Accelerating Physics-Informed Neural Network Training with Prior Dictionaries
arxiv(2020)
摘要
Physics-Informed Neural Networks (PINNs) can be regarded as general-purpose PDE solvers, but it might be slow to train PINNs on particular problems, and there is no theoretical guarantee of corresponding error bounds. In this manuscript, we propose a variant called Prior Dictionary based Physics-Informed Neural Networks (PD-PINNs). Equipped with task-dependent dictionaries, PD-PINNs enjoy enhanced representation power on the tasks, which helps to capture features provided by dictionaries so that the proposed neural networks can achieve faster convergence in the process of training. In various numerical simulations, compared with existing PINN methods, combining prior dictionaries can significantly enhance convergence speed. In terms of theory, we obtain the error bounds applicable to PINNs and PD-PINNs for solving elliptic partial differential equations of second order. It is proved that under certain mild conditions, the prediction error made by neural networks can be bounded by expected loss of PDEs and boundary conditions.
更多查看译文
关键词
dictionaries,training,physics-informed
AI 理解论文
溯源树
样例
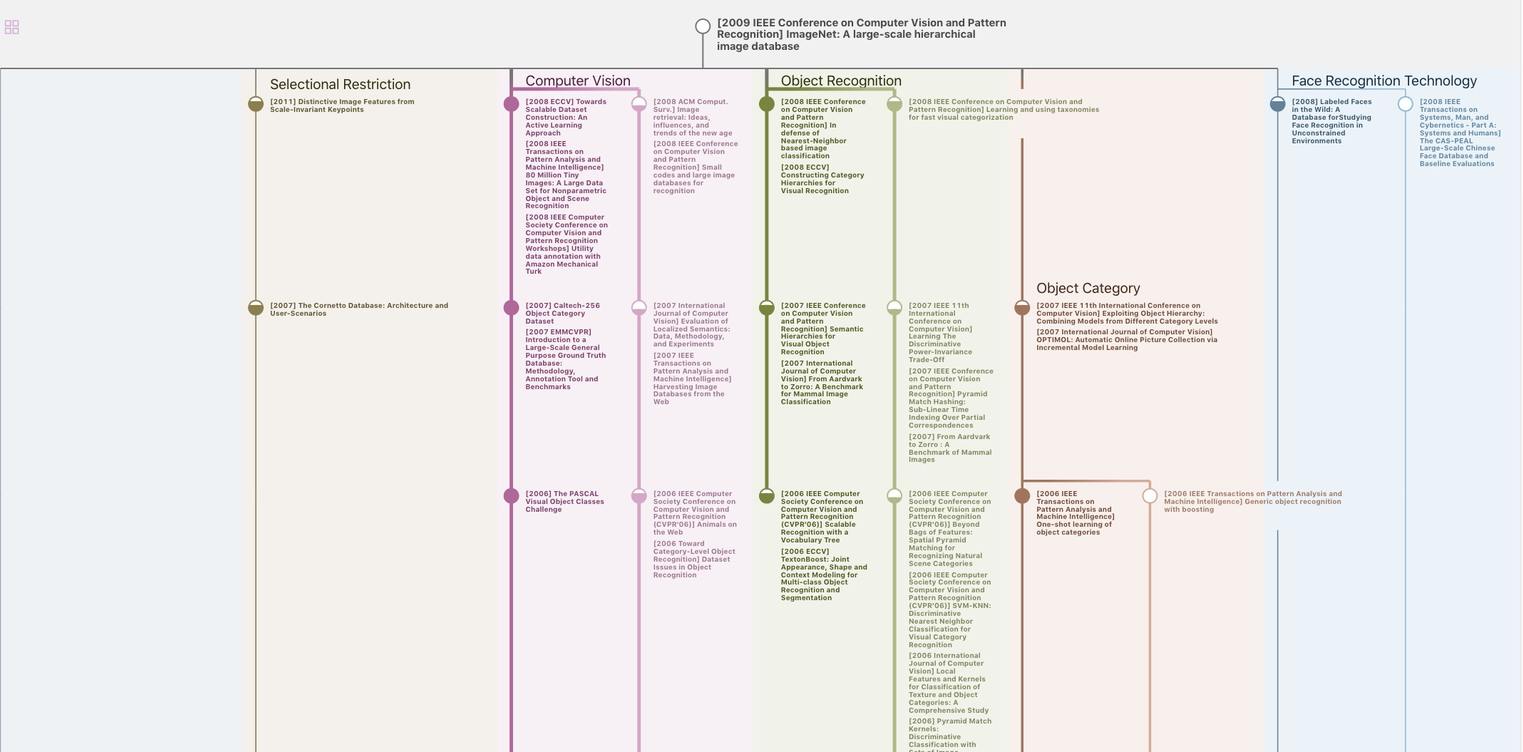
生成溯源树,研究论文发展脉络
Chat Paper
正在生成论文摘要