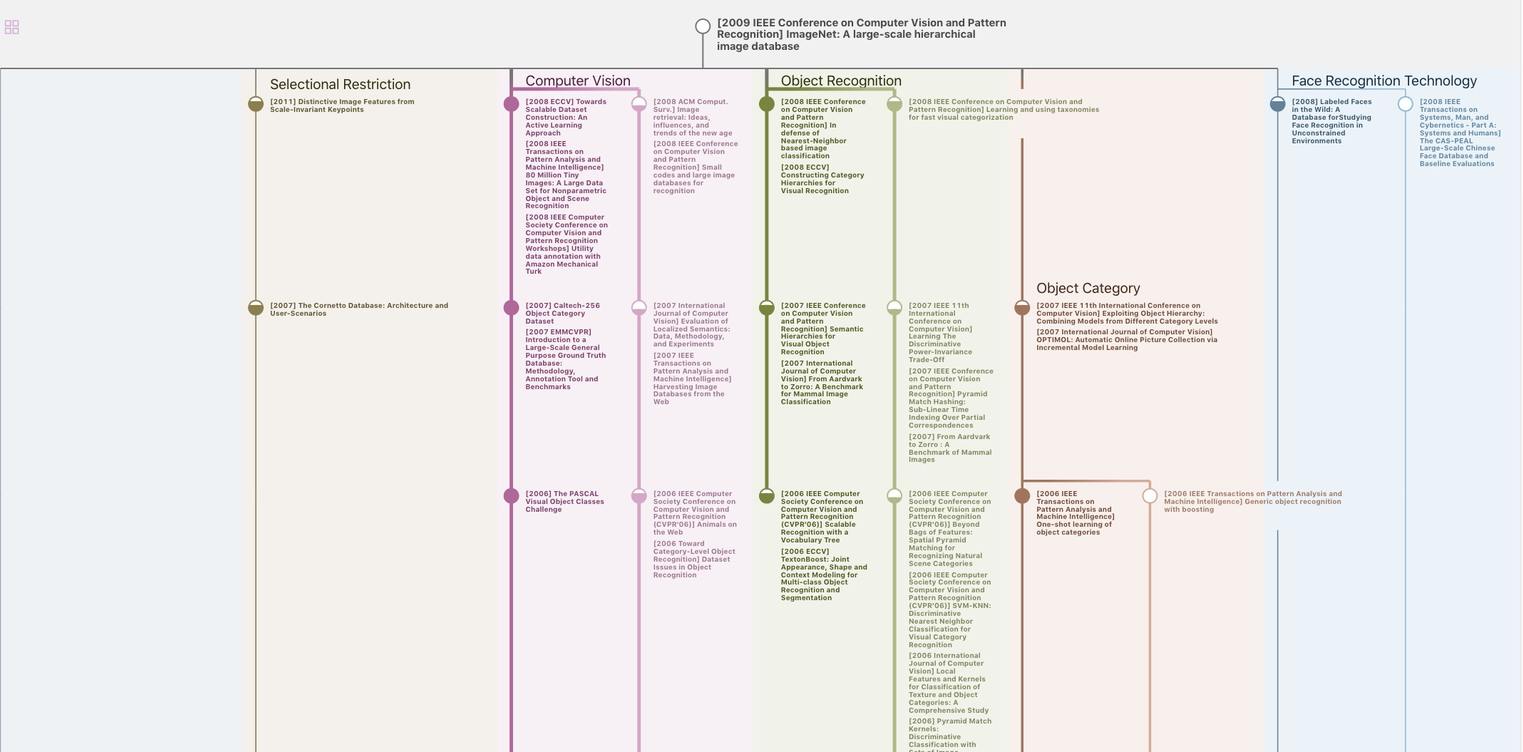
生成溯源树,研究论文发展脉络
Chat Paper
正在生成论文摘要