Automatic Segmentation Of Necrosis Zones After Radiofrequency Ablation Of Spinal Metastases
ICPRAM: PROCEEDINGS OF THE 9TH INTERNATIONAL CONFERENCE ON PATTERN RECOGNITION APPLICATIONS AND METHODS(2020)
摘要
In this work, we propose an automatic deep learning-based approach to segment necrotizing tissue (necrosis zones) after radiofrequency ablations (RFA) of spinal metastases in follow-up Magnetic Resonance (MR) images. While the manual segmentation of those necrosis zones is challenging and time consuming, it is a crucial step to assess, whether a preceding therapy using RFA was successful and to what extent, i.e., to quantitatively evaluate how much of the metastasis was necrotized throughout the therapy. Therefore, we trained a U-Net like deep neural network on 26 clinical cases (and various augmentations of those), where each case had an associated contrast enhanced T-1-weighted as well as a T-2-weighted MR sequence. We evaluated the proposed approach on both sequences separately as well as in a combined setting and report Dice coefficients, sensitivity-, and specificity rates for the automatic segmentations. A Dice coefficient of up to 77.2 % indicates promising segmentation quality, if compared to related work and similar segmentation tasks. To the best of our knowledge, this is the first work to tackle the problem of automatic segmentation of necrosis zones in MR images and therefore lacks comparability with related works. However, our best results are somewhat superior to semi-automatic approaches of liver metastases segmentation, which might be considered a problem of similar complexity.
更多查看译文
关键词
Medical Image Segmentation, Deep Learning, Radiofrequency Ablation, MRI
AI 理解论文
溯源树
样例
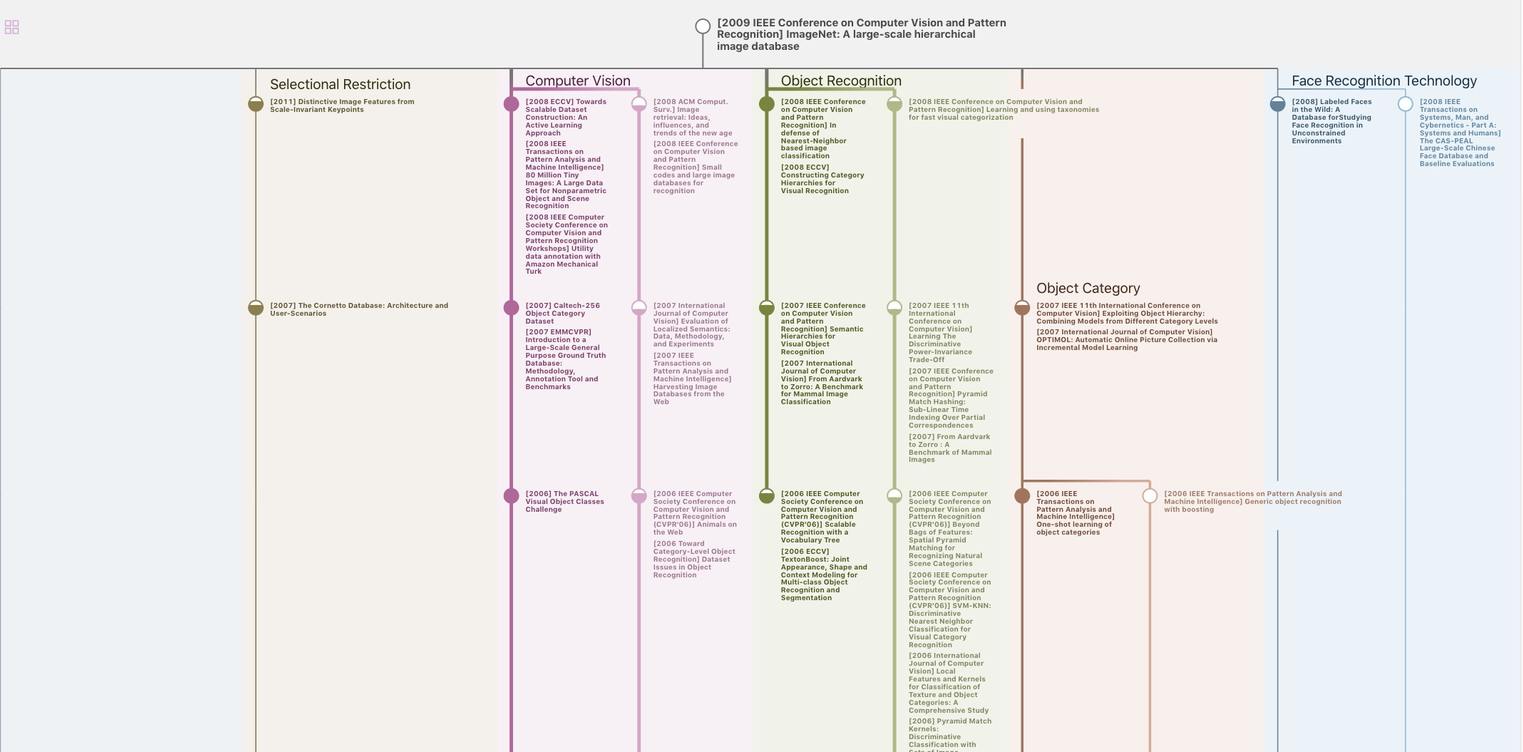
生成溯源树,研究论文发展脉络
Chat Paper
正在生成论文摘要