15.3 A 351tops/W And 372.4gops Compute-In-Memory Sram Macro In 7nm Finfet Cmos For Machine-Learning Applications
2020 IEEE INTERNATIONAL SOLID- STATE CIRCUITS CONFERENCE (ISSCC)(2020)
摘要
Compute-in-memory (CIM) parallelizes multiply-and-average (MAV) computations and reduces off-chip weight access to reduce energy consumption and latency, specifically for Al edge devices. Prior CIM approaches demonstrated tradeoffs for area, noise margin, process variation and weight precision. 6T SRAM [1]–[3] provides the smallest cell area for CIM, but cell stability limits the number of activated cells, resulting in low parallelization. 10T and twin-8T [4]–[5] isolate the read/write paths for noise margin improvement, however both require special design of the bit cell using logic layout rules, resulting in over a 2x area overhead compared to foundry yield-optimized 6T SRAMs. Furthermore, single-bit precision of weights, in prior work [1]–[4], cannot meet the requirement for high-precision operations and scalability for large neural networks.
更多查看译文
关键词
compute-in-memory,machine-learning
AI 理解论文
溯源树
样例
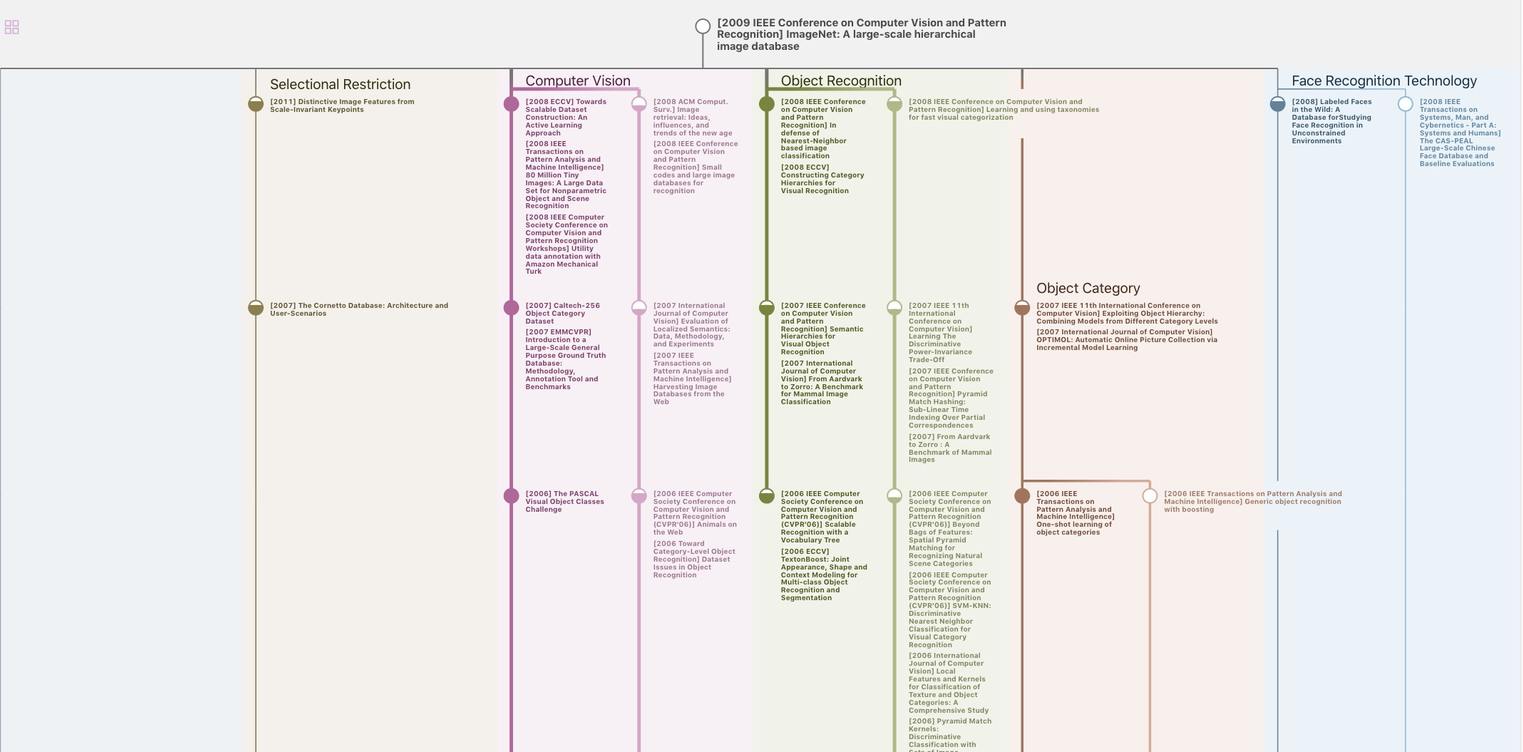
生成溯源树,研究论文发展脉络
Chat Paper
正在生成论文摘要