Approximate Inverse Reinforcement Learning from Vision-based Imitation Learning
2021 IEEE INTERNATIONAL CONFERENCE ON ROBOTICS AND AUTOMATION (ICRA 2021)(2021)
摘要
In this work, we present a method for obtaining an implicit objective function for vision-based navigation. The proposed methodology relies on Imitation Learning, Model Predictive Control (MPC), and an interpretation technique used in Deep Neural Networks. We use Imitation Learning as a means to do Inverse Reinforcement Learning in order to create an approximate cost function generator for a visual navigation challenge. The resulting cost function, the costmap, is used in conjunction with MPC for real-time control and outperforms other state-of-the-art costmap generators in novel environments. The proposed process allows for simple training and robustness to out-of-sample data. We apply our method to the task of vision-based autonomous driving in multiple real and simulated environments and show its generalizability. Supplementary video: https://youtu.be/WyJfT5lc0aQ
更多查看译文
关键词
real-time control,costmap generators,vision-based autonomous driving,approximate inverse reinforcement learning,vision-based imitation learning,implicit objective function,vision-based navigation,model predictive control,MPC,deep neural networks,approximate cost function generator,visual navigation challenge,cost function
AI 理解论文
溯源树
样例
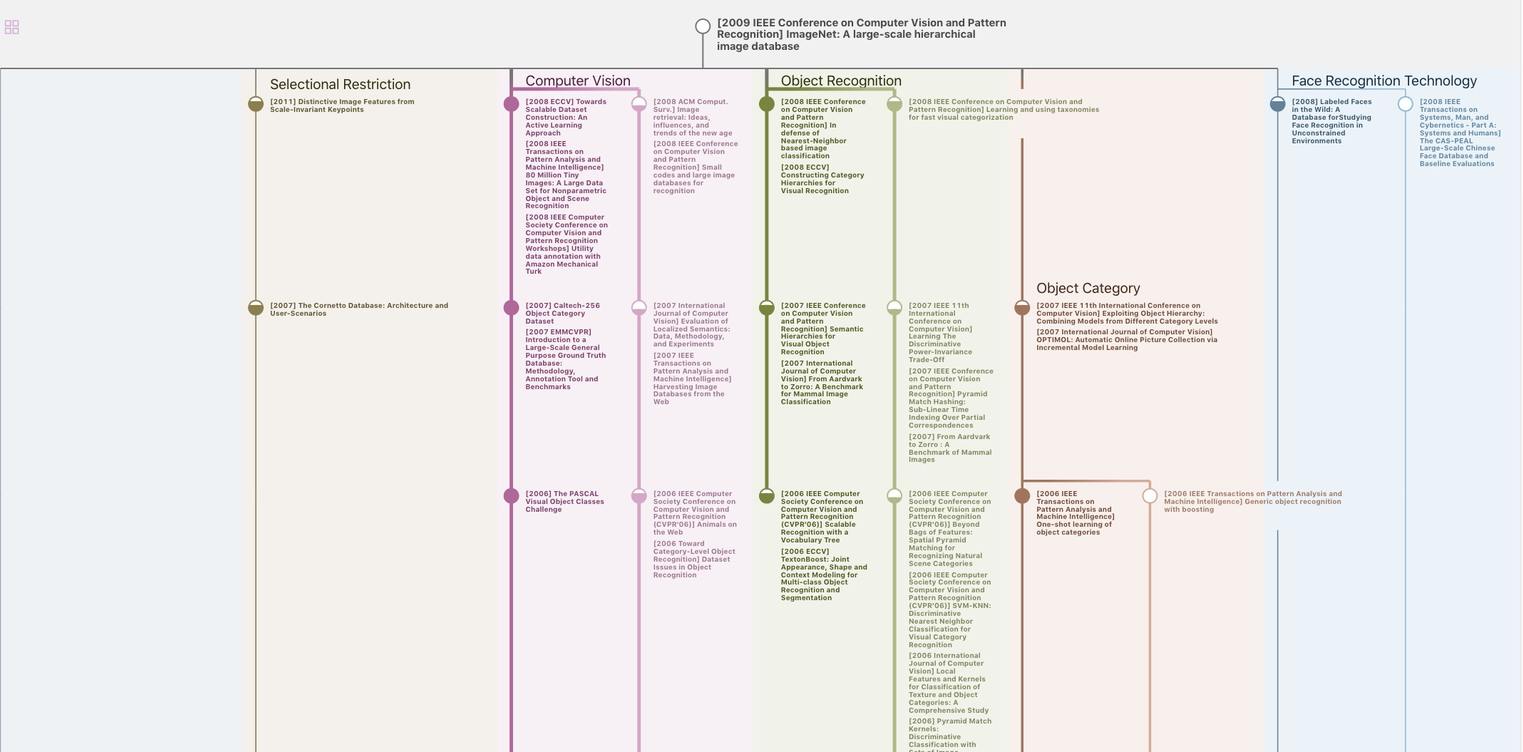
生成溯源树,研究论文发展脉络
Chat Paper
正在生成论文摘要