Mammography Image Quality Assurance Using Deep Learning
IEEE Transactions on Biomedical Engineering(2020)
摘要
Objective: According to the European Reference Organization for Quality Assured Breast Cancer Screening and Diagnostic Services (EUREF) image quality in mammography is assessed by recording and analyzing a set of images of the CDMAM phantom. The EUREF procedure applies an automated analysis combining image registration, signal detection and nonlinear fitting. We present a proof of concept for an end-to-end deep learning framework that assesses image quality on the basis of single images as an alternative. Methods: Virtual mammography is used to generate a database with known ground truth for training a regression convolutional neural net (CNN). Training is carried out by continuously extending the training data and applying transfer learning. Results: The trained net is shown to correctly predict the image quality of simulated and real images. Specifically, image quality predictions on the basis of single images are of similar quality as those obtained by applying the EUREF procedure with 16 images. Our results suggest that the trained CNN generalizes well. Conclusion: Mammography image quality assessment can benefit from the proposed deep learning approach. Significance: Deep learning avoids cumbersome pre-processing and allows mammography image quality to be estimated reliably using single images.
更多查看译文
关键词
Breast Neoplasms,Deep Learning,Female,Humans,Image Processing, Computer-Assisted,Mammography,Neural Networks, Computer,Phantoms, Imaging
AI 理解论文
溯源树
样例
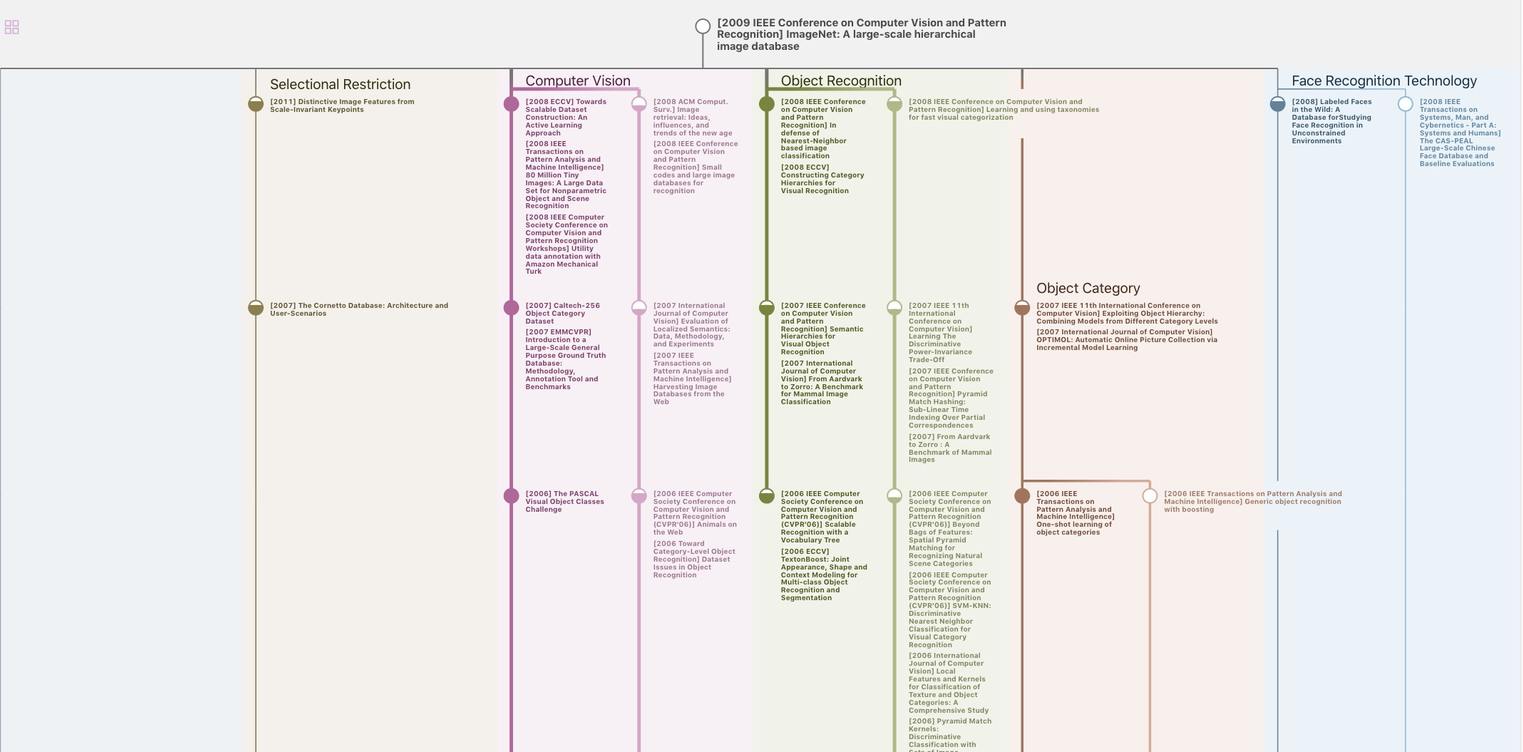
生成溯源树,研究论文发展脉络
Chat Paper
正在生成论文摘要